|
|
|
ABSTRACT |
This study focused on identifying the neural markers underlying optimal and suboptimal performance experiences of an elite air-pistol shooter, based on the tenets of the multi-action plan (MAP) model. According to the MAP model’s assumptions, skilled athletes’ cortical patterns are expected to differ among optimal/automatic (Type 1), optimal/controlled (Type 2), suboptimal/controlled (Type 3), and suboptimal/automatic (Type 4) performance experiences. We collected performance (target pistol shots), cognitive-affective (perceived control, accuracy, and hedonic tone), and cortical activity data (32-channel EEG) of an elite shooter. Idiosyncratic descriptive analyses revealed differences in perceived accuracy in regard to optimal and suboptimal performance states. Event-Related Desynchronization/Synchronization analysis supported the notion that optimal-automatic performance experiences (Type 1) were characterized by a global synchronization of cortical arousal associated with the shooting task, whereas suboptimal controlled states (Type 3) were underpinned by high cortical activity levels in the attentional brain network. Results are addressed in light of the neural efficiency hypothesis and reinvestment theory. Perceptual training recommendations aimed at restoring optimal performance levels are discussed. |
Key words:
MAP model, EEG, ERD/ERS, shooting, elite performance
|
Key
Points
- We investigated the neural markers underlying optimal and suboptimal performance experiences of an elite air-pistol shooter.
- Optimal/automatic performance is characterized by a global synchronization of cortical activity associated with the shooting task.
- Suboptimal controlled performance is characterized by high cortical arousal levels in the attentional brain networks.
- Focused Event Related Desynchronization activity during Type 1 performance in frontal midline theta was found, with a clear distribution of Event Related Synchronization in the frontal and central areas just prior to shot release.
- Event Related Desynchronization patterns in low Alpha band for Type 3 performance suggest that higher levels of general cortical arousal are associated with suboptimal-controlled performance states.
|
The analysis of psychobiosocial mechanisms underlying optimal performance experiences has received a great deal of attention in the domain of sport and exercise psychology (Hanin, 2007; Robazza, 2006). Researchers have adopted multimodal approaches to target different structural components (e.g., emotional processes, cognitive functioning, motor behaviour) underlying human performance (for a review see Hanin, 2007). In this context, Bortoli et al. (2012) recently proposed the multi-action plan (MAP) model based on empirical evidence that different performance levels are associated with unique behavioural, psychophysiological, and neurological patterns (Bertollo et al., 2013; Comani et al., 2014a). According to Bortoli et al., a fundamental assumption in the MAP model is a 2 × 2 (performance by control) relationship in which optimal and suboptimal levels of performance interact with high and low levels of action control (i.e., controlled vs. automated task execution). Consistent with this conceptualization, behavioural and psychophysiological patterns underlying distinct performance levels and attentional demands can be classified into four performance experiences: optimal-automatic, optimal-controlled, suboptimal-controlled, and suboptimal-automatic. Optimal-automatic performance experience (Type 1) is characterized by action “supervision” (i.e., parallel rather than serial processing) and smooth execution (Ericsson, 2003; Jackson and Csikszentmihalyi, 1999). Optimal-controlled performance (Type 2) is typified by an effective reinvestment of attention to core movement components that are not completely automated. Type 2 performance is likely in situations of distress, competitive anxiety and fatigue, when reinvestment of cognitive resources tends to occur (Masters and Maxwell, 2008). Noteworthy, when experiencing Type 2 performance states, athletes benefit from adopting an action-centred coping approach (Hanin and Hanina, 2009), in which a small number of specific core components of action are used to focus attention and improve performance. In pistol shooting, for example, the athlete can identify any element or behaviour encompassing the chain of movement as a core component. For example, these elements may include “stance and balance”, “sighting” and “triggering”. Mistakes and distress tend to result in suboptimal-controlled performance (Type 3), especially if an athlete lacks relevant experience and coping skills. The Type 3 performance state is typified by task-irrelevant focus of attention or excessive conscious control of movement execution and, as a consequence, undermined fluidity and automaticity of action (Maxwell et al., 2000; Oudejans et al., 2011). Finally, suboptimal-automatic performance (Type 4) can occur because of low levels of involvement, interest, energy, effort in task execution, attentional focus, and movement coordination (for more details see Bortoli et al., 2012). Recently, Bertollo et al. (2013) found that the four performance states were mirrored in both physiological (e.g., skin conductance responses, heart rate) and behavioural markers (e.g., kinematic patterns). Furthermore, Comani et al. (2014a) observed different neural patterns associated with the MAP model’s 2 × 2 performance types. In particular, an optimal-automatic performance state among shooters was characterized by lower Alpha power in the somato-sensory, contralateral parietal, and occipital areas (at shot release), in agreement with the neural efficiency hypothesis (i.e., global decrease in cortical activity). Conversely, optimal-controlled performance was characterized by increased Alpha power in the frontal and occipital areas. In the present study, we investigated neural markers of optimal and suboptimal performance states according to the MAP model’s tenets. Neurophysiological mechanisms in general, and cortical activity in particular, are proposed to be at the core of an integrated view of human performance (Del Percio et al., 2009; Hatfield and Kerick, 2007). Electroencephalographic (EEG) measurements have been useful in shaping our understanding of skilled performance in sports (Hatfield and Kerick, 2007; Nakata et al., 2010). In particular, Event Related Desynchronization/Synchronization (ERD/ERS) analysis has been widely used in sport settings to examine how functional changes in cortical activity influence performance in self-paced tasks, such as shooting and putting in golf (Babiloni et al., 2008; Del Percio et al., 2009; Hatfield and Kerick, 2007). In a seminal investigation of cortical activation in self-paced tasks, Bird (1987) found a correlation between successful shooting performance and lower-frequency EEG activity. Salazar et al. (1990) also observed a “quiescence” state (i.e., higher amplitude in Alpha band) prior to successful shots in archery. More recently, Del Percio et al. (2009) observed that the visuo-motor performance of elite shooters is associated with a global decrease in cortical activity. Thus, skilled performance in various self-paced sports seems to be accompanied by a decreased cortical activation immediately before task execution, according to the economy of effort principle or the neural efficiency hypothesis of psychomotor performance (see Haier et al., 1988; Hatfield and Kerick, 2007; Vecchio et al., 2012). The neural efficiency hypothesis of psychomotor performance stems from experimental evidence suggesting that skilled motor performance in self-paced sports is accompanied by a decrease in cortical activation (Babiloni et al., 2008; Haier et al., 1988; Hatfield and Kerick, 2007). It is also worth noting that EEG studies on attentional control and emotional regulation have focused on comparing athletes of different skill levels (i.e., the expert-novice paradigm) or skill levels within sports (i.e., expert performance approach) through a nomothetic approach. Together with nomothetic investigations, idiographic studies are also fundamental to advancing our understanding of the mechanisms underlying expertise. For instance, the pervasive deliberate practice theory has been validated through single-case studies, such as that of the memoirist Rajan Mahadevan, which demonstrated that “skilled memory” is an acquired rather than innate ability (Ericsson et al., 2004; Ericsson, 2006). Furthermore, the well-established individual zones of optimal functioning (IZOF) framework has been shaped through idiosyncratic analysis and single-case designs (Hanin, 2007). The importance of case studies in the advancement of sport psychology has been recently addressed in the literature. For example, Barker et al. (2013) emphasized that single-case designs allow researchers working in applied settings and with small samples to (a) identify applied principles and orient practice for both team and individual sports, and (b) develop applied procedures to assess intervention success. In the present investigation, we explored whether the different performance types described in the MAP model were associated with unique neural patterns. Our participant was an Olympic athlete with a rich history of successful experiences as recognized through top-level achievements (i.e., air-pistol shooting medallist in a number of international competitions). By means of ERD/ERS analysis we aimed to test four hypotheses. Specifically, we expected to find: (1) optimal-automatic performance experiences (Type 1) typified by an effective, minimal conscious control level matching task demands, and cortical activity synchronized with the event (i.e., the shot); (2) optimal-controlled experiences (Type 2) characterized by consciously focused control and cortical de-synchronization; (3) suboptimal-controlled experiences (Type 3) typified by a high level of conscious control with cortical activity completely desynchronized with the event; and (4) suboptimal-automatic experiences (Type 4) characterized by ineffective, minimal conscious control, despite a cortical activity synchronized with the event.
ParticipantThe participant was a 30-year-old male air-pistol shooter. He was a member of the Italian national team and had participated in numerous major international events, including the European and World Championships, the World Cup Championships, and the 2012 London Olympic Games. The shooter was accustomed with mental preparation programmes and, at the time of the study, was receiving mental training guidance from a senior sport psychologist. After learning about the purposes of the study, he agreed to participate and signed a written informed consent. The study conformed to the declaration of Helsinki and was approved by the local Institutional Review Board.
ProcedureThis case study involved three steps. First, according to the procedure developed by Bortoli et al. (2012), the participant was asked to detail his shooting action by providing a precise description of the chain of actions and behaviours related to his best shooting execution. He described the elements perceived as very important for his shooting action as: “good stance and balance”, “solid grip”, “vertical lift of the gun”, “attention focus on the front sight (i.e., aiming)”, “soft triggering”, “timing”, and “follow-through”. Then, the athlete was asked to identify a single core component of his shooting action that was not always executed in a completely automated mode, especially under distressful situations, and consequently needed to be kept under intentional control to enable a consistent and accurate execution (Bortoli et al., 2012). After reflecting on his shooting action, the participant selected “aiming” as his core component of action. The second step involved a warm-up period in a shooting range, after which the shooter was asked to perform 120 shots to the target, using a standard 4.5 calibre air-pistol. The participant was free to choose his “resting time” between consecutive shots and could relax using all the time he felt necessary. The distance between the shooter and the target was 10 m, and the diameter of the target was 6 cm in accordance with the international rules by the International Shooting Sport Federation (www.issf-sports.org). In pistol shooting, the width of the 9 score ring in the target is 27.5 mm, and the width of the 10 score ring is 11.5 mm. An electronic scoring target recorded each shooting score automatically. Shooting scores, recorded in decimal numbers, could range from 0 to 10.9. Among elite level shooters, scores can realistically vary from 8 to 8.9 (very poor performance; uncommon among high level athletes), from 9 to 9.9 (poor performance), and from 10 to 10.9 (good performance). The athlete was allowed to access the performance information (displayed on an LCD monitor) shot by shot, after assessment of his performance-related perceptions (see third step). For the third step, the shooter was asked to evaluate his hedonic tone prior to each shot using a Borg scale ranging from 0 (neither pleasant nor unpleasant) to ±11 (extremely pleasant or unpleasant), with negative scores being attributed to unpleasant states (see Pellizzari et al., 2011). After each shot, the participant was asked to report his perceived (a) control level on the core component of action (aiming); and (b) accuracy level on the execution of the core component. Both perceived control and accuracy were measured on the Borg scale ranging from 0 to 11, akin to previous studies in sport and exercise psychology (Bertollo et al., 2013; Comani et al., 2014a, 2014b). A feature of the scale is the congruence between numbers and verbal anchors (e.g., if a score corresponding to “very, very much” is rated 10, then an intensity corresponding to “much” is rated 5 to imply half that intensity). Single-item scores range from 0 to 11. Specifically, the verbal anchors were: 0 = nothing at all, 0.5 = very, very little, 1 = very little, 2 = little, 3 = moderate, 5 = much, 7 = very much, 10 = very, very much, • = maximum possible. No verbal anchors were assigned to 4, 6, 8 and 9 (Borg, 2001). After each shot, the shooter was allowed to switch on the monitor to check his actual score.
EEG recordingsElectroencephalographic data were recorded using the 32 channels EEG ASAlab system with Waveguard cap (Advanced Neuro Technology, Enschede, Netherlands). This system is supplied with shielded wires to make recordings less susceptible to external noise and movements. EEG data were continuously recorded with 1024 Hz sampling frequency. The ground electrode (AFz) and common average reference was positioned between Fpz and Fz to ensure low impedance values (generally < 5 Kθ). The 32 electrodes were distributed over the scalp according to the 10/5 system (Oostenveld and Praamstra, 2001). An electronic microphone with a sampling frequency of 1024 Hz using the Power lab 16/30 acquisition system (ADInstruments, Australia) was synchronized with the EEG system and was used to identify the precise instant of shot release.
Data analysisPerformance categorization: The participant’s shooting scores and perceived control levels were used to categorize the EEG epochs into a 2 × 2 matrix using the median split technique to identify the four types of performance as defined in the MAP model. Following this technique, shooting results ≥ 10.2 were categorized as optimal and the remaining scores as suboptimal. Attentional control levels ≤ 4 were categorized as automatic performance and the others as controlled performance. Therefore, when the shooting result was > 10.2 and the control level was < 4, performance was considered as optimal-automatic, and coded as Type 1 (a total of 16 events fell in this category). When the shooting result was > 10.2 and the control level was > 4, performance was classified as optimal-controlled (Type 2; 42 events). When the shooting result was < 10.2 and the control level was > 4, performance was categorised as suboptimal-controlled (Type 3; 11 events). Finally, when the shooting result was < 10.2 and the control level was < 4, performance was classified as suboptimal-automatic (Type 4; 51 events). The majority of the events fell within Type 2 or Type 4 performance categories. This may reflect the individual’s difficulty of reaching and maintaining a Type 1 performance flow-like state, as well as the relatively low level of stress experienced during assessment compared to competitive events in which a Type 3 performance state is more likely to occur. EEG pre-processing: EEG data were band-pass filtered between 0.2 to 40 Hz and segmented into epochs of 10 s duration, with each epoch starting at -7 s and ending at +3 s with respect to the instant of shot release (t = 0). EEG epochs showing instrumental, ocular and muscular artefacts were identified through visual inspection and corrected using the artefact correction tool available in the Asa software (Zanow and Knösche, 2004). EEG epochs with residual artefacts were not considered for further analysis. Event-Related Desynchronization/Synchronization (ERD/ERS): The event-related changes were quantified in the Theta (4-8 Hz), low Alpha (8-10 Hz), high Alpha (10-12 Hz), and Beta (16-24 Hz) bands. Low and high Alpha bands were defined with respect to the Individual Alpha Peak of the participant (10 Hz), as suggested by Nakata and colleagues (2010). The Beta band peak was set at 20 Hz (± 4 Hz range) as the first harmonic of the Individual Alpha Peak of the participant (for a review about the relationship between Alpha and Beta see Klimesch, 2012). Results for the other two Beta sub-bands (12-16 and 24-30 Hz) are available in the Supplemental online material (Available at URL: http://dx.doi.org/10.6084/m9.figshare.1335990). The individual ERD/ERS maps were calculated following the procedure proposed by Zanow and Knösche (2004) and implemented in the ASA software (Advanced Neuro Technology, Enshede, Netherlands). Specifically, ERD and ERS were defined as the percent variations of signal power with respect to the baseline. From the definitions given in Zanow and Knösche (2004), it follows that ERD results in a relative increase of signal power, whereas ERS results in a relative decrease of signal power with respect to the baseline. For each defined frequency band, the ERD/ERS maps were calculated by averaging the values obtained from each EEG channel and in respect to each trial. This computation was conducted for the following three intervals before shot release: T1=[-3 s,-2 s], T2=[-2 s,-1 s], T3=[-1 s, 0 s]. Of note, the baseline was defined in the interval [-5 s,-4 s], as intervals prior to -5 s were affected by body movements, small adjustments of head/trunk, and respiration artefacts (see Del Percio et al., 2009).
Behavioural analysisDescriptive statistics for performance, perceived levels of control, accuracy, and hedonic tone are given in Table 1. The correlation coefficient between perceived accuracy ratings and shooting outcomes was .75, thereby suggesting that the idiosyncratic core component was relevant for the shooter’s performance. Consistent with the MAP model categorization, we observed higher perceived accuracy levels for optimal performance states (Type 1 and Type 2 categories), and lower values for suboptimal states (Type 3 and Type 4 categories). Moreover, we observed higher control levels for Type 2 and Type 3 performance categories than for Type 1 and Type 4. Perceived hedonic tone was comparable across categories.
ERD/ERS analysisERD/ERS analysis, which is time-locked to the event and highly frequency-band specific (Pfurtscheller, 2001), revealed differences in cortical activity across performance types and frequency bands. Figure 1, Figure 2, and Figure 3 represent the topographical ERD/ERS maps based on data from the 30 electrodes (M1 and M2 are excluded because of interference from muscular artefacts), and for the theta, alpha, and beta bands akin to previous research in sport psychology (Del Percio et al., 2009). Temporal dynamics and topographic maps for all performance types in each frequency band are available in the supplemental materials (Available at URL: http://dx.doi.org/10.6084/m9.figshare.1335990). Theta band: ERD/ERS analysis in the Theta band (see Figure 1 and video in the supplemental material) revealed that Type 1 performance was mainly characterized by lower ERD and higher ERS in the bilateral pre-frontal and temporal areas during T1 and T2, which also involved the fronto-central and parietal areas during T3. For Type 2 performance, we observed a higher ERS in the left parietal area during T1, which became more evident and included the somato-sensory areas during T2. During T3, this ERS activity was reduced, and a higher ERD appeared in the right parietal areas. Type 3 performance showed a different pattern, with ERD in the pre-frontal, frontal and temporo-parietal areas during T1 more marked in the left regions. This pattern persisted, although decreasing in amplitude, until shot release. ERS was also observed in the occipital areas throughout all intervals. Finally, Type 4 performance was typified by a bilateral ERS in the frontal areas, which started during T1 and became more intense (involving also the fronto-central areas) until shot release, similar to Type 1 performance. Low Alpha band: The results of ERD/ERS analysis in the low Alpha band are shown in Figure 2, left panels and in the video. Type 1 performance was characterized by ERS in the prefrontal and frontal (mainly left) areas, and by an ERD in the left temporo-parietal areas. This pattern appeared during T1 and became more evident as shot release approached. Type 2 performance did not show any remarkable differences with respect to the baseline, except for a small ERD in the parietal areas (mainly right) and an ERS in the occipital areas during T3. As for the Theta band, Type 3 performance was characterized by a different pattern of activation, with a persistent ERD in the right frontal areas from T3 until shot, and by an ERS in the occipital areas that became more significant and expanded to the left frontal areas as shot release approached. Type 4 performance showed a pattern similar to Type 1 performance, although with less remarkable changes of ERD/ERS, a greater involvement of the occipital areas, and with a reduced involvement of the frontal areas. High Alpha band: The results of ERD/ERS analysis in the high Alpha band are shown in Figure 2, right panels. Type 1 performance was characterized by a stable ERS pattern in the prefrontal areas. This pattern increased and was accompanied by ERS in the occipital and right parietal areas during the last second before shot. A small ERD in the left and centro-parietal areas and in the right temporal area during T1 tended to increase during T2 and then to disappear before shot release. Type 2 performance did not show any significant differences with respect to the baseline during T1. A clear pattern was only noticeable during T3, with ERS in the pre-frontal, occipital and right centro-parietal areas, and ERD in the right parietal area. Type 3 performance was characterized by a marked ERD in the right fronto-temporo-parietal areas during T1. During T2, it was possible to observe ERS in the pre-frontal, parietal and occipital areas, and ERD in the right frontal areas, particularly evident during T3. Type 4 performance did not show significant changes with respect to the baseline during T3, except for a localized ERS in the occipital area particularly evident during T2 and T3. Just before shot release, further ERS was observed in the pre-frontal and right centro-frontal areas. Beta band: ERD/ERS analysis in the Beta band (see Figure 3), revealed that Type 1 performance was characterized by a clear ERS pattern in the right prefrontal and centro-parietal areas, that appeared during T1 and became more evident during T2 and T3. Just before shot release, a specific ERD in the centro-midline area was also observed. Type 2 performance was characterized by an ERS pattern similar to Type 1 performance, but less pronounced. Type 3 performance was characterized by a stable ERD in the right frontal area, and an ERS including the prefrontal, midline and occipital areas that appeared only during the second before shot release. Type 4 performance showed no significant changes with respect to the baseline until the/ ableast second before shot release, when an ERS pattern including the right prefrontal, parietal, and left occipital areas was observed.
In the present case study, we were interested in identifying cortical markers associated with optimal (Type 1 and Type 2) and suboptimal (Type 3 and Type 4) performance states. Our results revealed that optimal and suboptimal performance states were associated with different cortical patterns. Most importantly, Type 3 performance was characterized by an increase in theta ERD in the: (a) temporal left hemisphere, which is associated with verbal analytical processes; and (b) frontal midline theta area, which is associated with controlled attentional engagement. According to the reinvestment hypothesis and previous research on the link between attentional engagement and motor performance, this pattern of results suggests decreased automaticity in movement control (Kao et al., 2013; Masters and Maxwell, 2008). From visual inspection of the Figures and Video (supplemental material), it is possible to note different topographical patterns associated with Type 1, Type 2 and Type 4 performance states with respect to the theta, alpha and beta bands. These differences corroborate the MAP model tenets in the sense that different performance states are associated with unique neural patterns. The MAP model is aimed at capturing performance experiences in which an athlete is able to attain good outcomes without necessarily experiencing flow-like states, as often happens under the distressful conditions of competition (Hatfield, 2013). Indeed, in our study, Type 1 and Type 2 performance states showed different ERD/ERS patterns, thus indicating that good performance outcomes are not always characterized by neural efficiency (Babiloni et al., 2008; Vecchio et al., 2012). Our findings also support the notion that synchronized cortical activity (i.e., ERS) just before task execution (i.e., shot release) is associated with an automatic mode of functioning, which is typical of Type 1 (optimal-automatic) and Type 4 (suboptimal-automatic) performance states. In fact, both Type 1 and Type 4 performance states were typified by quiescence, automaticity and fluidity, which seem to be mirrored in the ERS patterns in the low Alpha band (8-10 Hz) usually associated with relaxation states (Wilson et al., 2011). Regarding the Theta band, the ERD/ERS pattern found might be related to a “default mode” network functioning, proper to autonomous skills and goal-relevant attentional focus upon approaching shot release (Kao et al., 2013; Raichle et al., 2001). The ideal performance state (Type 1) can be easily disrupted by stress, fatigue, or unexpected performance problems often found in competition. When athletes redirect their attentional focus on movement execution in the attempt to regain Type 1 performance, a drop to a suboptimal-controlled state (Type 3) is likely to occur. Excessive reinvestment in controlled processing undermines automaticity and is related to higher cortical activity in the attentional network, particularly in the parietal and frontal areas (Kao et al., 2013; Masters and Maxwell, 2008). We observed this cortical pattern in both Theta and high Alpha bands, which suggests a high level of attentional focus on movement control. In the Alpha band, we found a high level of attentional focus related to cortical de-synchronization among brain areas, which in turn was associated with poor motor performance (see Nakata et al., 2010). In self-paced sports, a step-by-step monitoring of a skill ultimately compromises one’s ability to focus on the present (“here and now”) and on relevant cues (Chuang et al., 2013; Kao et al., 2013). Our results can be interpreted in the framework of the neural efficiency hypothesis. According to Callan and Naito (2014), neural efficiency can reflect two different processes; “The first is a reduction in neural activity in certain brain regions as a particular skill becomes more automated and less controlled… The second is a reduction of activity in sensory and motor cortex, reflecting more efficient processing made possible by less energy expenditure…” (p. 183). In particular, the results obtained for Type 1 performance support the neural efficiency hypothesis, in which skilled performance is characterized by an effective activation of task specific brain areas (i.e., selective cortical activation during shooting; see Del Percio et al., 2009; Dunst et al., 2014; Hatfield and Kerick, 2007). Our results also lend support to previous research on the visuo-motor performance network of expert athletes showing a global synchronization in cortical activity just before task execution (Del Percio et al., 2009). More specifically, our findings concur with the observation that high Alpha rhythms are involved in visuo-motor performance in self-paced sports (e.g., air-pistol shooting), and mirror functional processes of thalamo-cortical and cortico-cortical loops facilitating or inhibiting the transmission and retrieval of sensorimotor and cognitive information in the brain (Pfurtscheller and Lopes da Silva, 1999). Furthermore, 2 s prior to shot release, Type 1 performance was marked by focused ERD activity in the left-parietal areas, consistent with the assumption that high-skilled preparation and movement execution involve the left parietal cortex (Wheaton et al., 2009). Type 3 performance findings concur with the evidence indicating relationships between: (1) low Alpha power and general cortical arousal, and (2) high Alpha power and task-relevant attentional processing (Hatfield, 2013; Pfurtscheller and Lopes da Silva, 1999). Specifically, we observed ERD patterns in low Alpha band for Type 3 performance, suggesting that higher levels of general cortical arousal were associated with suboptimal-controlled performance states. Moreover, we observed a pronounced ERD for the high Alpha band in the somato-sensory, right frontal, and parietal regions, which might indicate a reinvestment of attention proper to suboptimal performance states (Masters and Maxwell, 2008). Our results also suggest that Alpha and Beta ERS patterns are related to both Type 1 and Type 4 performance states. Specifically, Type 4 performance was characterized by a synchronization of cortical activity, whereas Type 2 performance (optimal-controlled) was marked by a minimum decrease of Alpha and Beta power. Collectively, these findings suggest that an elite shooter may attain good performance when consciously redirecting his attentional focus to a core component of action (Bertollo et al., 2013; Bortoli et al., 2012; Comani et al., 2014a). From an applied perspective, these results support the view that focusing attention on idiosyncratic core components of action can improve performance in distressful situations, whereas directing attention to the execution of automated actions can hamper the control processes that naturally regulate movement coordination (see Wulf, 2007). Conversely, focusing on a core component of the action can benefit performance as attentional focus is not reinvested in a step-by-step mode, but rather is directed at specific triggers of skilled motor execution. In fact, focusing on a core component of action tends to counteract the detrimental effects of a voluntary control of processes underlying execution (Schücker et al., 2014). Results for the Theta band revealed a focused ERD activity during Type 1 performance in frontal midline, with a clear distribution of ERS in the frontal and somato-sensory areas just prior to shot release. During Type 3 performance, ERD activity was particularly higher in the shooter’s left-frontal and temporal areas. Previous research comparing experts and novices has demonstrated that skilled shooters show increased frontal-midline Theta power during pre-performance periods, which suggests an increased allocation of attentional control resources (Baumesteir et al., 2008; Doppelmayr et al., 2008). From an applied standpoint, our findings corroborate the assumption that pre-performance routines may benefit the athlete’s control of attentional resources prior to movement execution. For instance, an athlete may benefit from arousal, attention, and emotion regulation strategies to increase Theta activity and modulate low and high Alpha activity (Konttinen et al., 2000; Wilson et al., 2011).
Altogether, our findings support the MAP model tenets. Optimal-automatic (Type 1) and suboptimal-controlled (Type 3) states were underpinned by distinct neural activity patterns. Furthermore, we observed that good performance might occur in the absence of automated motor behaviour (Type 2 performance). As such, a task-relevant focus on the core components of the action can enable the athlete to recover from suboptimal performance levels. Future research should address some limitations of our study. First, inter-subject validation is needed to increase the generalizability of our findings. Second, attempts should be made to induce Type 1 flow-like states as well as Type 2 performance states. Type 1 states are difficult to reach in controlled settings because peak experiences are rare and ephemeral. In addition, the competitive pressure that can engender Type 3 states is not easily attainable during practice. Advancement in EEG technology, including wireless features and dry electrodes, might be implemented during competition to assess suboptimal states in more ecological settings.
|
AUTHOR BIOGRAPHY |
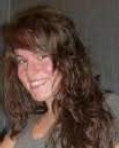 |
Selenia di Fronso |
Employment: Post Doc Research Fellow, BIND - Behavioral Imaging and Neural Dynamics Center, Department of Medicine and Aging Sciences, University “G. D’Annunzio” of Chieti-Pescara, Italy |
Degree: PhD |
Research interests: Sport Psychology, Performance Optimization, Psychophysiology, EEG and Emotion in sports |
E-mail: s.difronso@gmail.com |
|
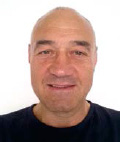 |
Claudio Robazza |
Employment: Associate Professor, BIND - Behavioral Imaging and Neural Dynamics Center, Department of Medicine and Aging Sciences, University “G. D’Annunzio” of Chieti-Pescara, Italy |
Degree: PhD |
Research interests: Sport psychology, performance optimization, emotion, motivation, motor control, motor learning |
E-mail: c.robazza@unich.it |
|
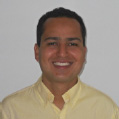 |
Edson Filho |
Employment: Lecturer in Sport and Exercise Psychology, School of Psychology, University of Central Lancashire, UK. |
Degree: PhD |
Research interests: Spot Psychology, peak performance experiences, team processes, and social neuroscience. |
E-mail: efilho@uclan.ac.uk |
|
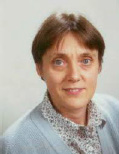 |
Laura Bortoli |
Employment: Assistant Professor, BIND - Behavioral Imaging and Neural Dynamics Center, Department of Medicine and Aging Sciences, University “G. D’Annunzio” of Chieti-Pescara, Italy |
Degree: MSc |
Research interests: Sport and exercise psychology, physical education, performance optimization, emotion, motivation and motor learning |
E-mail: |
|
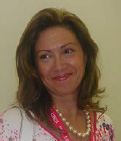 |
Silvia Comani |
Employment: Associate Professor in Applied Physics, Director of the BIND - Behavioral Imaging and Neural Dynamics Center and affiliated to the Department of Neuroscience, Imaging and Clinical Sciences, both at University “G. D’Annunzio” of Chieti-Pescara, Italy |
Degree: PhD |
Research interests: Linear and nonlinear biomedical signal processing; Development of novel EEG system technology; Functional imaging of the neural basis of motor development, learning and control. |
E-mail: comani@unich.it |
|
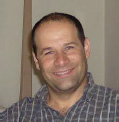 |
Maurizio Bertollo |
Employment: Associate Professor in Motor Behaviour, BIND - Behavioral Imaging and Neural Dynamics Center, Department of Medicine and Aging Sciences, University “G. D’Annunzio” of Chieti-Pescara, Italy |
Degree: PhD |
Research interests: Sport and exercise psychophysiology, performance optimization, bio-neuro feedback, motor learning and control |
E-mail: m.bertollo@unich.it |
|
|
|
REFERENCES |
 Babiloni C., Del Percio C., Lacoboni M., Infarinato F., Lizio R., Marzano N., Crespi G., Dassù F., Pirritano M., Gallamini M., Eusebi F. (2008) Golf putt outcomes are predicted by sensorimotor cerebral EEG rhythms. Journal of Physiology 586, 131-139.
|
 Barker J.B., Mellalieu S.D., McCarthy P.J., Jones M.V., Moran A. (2013) A review of single-case research in sport psychology 1997-2012: Research trends and future directions. Journal of Applied Sport Psychology 25, 4-32.
|
 Baumeister J., Reinecke K., Liesen H., Weiss M. (2008) Cortical activity of skilled performance in a complex sports related motor-task. European Journal of Applied Physiology 104, 625-631.
|
 Bertollo M., Bortoli L., Gramaccioni G., Hanin Y., Comani S., Robazza C. (2013) Behavioural and psychophysiological correlates of athletic performance: A test of the multi-action plan model. Applied Psychophysiology and Biofeedback 38, 91-99.
|
 Bird E.I. (1987) Psychophysiological processes during rifle shooting. International Journal of Sport Psychology 18, 9-18.
|
 Borg G (2001) Borg’s range model and scales. International Journal of Sport Psychology 32, 110-126.
|
 Bortoli L., Bertollo M., Hanin Y., Robazza C. (2012) Striving for excellence: A multi-action plan intervention model for shooters. Psychology of Sport and Exercise 13, 693-701.
|
 Callan D.E., Naito E. (2014) Neural processes distinguishing elite from expert and novice athletes. Cognitive Behavioural Neurology 27, 183-188.
|
 Chuang L.Y., Huang C.J., Hung T.M. (2013) The differences in frontal midline Theta power between successful and unsuccessful basketball free throws of elite basketball players. International Journal of Psychophysiology 90, 321-328.
|
 Comani S., Bortoli L., Di Fronso S., Filho E., De Marchis C., Schmid M., Conforto S., Robazza C., Bertollo M. (2014a) ERD/ERS patterns of shooting performance within the multi-action plan model. IFMBE Proceedings 41, 141-144.
|
 Comani S., Di Fronso S., Filho E., Castronovo A.M., Schmid M., Bortoli L., Conforto S., Robazza C., Bertollo M. (2014b) Attentional focus and functional connectivity in cycling: An EEG case study. IFMBE Proceedings 41, 137-140.
|
 Del Percio C., Babiloni C., Bertollo M., Marzano N., Iacoboni M., Infarinato F., Lizio R., Stocchi M., Robazza C., Cibelli G., Comani S., Eusebi F. (2009) Visuo-attentional and sensorimotor alpha rhythms are related to visuo-motor performance in athletes. Human Brain Mapping 30, 3527-3540.
|
 Doppelmayr M., Finkenzeller T., Sauseng P. (2008) Frontal midline Theta in the pre-shot phase of rifle shooting: Differences between experts and novices. Neuropsychologia 46, 1463-1467.
|
 Dunst B., Benedek M., Jauk E., Bergner S., Koschutnig K., Sommer M., Ischebeck A., Spinat B., Arendasy M., Buhner M., Freudenthaler H., Neubauer A. C. (2014) Neural efficiency as a function of task demands. Intelligence 42, 22-30.
|
 Ericsson K.A. (2006) The Cambridge handbook of expertise and expert performance. New York. Cambridge University Press.
|
 Ericsson K.A., Delaney P.F., Weaver G., Mahadevan R. (2004) Uncovering the structure of a mnemorist’s superior “basic” memory capacity. Cognitive Psychology 49, 191-237.
|
 Ericsson K.A., Starkes J.L., Ericsson K.A. (2003) Expert performance in sports: Advances in research on sport expertise. Development of elite performance and deliberate practice: an update from the perspective of the expert performance approach. Champaign. Human Kinetics.
|
 Furley P., Schweizer G., Bertrams A. (2015) The two modes of an athlete: Dual process theories in the field of sport. International Review of Sport and Exercise Psychology 8, 106-124.
|
 Haier R.J., Siegel B.V., Nuechterlein K.H., Hazlett E., Wu J.C., Paek J., Browning H.L., Buchsbaum M.S. (1988) Cortical glucose metabolic rate correlates of abstract reasoning and attention studied with positron emission tomography. Intelligence 12, 199-217.
|
 Hanin Y., Hanina M. (2009) Optimization of performance in top-level athletes: An action-focused coping approach. International Journal of Sports Science and Coaching 4, 47-91.
|
 Hanin Y.L., Tenenbaum G., Eklund R. C. (2007) Handbook of sport psychology. Emotions in sport: Current issues and perspectives. Hoboken, NJ. Wiley.
|
 Hatfield B.D., Costanzo M.E., Godman R.N., Lo L.C., Oh H., Rietschel J.C., Saffer M., Bradbeerry T., Cotreras-Vidal J., Haufler A. (2013) The influence of social evaluation on cerebral cortical activity and motor performance: A study of “real-life” competition. International Journal of Psychophysiology 90, 240-249.
|
 Hatfield B.D., Kerick S.E., Tenenbaum G., Eklund R. C. (2007) Handbook of sport psychology. The psychology of superior sport performance: a cognitive and affective neuroscience perspective. Hoboken, NJ. Wiley.
|
 Jackson S.A., Csikszentmihalyi M. (1999) Flow in sports: The keys to optimal experiences and performances. Champaign. Human Kinetics.
|
 Kao S.C., Huang C.J, Hung T.M. (2013) Frontal midline Theta is a specific indicator of optimal attentional engagement during skilled putting performance. Journal of Sport and Exercise Psychology 35, 470-478.
|
 Klimesch W (2012) α-band oscillations, attention, and controlled access to stored information. Trends in Cognitive Sciences 16, 606-617.
|
 Konttinen N., Landers D.M., Lyytinen H. (2000) Aiming routines and their electrocortical concomitants among competitive rifle shooters. Scandinavian Journal of Medicine and Science in Sports 10, 169-177.
|
 Masters R., Maxwell J. (2008) The theory of reinvestment. International Review of Sport and Exercise Psychology 1, 160-183.
|
 Maxwell J.P., Masters R.S.W., Eves F.F. (2000) From novice to no know-how: A longitudinal study of implicit motor learning. Journal of Sports Sciences 18, 111-120.
|
 Nakata H., Yoshie M., Miura A., Kudo K. (2010) Characteristics of the athletes’ brain: Evidence from neurophysiology and neuroimaging. Brain Research Reviews 62, 197-211.
|
 Oostenveld R., Praamstra P. (2001) The five percent electrode system for high resolution EEG and ERP measurement. Clinical Neurophysiology 112, 713-719.
|
 Oudejans R.R.D., Kuijpers W., Kooijman C.C., Bakker F.C. (2011) Thoughts and attention of athletes under pressure: skill-focus or performance worries?. Anxiety, Stress, and Coping 24, 59-73.
|
 Pellizzari M., Bertollo M., Robazza C. (2011) Pre- and post-performance emotions in gymnastics competitions. International Journal of Sport Psychology 42, 278-302.
|
 Pfurtscheller G (2001) Functional brain imaging based on ERD/ERS. Vision Research 41, 1257-1260.
|
 Pfurtscheller G, Lopes da Silva F.H. (1999) Event-related EEG/MEG synchronization and desynchronization: Basic principles. Clinical Neurophysiology 110, 1842-1857.
|
 Raichle M.E., MacLeod A.M., Snyder A.Z., Powers W.J., Gusnard D.A., Schulman G.L. (2001) A default mode of brain function. Proceedings of the National Academy of Sciences of the United States of America 98, 676-682.
|
 Robazza C, Hanton S., Mellalieu S. D. (2006) Literature Reviews in Sport Psychology. Emotion in sport: An IZOF perspective. New York, NY. Nova Science.
|
 Salazar W., Landers D.M., Petruzzello S.J., Han M., Crews D.J., Kubitz K.A. (1990) Hemispheric asymmetry, cardiac response, and performance in elite archers. Research Quarterly for Exercise and Sport 61, 351-359.
|
 Schücker L., Knopf C., Strauss B., Hagemann N. (2014) An internal focus of attention is not always as bad as its reputation: How specific aspects of internally focused attention do not hinder running efficiency. Journal of Sport and Exercise Psychology 36, 233-243.
|
 Silverstein R, Nunez P. L. (1995) Neocortical dynamics and human EEG rhythms. Neuromodulation of neocortical dynamics. New York, NY. Oxford University Press.
|
 Vecchio F., Del Percio C., Babiloni C. (2012) Is there a neural efficiency in the athlete’s brain?. International Journal of Psychophysiology 85, 296-297.
|
 Wheaton L., Fridman E., Bohlhalter S., Vorbach S., Hallett M. (2009) Left parietal activation related to planning, executing and suppressing praxis movements. Clinical Neurophysiology 120, 980-986.
|
 Wilson W.S., Thompson M., Thompson L., Peper E., Strack B., Linden M., Wilson V. S. (2011) Biofeedback and neurofeedback applications in sport psychology. Using EEG for enhancing performance: Arousal, attention, self talk, and imagery. Wheat Ridge, CO. American Association Psychophysiology and Biofeedback.
|
 Wilson W.S., Thompson M., Thompson L., Thompson J., Fallahpour K., Strack B., Linden M., Wilson V. S. (2011) Biofeedback and neurofeedback applications in sport psychology. Introduction to EEG biofeedback neurofeedback. Wheat Ridge, CO. American Association Psychophysiology and Biofeedback.
|
 Wulf G (2007) Attention and motor skill learning. Champaign. Human Kinetics.
|
 Zanow F., Knösche T.R. (2004) Asa-advanced source analysis of continuous and event-related eeg/meg signals. Brain Topography 16, 287-290.
|
|
|
|
|
|
|