|
|
|
ABSTRACT |
Progression of a team’s performance is a key issue in competitive sport, but there appears to have been no published research on team progression for periods longer than a season. In this study we report the game-score progression of three teams of a youth talent-development academy over five seasons using a novel analytic approach based on generalised mixed modelling. The teams consisted of players born in 1991, 1992 and 1993; they played totals of 115, 107 and 122 games in Asia and Europe between 2005 and 2010 against teams differing in age by up to 3 years. Game scores predicted by the mixed model were assumed to have an over-dispersed Poisson distribution. The fixed effects in the model estimated an annual linear pro-gression for Aspire and for the other teams (grouped as a single opponent) with adjustment for home-ground advantage and for a linear effect of age difference between competing teams. A random effect allowed for different mean scores for Aspire and opposition teams. All effects were estimated as factors via log-transformation and presented as percent differences in scores. Inferences were based on the span of 90% confidence intervals in relation to thresholds for small factor effects of x/÷1.10 (+10%/-9%). Most effects were clear only when data for the three teams were combined. Older teams showed a small 27% increase in goals scored per year of age difference (90% confidence interval 13 to 42%). Aspire experienced a small home-ground advantage of 16% (-5 to 41%), whereas opposition teams experienced 31% (7 to 60%) on their own ground. After adjustment for these effects, the Aspire teams scored on average 1.5 goals per match, with little change in the five years of their existence, whereas their opponents’ scores fell from 1.4 in their first year to 1.0 in their last. The difference in progression was trivial over one year (7%, -4 to 20%), small over two years (15%, -8 to 44%), but unclear over >2 years. In conclusion, the generalized mixed model has marginal utility for estimating progression of soccer scores, owing to the uncertainty arising from low game scores. The estimates are likely to be more precise and useful in sports with higher game scores. |
Key words:
Association football, generalised mixed model, key performance indicators, performance trends
|
Key
Points
- A generalized linear mixed model is the approach for tracking game scores, key performance indicators or other measures of performance based on counts in sports where changes within and/or between games/seasons have to be considered.
- Game scores in soccer could be useful to track performance progression of teams, but hundreds of games are needed.
- Fewer games will be needed for tracking performance represented by counts with high scores, such as game scores in rugby or key performance indicators based on frequent events or player actions in any team sport.
|
Has your team improved?" is an important question for coaches and support staff that needs to be addressed with appropriate measures of performance in competitions. Match analysis can provide measures of various aspects of technical/tactical and physical performance, but the game score itself is the criterion for assessing overall progression. Surprisingly, there has been no published research using game scores to track progression of team performances over periods longer than a year. In previous studies of association football (soccer), game scores have been analysed mainly to predict individual game outcomes and probability of a team winning a national league (Karlis and Ntzoufras, 2003; 2009; Lee, 1997; Maher, 1982; Rue and Salvesen, 2000) or a knock-out tournament (Dyte and Clarke, 2000; Koning et a., 2003). In these analyses game scores were modelled assuming a distribution appropriate for count data, the Poisson or over-dispersed Poisson distribution. Important predictors included in previous models were parameters describing relative quality of teams. In national leagues, where all teams play each other the same number of times, the parameters described each team’s attacking and defensive ability (Karlis and Ntzoufras, 2003; Lee, 1997; Maher, 1982; Rue and Salvesen, 2000). For analyses of tournaments at World Cups, differences in teams’ abilities were addressed using the FIFA ranking system (Dyte and Clarke, 2000). All previous models included a game location effect addressing whether a team was playing at home or away. The models used in previous studies cannot be applied directly to develop the performance progression of soccer teams of youth talent-development academies, for the following reasons. First, progression implies tracking the performance in different years, therefore a time variable is required in the analyses. Secondly, quality of competitors cannot be addressed using attacking/defensive parameters or FIFA world rankings, which are derived from series of games between most or all possible pairings of teams. Finally, the models need to include an effect for age difference between playing teams, which at an academy level is likely to impact performance. In the present study we have applied a generalised mixed linear model to game scores with effects accounting for an annual trend of performance, quality of teams, age of competitors and home-ground advantage. We investigated the progression of three youth soccer teams from the Aspire Academy for Sport Excellence (Doha, Qatar) for the years 2005 to 2010, comparing their performance against that of their opponents.
Performance dataThe data were official game scores of three Aspire teams and their respective opponents over the period 2005 to 2010. Informed consent was not required for approval by our institutional ethics committee, because game scores are in the public domain. The three Aspire cohorts consisted of players born in 1991, 1992 and 1993. Over the five years of their development program, these cohorts played 115, 107 and 122 games scoring 163, 176 and 188 goals against 61, 56 and 60 different opponents, who scored totals of 173, 141 and 174 goals, respectively. Matches were contested in Asia and Europe, either as friendly games (when one team is played at home and other away) or at small tournaments (both teams playing away). The age difference between Aspire team and their opponents was up to three years.
Statistical analysisThe analysis presented an opportunity to trial the generalized linear mixed modelling procedure, Proc Glimmix, recently available in the Statistical Analysis System (Version 9.2, SAS Institute, Cary, NC). This pro-cedure can model complex repeated-measures structures that cannot be accommodated than the established form of the generalized linear model known as generalized estimating equations; although these could have used with our data. The number of goals scored by each team was modelled as an over-dispersed Poisson distribution to allow for the variance of the counts to be different from the mean count. The fixed effects (and their estimates) were as follows: Team (with two levels, estimating a different mean score for Aspire and for the other teams grouped as Opposition), Team interacting with the play-ing season (allowing for a linear annual trend in perform-ance for Aspire and Opposition), HomeAway interacted with Team (accounting for an advantage when Aspire or Opposition were playing at home), and a linear AgeDifference interacted with Team (reflecting the advantage per year of difference between the mean age of the teams, with a separate estimate for Aspire and Opposition). An annual linear trend in performance rather than quadratic or higher order trend was deemed the most appropriate, based on assessment of the annual mean scores. In the model the estimated mean goals were adjusted to a zero age difference and equal numbers of games played at home and away. The random effect Team interacting with identity of the team in opposition was included to account for opponents’ different abilities and Aspire’s ability against those opponents. The analyses were performed individually for each Aspire cohort and for the three cohorts combined. In the combined analysis opposition teams with the same name in different years were treated as independent teams (i.e., not counted as repeated measurements). Modelling was also investigated for team-performance progression within a season. Dates of each game were not available, but the temporal order was known and used as the time variable. Team performance within-season was predicted with similar Team, HomeAway and AgeDifference effects and an interaction between Team and game order to estimate different within-season rates of progression for Aspire and Opposi-tion. The effects were derived as ratios from the model but expressed as percentage difference. Magnitudes of effects were categorised in relation to the default thresholds for counts, with small, moderate and large factor effects of x/÷1.10, x/÷1.40 and x/÷2.0 (+10%/-9%, +40%/-29% and 100%/-50%) (Hopkins, 2010). An inference about the true (large-sample) value of the effect was based on uncertainty in its magnitude: if the 90% confidence interval overlapped small positive and negative values, the magnitude was deemed unclear; otherwise, the magnitude was deemed to be the observed magnitude (Batterham and Hopkins, 2006).
Results are presented only for the analysis when games data from the three cohorts (Aspire teams born 1991, 1992 and 1993) were combined. The individual analysis for each cohort produced mainly unclear effects.
Age effectAge difference had similar effects for Aspire and Opposition, so they were combined into a single effect. An one-year difference between playing teams offered a small advantage of 27% more goals for the older one (confidence interval 13 to 42%). The age effect was mod-elled as a linear variable with the log of mean number of goals; consequently two- and three-year gaps resulted in moderate and large effects of 61% and 105% more goals scored by the older team.
Home-ground effectThe Aspire team experienced an advantage of 16% higher scores (-5 to 41%), whereas Opposition scores where higher by 31% (7 to 60%) when playing on their own ground, both effects being small. The difference between the two effects was unclear (13%, -15 to 49%).
Performance progressionFigure 1 shows the mean number of goals scored per season by the Aspire and Opposition teams over the five years (Season 04/05 through to 09/10). After adjusting for age-difference and home-ground effects, Aspire scored on average 1.5 goals per match, with no change over the five years. On the other hand, the Opposition’s mean performance fell from 1.4 goals per match in their first season to 1.0 goals in the last. At the end of the first year (04/05) the difference between the two adjusted means was trivial (5%, -15% to 30%), whereas by the end of 09/10 Aspire scored moderately more goals than the Opposition (40%, 0 to 96%). The comparison of the performance progressions showed a trivial difference between the two teams over one year (7%, -4 to 20%), small over two years (15%, -8 to 44%) and unclear for three years (24%, -11 to 74%) and longer periods. Within-season team progression was explored using data from the 15-31games for each season of each the three cohorts. When the model specifying home-ground and age-difference effects as predictors of mean number of goals was applied, the estimated ratios of progression of Aspire vs Opposition had on average an uncertainty of x/÷4.0. Thus, for observed differences to be clear, they would have to be at least very large. When a more simplistic model ignoring home-ground and age effects was applied, the uncertainty decreased to x/÷2.7, which still represent large uncertainty.
We have investigated the five-year performance progression of three academy soccer-team cohorts using a novel application of generalised linear mixed modelling. The analysis revealed substantial effects on performance for an age difference between teams, for game location, and for differences in progression of the Aspire and Opposition teams. There were no clear outcomes for within-season performance progressions. An age difference of one year between opposing teams resulted in a small advantage for the older team. This advantage is obviously due to differences in physical maturity, which is highly correlated with physical performance during puberty (Mujika et al., 2009). Even an age difference of less than a year produces the well-known relative age-effect in performance, which has been demonstrated in soccer (Helsen et al., 2005) amongst many other sports. The same authors suggest that advantage experienced by older players may also reflect psychological maturity and longer exposure to practise and matches, resulting on the development of technical and game intelligence skills. Our estimate of the age effect is likely to be biased low, because games between teams differing in age are more likely to have been set up when the perceived abilities of opposing teams were similar. The estimated small advantage for the team playing at home is consistent with previous studies, in which the home-ground factor represented approximately 40% higher number of goals for the hosting team (Koning et al., 2003; Lee, 1997). The estimated home-ground effect in our study was a little lower, but differences between the two values may be due to the different nature of players (professional vs. youth). The difference between home advantage experienced by the Aspire and Opposition teams was unclear; however, there was an indication of a greater home-ground effect for the opposition. If the true difference between the home advantages is substantial, possible reasons include different climate conditions and different fan support that players experienced in the Qatar venue vs the opposition venues. Although the analysis for progression for each cohort involved ~100 games, the effects on progression were not clear until all three cohorts were included in the analysis-a sample size of ~300 games. The average performance of Aspire cohorts was fairly constant over the five-year period, while the opposition gradually scored less goals. The most obvious explanation for this outcome is an improvement of Aspire performance through development of their defensive ability. A reduction in the opposition’s attacking ability seems a less likely explanation, but this issue could be resolved only by an analysis of scores from games where opposition teams play each other. The assessment of the magnitude of effects in this study depends on the chosen thresholds. The threshold for small was the default 10% change in the score. However to be consistent with previous research on solo athletes, the threshold should be the smallest change that would increase by 10% the chance of winning against an equally match opponent. Further research is needed to establish this change. The large uncertainty on the estimates for the within-season progression prevented any investigation of teams’ abilities. Indeed, the only useful finding here is that there are insufficient games in a season to quantify anything less than large or very large effects. The removal of predictors from a model normally increases the uncertainty in the estimates of effects, but in the present case collinearity among the predictors and limited sample size resulted in better precision with the simpler model. The resulting uncertainty was still unacceptable for any practical application. The unclear effects on progression arise from the fundamentally noisy nature of scores with low counts. Evidently, chance is such a major contributor to soccer outcomes that even an entire season of games is insufficient to explore performance progression. Estimates with better precision would be produced using performance indicators with higher numbers of counts as measures of team performance or effectiveness. Scoring opportunities or score box possessions as defined in Tenga et al., 2010 are two examples of such measures for soccer. Modelled progressions could also be extended to other performance indicators describing the different technical aspects of performance, such as defence, passing, crossing and goal attempts (Oberstone, 2009). Progressions of such performance indicators would then provide evidence and help to explain the progression of game scores. A more detailed match analysis using such performance indicators was beyond of the scope of this study.
We have presented a novel statistical approach for using objective performance measures to investigate progression of a team. The methodology uses the generalized linear mixed model to account for the different teams’ abilities via the repeated-measures structure of the data. This statistical approach will be particularly useful for analyses of other complex performance data. Although limited in its application for soccer scores, the model we have devised should be useful for modelling progression of competitive performance in sports where scores are higher.
|
AUTHOR BIOGRAPHY |
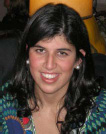 |
Rita M. Malcata |
Employment: PhD scholar/Performance and Technique Analysis, High Performance Sport New Zealand, Auckland, NZ. |
Degree: BSc, MSc in Biomedical Engineering |
Research interests: Research design and analysis; athletic performance. |
E-mail: rita.malcata@aut.ac.nz |
|
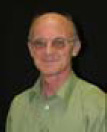 |
Will G Hopkins |
Employment: Professor of Exercise Science, Auckland University of Technology, Auckland, NZ. |
Degree: BSc, BA, MSc, PhD |
Research interests: Research design and analysis; athletic performance. |
E-mail: will.hopkins@aut.ac.nz |
|
 |
Scott Richardson |
Employment: Senior Sports Performance Officer, Aspire Academy for Sports Excellence, Doha Qatar. |
Degree: BSc, BA, MSc, PhD |
Research interests: Athletic performance. |
E-mail: scott.richardson@aspire.qa |
|
|
|
REFERENCES |
 Batterham A.M., Hopkins W.G. (2006) Making meaningful inferences about magnitudes. International Journal of Sports Physiology and Performance 1, 50-57.
|
 Dyte D., Clarke S.R. (2000) A rating based Poisson model for World Cup soccer simulation. Journal of the Operational Research Society 51, 993-998.
|
 Helsen W.F., Van Winckel J., Williams A.M. (2005) The relative age effect in youth soccer across Europe. Journal of Sports Sciences 23, 629-636.
|
 Hopkins W.G. (2010) Linear models and effect magnitudes for research, clinical and practical approaches. Sportscience 14, 49-57.
|
 Karlis D., Ntzoufras I. (2003) Analysis of sports data by using bivariate poisson models. Journal of Royal Statistical Society-Serie D (The Statistician) 52, 381-393.
|
 Karlis D., Ntzoufras I. (2009) Bayesian modelling of football outcomes: using the Skellam's distribution for the goal difference. IMA Journal of Management Mathematics 20, 133-145.
|
 Koning R.H., Koolhaas M., Renes G., Ridder G. (2003) A simulation model for football championships. European Journal of Operational Research 148, 268-276.
|
 Lee A.J. (1997) Modeling scores in the premier league: Manchester United really the best?. Chance 10, 15-19.
|
 Maher M.J. (1982) Modelling association football scores. Statistica Neerlandica 36, 109-118.
|
 Mujika I., Vaeyens R., Matthys S.P.J., Santisteban J., Goiriena J., Philippaerts R. (2009) The relative age effect in a professional football club setting. Journal of Sports Sciences 27, 1153-1158.
|
 Oberstone J. (2009) Differentiating the top english Premier League football clubs from the rest of the pack: identifying the keys to sucess. Journal of Quantitative Analysis in Sport 5, -.
|
 Rue H., Salvesen O. (2000) Prediction and retrospective analysis of soccer matches in a league. Journal of Royal Statistical Society-Serie D (The Statistician) 49, 399-418.
|
 Tenga A., Ronglan L., Bahr R. (2010) Measuring the effectiveness of offensive match-play in professional soccer. European Journal of Sport Science 10, 269-277.
|
|
|
|
|
|
|