|
|
|
ABSTRACT |
The primary aim of the study was to determine if heart rate variability (HRV), and heart rate recovery (HRR) are related to several subjective indicators of recovery status (muscle soreness, hydration status, sleep quality and quantity as well as pre-competition mood states) for different match periods in male, elite, African, singles badminton players. HRV and HRR were measured in twenty-two badminton players before (pre-match), during (in-match), after (post-match) and during rest periods (in-match rest) of 46 national and international matches. Muscle soreness, hydration status, and sleep quality and quantity were measured on a daily basis whereas mood states were measured just before each match via questionnaires. Prior to each match warm-up, players were fitted with a Fix Polar Heart Rate Transmitter Belt to record heart rate every second during each match and HRR during service breaks and after matches. Kubios HRV software was used for final HRV analyses from the series of R-R-intervals. A strong, significant canonical correlation (Rc = 0.96, p = 0.014) was found between HRV, HRR and subjective indicators of recovery status for the in-match period, but only strong, non-significant relationships were observed for pre-match (Rc = 0.98, p = 0.626) and post-match periods (Rc = 0.98, p = 0.085) and a low non-significant relationship (Rc = 0.69, p = 0.258) for the in-match rest period. Canonical functions accounted for between 47.89% and 96.43% of the total variation between the two canonical variants. Results further revealed that Ln-HFnu, the energy index and vigour were the most prominent variables in the relationship between the autonomic markers of heart rate and recovery-related variables. In conclusion, this study proved that subjective indicators of recovery status influence HRV and HRR measures obtained in a competitive badminton environment and should therefore be incorporated in protocols that evaluate these ANS-related parameters. |
Key words:
Heart rate variability, heart rate recovery, subjective recovery questionnaires, racquet sports
|
Key
Points
- Subjective recovery indicators do in fact influence short-term HRV and HRR values measured during a real badminton competitive environment.
- Practitioners are advised to incorporate subjective recovery indicators in their protocols when evalua-ting HRV and HRR in competitive conditions to avoid clouded and obscure results.
- Mood state-related variables such as energy index and vigour as measured by Lickert scale-based questionnaires should be incorporated as recovery indicators as they have the biggest influence on HRV-related values during badminton competition participation.
|
The emergence of heart rate variability (HRV) and heart rate recovery (HRR) as indicators of autonomic nervous system (ANS) activity has stimulated research in sport science (Buchheit, 2014). However, HRV and HRR measuring protocols should adhere to certain guidelines in order to ensure the accuracy, validity and reliability of HRV and HRR measures in sport and exercise settings (Plews et al., 2014). Although existing guidelines do provide some direction with regard to the measurement of autonomic markers of heart rate, various factors that are related to competition participation and could negatively influence HRV and HRR measures, are often not considered. In this regard valid and widely used subjective indicators of recovery status such as muscle soreness, hydration status, sleep quality and quantity as well as pre-competition mood states may influence HRV and HRR measures in such a way that the accuracy, validity and reliability of these cardiac autonomic measures are questioned (Buchheit et al., 2013; Stanley et al., 2013; Plews et al., 2012). Nevertheless, available studies that have explored these potential associations have several shortcomings such as, only focusing on one of the aforementioned indicators at a time, the use of a small number of HRV-related measures, the exclusion of the HRR measure and the use of non-athletes as study participants. Oliveira et al. (2011) established that the root mean square of differences between successive R-R intervals (RMSSD), as well as the standard deviation of R-R intervals (SDNN) were significantly higher in a group who ingested 500 ml of water after a 20-minute, sub-maximal, cycle test compared to a group who ingested no water. They therefore concluded that water intake had a positive effect on post-exercise HRV (Oliveira et al., 2011). Researchers also showed that sleep deprivation caused a significant increase in vagal activity [i.e., high frequency power (HF) and low frequency power (LF)] and a significant decrease in heart rate over a period of 60 hours in physically fit adults (Vaara et al., 2009). Furthermore, a psychological mood related factor, namely anxiety, produced significant decreases in RMSSD, LF:HF ratio and normalised LF power (LFnu) in high level swimmers during competition periods when higher pre-competitive anxiety levels were experienced (Blasquez and Ortis, 2009). In addition, Buchheit et al. (2013) found that increased daily muscle soreness was significantly, negatively correlated (r = -0.53) with the natural logarithmic transformation of standard descriptor one of a Poincare plot (ln-SD1) in professional Australian football players throughout a training camp. Last-mentioned findings suggest that athletes’ hydration status, muscle soreness, sleep quality and quantity as well as pre-competition mood state levels will significantly influence HRV-related measures. The presumed parasympathetic origin of both HRR and vagal-related HRV indices as well the esta-blishment of moderate relationships between vagally mediated measures of HRV and HRR immediately after exercise (Buchheit et al., 2007; Nunan et al., 2010), would suggest that the above-mentioned recovery indicators would have a similar effect on HRR. However, several researchers reported no relationships between absolute and relative HRR at the 1st minute post-exercise with HRV parameters (Javorka et al., 2002; Molina et al., 2016). In contrast, significant positive correlations were observed between relative HRR and all assessed time and frequency domain HRV parameters measured from the 5th and 10th minute of recovery post-exercise (Javorka et al., 2002). Last-mentioned findings would suggest that parasympathetic outflow differentially affect HR level (HRR) and HR modulation (HRV), although proof for this contention must still be found (Buchheit et al., 2007). Thus, the extent to which above-mentioned recovery indicators affect HRV and HRR is still unknown since both markers appear to be independently linked to ANS control (Esco et al., 2010). Up until now, researchers made use of subjective questionnaires to measure muscle soreness, hydration status, sleep quality and quantity as well as pre-competition mood states of participants. For example, Gastin et al. (2013) reported significant pre- versus post-differences in muscle fatigue, sleep quality, stress and general well-being following training in elite rugby league players when subjective questionnaire ratings were used. Other researchers also suggested that self-reported player ratings are useful tools to monitor different aspects of recovery (mood states, quality of sleep, over-all fatigue, muscle soreness and hydration levels) during trai-ning and competition participation (Buchheit et al., 2013; Leti and Bricout, 2013; Lew et al., 2010; Saw et al., 2015). Consequently, it is necessary to determine if HRV and HRR are specifically related to several subjective indicators of recovery status (muscle soreness, hydration status, sleep quality and quantity as well as pre-competition mood states) for different match periods in male, elite, African, singles badminton players. Therefore the hypothesis of this study is that significant relationships exist between HRV, HRR and last-mentioned subjective indicators of recovery status for different match periods in male, elite, African, singles badminton players. The study may establish whether relationships exist as well as determine the size and direction of these relationships between last-mentioned variables. This is also the first study of which the outcome may provide HRV and HRR protocol guidelines to researchers, sport scientists and coaches who want to measure HRV and HRR in competitive badminton and other sport settings.
ParticipantsTwenty-two male, elite, African, singles badminton players (age 23.3 ± 3.9 years; height 1.77 ± 0.03 m; weight 83.4 ± 14.5 kg) who participated in the following championships during the 2014/2015 season gave written informed consent to participate in the study: All African Badminton Senior Championships, South African International Championships, Free-State National Championships, u/19 South African National Championships and the University Sport South Africa (USSA) Badminton Championships. Players represented ten African countries, namely Botswana, Cameroon, Congo, Egypt, Namibia, Nigeria, South Africa, Uganda, Zambia and Zimbabwe. According to definitions of Swann et al. (2015) players could be categorised into three categories, namely: competitive-elite players who regularly compete at the highest level but did not have any success at that level; successful-elite players who have experienced some (infrequent) success at the highest level and world-class elite players who sustained success at the highest level, with repeated wins over a prolonged period of time. Only players who were actively involved and competing as members of their respective teams and national badminton federations in above-mentioned tournaments as well as those who were totally injury free at the time of testing were eligible to participate in the study. Players’ competitive badminton playing experience ranged between 4 and 12 years (mean = 9.5 ± 2.7 years). The following information with regard to the training regimen of the badminton players was also obtained: players trained for 4 ± 1 days a week which consisted of on-court training for 4 ± 1 days a week and weight training for 2 ± 2 days a week. Approval for the study was obtained from the Health Research Ethics Committee of the institution where the research was conducted (NWU-00199-14-A1). The study was conducted according to the ethical guidelines and principles of the international Declaration of Helsinki and the ethical guidelines of the National Health Research Ethics Council of South Africa. Furthermore, permission to conduct the research was obtained from the Badminton World Federation (BWF), the Badminton Confederation of Africa (BCA), the Botswana Badminton Association (BBA), and Badminton South Africa (BSA).
Test procedureTwenty-two players were measured before, during and after 46 matches. Each player was therefore measured more less two times with several players being measured three times. All championships took place over a period of two to three days, except for the Free-State National Championships which took place over a day, which meant that players were monitored and measured on consecutive days. Every day before the start of each match, participants completed a recovery and hydration status as well as muscle soreness questionnaire during which researchers were present to assist players who had questions regarding the questionnaire. Participants also completed a general information questionnaire regarding their exer-cising habits, injury incidence and competing level. After completion of all relevant documentation, participants’ stature and body weight measures were taken. Ten minutes before the start of each match, players also completed the Stellenbosch Mood Scale (STEMS) (Terry et al., 2003) under the supervision and guidance of the research team. Prior to each match warm-up participants were fitted with a Fix Polar Heart Rate Transmitter Belt to record heart rate every second during each match. The warm-up period was also used to check the signals of the heart rate monitors before each match began, to make participants accustomed to the equipment and to adjust the heart rate transmitter belts according to each participant’s preference. Before the start of each match a video camera was stationed on a tri-pod stand behind each of the courts that matches were played on in order to make recordings. The test procedure is illustrated in Table 1.
Test components Demographic and general information questionnaireParticipants’ demographic and personal information was collected by means of the above-mentioned questionnaire. Participants’ ages, exercising habits, injury incidents, competing levels and best performances were also obtained by means of this questionnaire. Participants also had to indicate whether they were taking any medication or supplements during their participation in the tournament.
Anthropometric measurementsThe following anthropometric components were determined according to the protocols of the International Society for the Advancement of Kinanthropometry (ISAK) (Stewart et al., 2011) to describe the specific cohort of participants: Body mass to the nearest 0.1 kg, using a calibrated BFW 300 Platform scale (Adam equipment Co. Ltd., U.K.) and body stature to the nearest 0.1 cm, using a Harpeden portable stadiometer (Holtain Ltd., U.K.). Measurements were performed twice by a Level 2 ISAK certified Anthropometrist.
Heart rate variability (HRV)A Fix Polar Heart Rate Transmitter Belt along with a Polar Heart Rate Monitor (Polar Team2 Pro, Polar Electro, Kempele, Finland) were used to record variations in beat to beat intervals (R-R intervals) before, during and after matches. Polar Heart Rate Monitors have been shown to provide valid measures of heart rate and HRV when compared to ambulatory Holter ECG devices (Gamelin et al., 2006). The Polar Heart Rate Transmitter Belt was adjus-ted according to each participant’s chest size and tied around the chest, just below the chest muscles after the electrodes were moistened by tap water. A Polar Heart Rate Monitor was then clipped onto the Polar Heart Rate Transmitter Belt of each participant before he stepped onto the court to begin his pre-match warm-up routine. R-R-intervals were obtained for the following time periods: The first set of HRV-related measurements were taken 60 seconds before the match warm-up (pre-match) which took place 5 minutes before the start of each match. The next set of HRV-related measurements were calcula-ted by determining the average R-R values for each 60-second interval playing time during the entire match (in-match). The last set of R-R intervals was taken 60 seconds directly after play stopped (post-match). The first and third sets of HRV-related measurements were taken while participants stood upright and maintained a normal breathing pattern. Finally, R-R-intervals were also calculated for rest periods between sets which lasted a minimum of 60 seconds (in-match rest). This was made possible by synchronising the chronological time indexes of the digital video camera, Polar heart rate monitor, and wrist watches being used during data collection. After data collection researchers meticulously went through the video footage to identify and divide different periods of the match. Kubios HRV software (Version 2.1, Biosignal Analysis and Medical Imaging Group at the Department of Applied Physics, University of Kuopio, Kuopio, Finland) was used for final HRV analyses from the series of R-R-intervals obtained from Polar devices. This software has become popular and has been used in several studies that employed HRV analysis software (Nakamura et al., 2009; Perandini et al., 2009; Plews et al., 2014). Frequency domain-related indices were obtained by making use of the fast Fourier transform algorithm. Results of the following 14 HRV indices were obtained by making use of the above-mentioned software and used in subsequent statistical analyses: Time domain-related HRV indices consisting of:
The natural logarithmic transformation of the standard deviation of R-R intervals in milliseconds (Ln-SDNN);
The natural logarithmic transformation of the square root of the mean squared differences between successive R-R intervals in milliseconds (Ln-RMSSD) and
The mean of R-R intervals in milliseconds (Mean R-R).
Frequency domain-related HRV indices consisting of:
The very low band peak frequencies in hertz (Peak VLF Hz);
The low band peak frequencies in hertz (Peak LF Hz);
The high band peak frequencies in hertz (Peak HF Hz);
The natural logarithmic transformation of LF relative power expressed as normalised units (Ln-LFnu);
the natural logarithmic transformation of HF relative power expressed as normalised units (Ln-HFnu);
VLF relative power expressed as percentage (VLF %);
LF relative power expressed as percentage (LF %);
HF relative power expressed as percentage (HF %) and
The ratio between Ln-LFnu and Ln-HFnu components (Ln-LFnu/HF Ln-HFnu).
Non-linear domain related HRV indices consisting of:
The natural logarithmic transformation of Standard descriptor 1 (Ln-SD1) and
Standard descriptor 2 (Ln-SD2)
Heart rate recovery (HRR)HRR was calculated by determining the absolute value at 60 seconds (i.e., heart rate, (HR) at 1-min recovery subtracted from immediate post-HR) as well as percentage decline (i.e., HR at 1-min recovery divided by immediate post-HR multiplied by 100) for 60 seconds from the post-set or -match attained heart rate directly after play had stopped. However, in cases where a participant’s post-set or -match HR did not obtain a value above 85% of a participant’s theoretical maximal HR (208–0.7*age) (Roy and McCrory, 2015), the HRR measurement was discarded (Boullosa et al., 2013). Boullosa et al. (2013) successfully measured short-term HRR (20 seconds) from sub-maximal HR during periods of active recovery in soccer players who played small-sided soccer games. However, researchers only measured HRR in cases where players actively recovered for at least 20 seconds during which they did not exceed a walking pace of 4 km per hour. HRR was also not measured if players did not reach at least 85% of their theoretical maximal HR. HRR was considered a measure of parasympathetic reactivation (Daanen et al., 2012).
Recovery and hydration status as well as muscle soreness questionnaireEvery day before the start of each match, participants completed a general recovery and hydration status questionnaire that provided researchers with information regarding sleep quantity and quality of the previous night, current hydration status, as well as the degree of muscle soreness that a participant experienced at that moment in time. In order to determine sleep quantity and quality, players needed to indicate the number of hours that they slept the previous night (2-10 hours) as well as the quality of sleep by means of a 5-point Likert scale: “very poor”, “poor”, “average”, “good”, “very good”. Various studies have also used this method to report sleep quantity and quality successfully for a range of participants (Tavernier and Willoughby, 2014; Spira et al., 2012). Participants also indicated what the colour of their urine was when they last urinated. A 5-point Likert scale with the following categories was used for urine colour identification: “transparent”, “a shade of yellow”, “light yellow”, “dark yellow”, “very dark yellow”. Urine colour scale determination is a valid and practical means of determining hydration status in athletes due to the significant correlation that exists between the specific gravity of urine (which is a quantitative indicator of hydration status) and urine colour (Lew et al., 2010). In view that Trost et al. (2012) reported that muscle soreness can be determined by means of a 3-point Likert scale with the categories of “none”, “some”, or “a lot”, this method was also used in this study. Results of the first-mentioned questionnaire therefore aided in evaluating the overall recovery status of participants.
The Stellenbosch Mood Scale (STEMS)The STEMS was used to determine the pre-match mood states of each participant. The STEMS of Terry and co-workers (2003) is a dual language (English and Afrikaans) questionnaire which is a derivative of the Profile of Mood States (POMS) of McNair et al. (1971). The STEMS measures six subscales, i.e. Tension, Depression, Anger, Vigour, Fatigue and Confusion, with four items contributing to each subscale. Participants were requested to indicate “How are you feeling right now” in terms of 24 mood descriptors on a six-point Likert scale, anchored by descriptors ranging from “Not at all” [0], to “Extremely” [5]. Energy index (EI) was also determined by calculating the ratio of vigour to fatigue scores for each participant (Kenttä et al., 2006). Terry et al. (2003) showed that all six mood scale items of the STEMS provided acceptable internal consistency for all groups with alpha coefficient values that met or exceeded the 0.7 threshold of acceptability. A study in which the STEMS was used to measure the mood states of rugby union players (mean age: 22.26 ± 1.39 years) also reported acceptable internal consistencies for the data of these players, with Cronbach alpha values ranging between 0.65 and 0.87 (Grobbelaar et al. 2011). The criterion validity of the POMS-A (on which the STEMS is based) is supported due to relationships (r = 0.67-0.90, p < 0.05) with previously validated inventories such as the Positive and Negative Affect Schedule (PANAS), the State-Trait Anger-expression Inventory (STAXI) and the original Profile of Mood States inventory (POMS) (Terry et al., 2003). Previously researchers also found that the fatigue subscale of the POMS was significantly correlated (r = 0.72, p < 0.05) with frequency domain-related HRV indices (LFnu and HFnu, both r = 0.66, p < 0.05) over a 12 week training period in athletes (Leti and Bricout, 2013). A comprehensive review of Saw et al (2015) also concluded that va-rious studies observed significant relationships between subscales of the POMS and HRV. Participants completed the STEMS ten minutes before each match under the supervision of a research team member who was available at all times to answer questions or to eradicate any uncertainties. All abovementioned questionnaires were completed while participants were seated in an area that was secluded under the supervision of researchers.
Video match recordingsA digital video camera (Sony HDR-PJ790VE handycam, Sony Corporation, Tokyo, Japan) with a high frame rate, good resolution, wide angle lens and an ability to deal with lower light levels of indoor sport facilities was stationed behind the court on a tripod stand to cover the entire court. Video footage was used to determine the time periods of each match so that researchers were able to set the correct duration for each of the HRV and HRR variables.
Statistical analysisThe Statistical Data Processing package (Statsoft Inc., 2015) was used to process data. Firstly, recovery indicators that obtained negative values were changed to positive values in order to ensure that all data showed the same trend i.e. all recovery indicator values showed ascending values as strength increased. This change is re-commended in order to increase the accuracy and effectiveness of canonical correlation analyses (Statsoft, 2015). Next the Box Cox transformation was applied to HRV variables to improve the normality of data. Thirdly, each variable’s descriptive statistics (minimum and maximum values, averages, and standard deviation) were calculated. In the next step cluster analyses (with distance determined by 1-Pearson correlation coefficient) of all HRV-, HRR-, recovery- and mood state related variables were performed to detect clusters of measures that are related to each other. Linkage distance for detection of different clusters was set at 0.2. The cluster analysis reduced variables were then entered into a Canonical Correlation Ana- lysis, which is a technique for analysing relationships between different sets of variables (Razavi et al., 2005). Results of several recovery indicators were correlated to pre-match, in-match, resting and post-match HRV as well as HRR values by doing separate analyses. Therefore, hydration status, muscle soreness, sleep quality and quantity as well as pre-match mood states were categorized under one category whereas pre-match, in-match, resting and post-match HRV as well as HRR results were categorised under a separate category. Finally, the level of significance was set at p ≤ 0.05.
The descriptive statistics of badminton players’ HR-, HRR- as well as HRV-related variables over different time periods are displayed in Table 2. From Table 2 it is evident that Mean R-R values declined from the pre- to the in-match period. Although Mean R-R recovered during the in-match rest and post-match periods, values did not recover back to pre-match values. Ln-SDNN, Ln-SD1 and Ln-SD2 also followed the same trend while the LF/HF ratio increased as the match progressed with the post-match period obtaining the highest values. HRR was higher for the post- compared to in-match rest periods. Average HR exhibited by all participants was 167 ± 14 bpm and the average maximum HR attained during matches, 193 ± 11 bpm. Table 3 presents descriptive statistics of recovery indicators that are related to competition participation. Table 3 shows that participants slept on average 6.64 ± 1.38 hours per night and indicated that their sleeping quality was quite high (3.57 ± 1.03). Urine shade scale results were moderate (2.76 ± 1.08) and muscle soreness tended to move to the higher side (1.74 ± 0.68). Regar-ding STEMS scores, vigour was the most prominent mood state among players with an average score of 11.08 ± 2.96. This result also contributed to a high average energy index scores (7.30 ± 4.64). In an attempt to first identify the recovery-related variables that relate to each other and to retain only relevant variables for canonical correlation analyses, a cluster analysis was executed. The recovery-related variables were reduced from 11 to the following 9 variables: Sleep duration, sleep quality, shade of urine, muscle soreness, STEMS tension, vigour, energy index, anger and confusion. These recovery indicators were used for further analyses.
Pre-matchIn the first canonical correlation analysis, the relationship between recovery indicators and the cluster analysis’ reduced HRV-related variables for the pre-match period was determined. A cluster analysis reduced the HRV-related variables from 14 to the following 8 variables for this period: Mean R-R, Ln-RMSSD, peak HF (Hz), Ln-HFnu power, peak VLF (Hz), VLF power (%), LF power (%) and Ln-LFnu/Ln-HFnu ratio. Results of this analysis are displayed in Table 4. A strong non-significant canonical correlation of 0.98 (p = 0.063) was found between the recovery indicators and pre-match HRV-related variables of participants. The canonical function, therefore, accounted for about 90.06% (r2 x 100) of the total variation between the two canonical variants. The variance extracted values were also calculated which indicates the average amount of variance extracted from the variables in the respective set by all canonical roots. Therefore, 100% of the variance was extracted from the right set of variables and 85.23% of the variance of the left set. Lastly, the total redundancy values showed that 32.53% of the variance in the nine recovery indicators could be accounted for given the eight pre-match HRV-related variables. Likewise, 51.12% of the variance in the pre-match HRV-related variables could be accounted for given the recovery indicators.
In-matchIn the second canonical correlation analysis, the relationship between recovery indicators and the cluster analysis’ reduced HRV-related variables for the in-match period was determined. The number of HRV-related variables was reduced from 14 to 8 by means of the cluster analysis, which included the following: mean R-R, Ln-RMSSD, VLF power (%), LF power (%), Ln-HFnu power, Ln-LFnu/Ln-HFnu ratio, peak VLF (Hz) and peak HF (Hz). Results are displayed in Table 5. A strong significant canonical correlation of 0.96 (p = 0.014) was found for the relationship between the above-mentioned sets of variables. The canonical function, therefore, accounted for about 91.58% (r2 x 100) of the total variation between the two canonical variants. The variance extracted values showed that 82.24% and 100% could be extracted for the left and right set respectively. Lastly, the total redundancy values showed that, on average, 44.96% of the variance in the nine recovery indicators could be accounted for by the eight in-match HRV-related variables. Likewise, 58.37% of the variance in the eight in-match HRV-related variables could be accounted for, given the nine recovery indicators.
In-match rest periodsIn the third canonical correlation analysis, the relationship between recovery indicators and the cluster analysis’ reduced HRV- and HRR-related variables for the in-match rest periods was determined. The cluster analysis reduced the number of HRV and HRR from 15 to the following 9 variables: HRR, Ln-RMSSD, peak VLF (Hz), peak LF (Hz), peak HF (Hz), LF power (%), VLF power (%), Ln-HFnu power and Ln-LFnu/Ln-HFnu ratio. The results are displayed in Table 6. The canonical correlation between the recovery indicators and in-match rest HRV-as well as HRR-related variables of the group of players revealed a non-significant canonical correlation of 0.69 (p = 0.258). The canonical function, therefore, accounted for about 47.89% (r2 x 100) of the total variation between the two canonical variants. The variance extracted values showed that 100% could be extracted for both sets. Lastly, the total redundancy values revealed that 26.91% of the variance in the nine recovery indicators could be accounted for by the nine in-match rest HRV-and HRR-related variables. Likewise, 47.27% of the variance in the nine in-match rest HRV- as well as HRR-related variables could be accounted for, given the nine recovery indicators.
Post-matchIn the fourth and final canonical correlation analysis, the relationship between recovery indicators and the cluster analysis’ reduced HRV- and HRR-related variables for the post-match period was determined. The number of HRV- and HRR-related variables was reduced from 15 to 9 by means of the cluster analysis, which included the following: HRR post-game, mean R-R, Ln-RMSSD, peak VLF (Hz), peak HF (Hz), Ln-LFnu/Ln-HFnu ratio, VLF power (%), LF power (%) and Ln-HFnu power. The results are displayed in Table 7. The canonical correlation between the recovery indicators and post-match HRV- as well as HRR-related variables of players revealed a strong non-significant canonical correlation of 0.98 (p = 0.085). The canonical function, therefore, accounted for about 96.43% (r2 x 100) of the total variation between the two canonical variants. The variance extracted values showed that 100% could be extracted for both sets. The total redundancy values revealed that 47.64% of the variance in the nine recovery indicators could be accounted for, given the nine post- match HRV- and HRR-related variables. Likewise, 52.71% of the variance in the nine post-match HRV- as well as HRR-related variables could be accounted for, given the nine recovery indicators.
The primary aim of this study was to determine if HRV and HRR are related to several subjective indicators of recovery status for different match periods in male, elite, African, singles badminton players. Canonical correlations for relationships between recovery indicators that are related to competition participation and HRV- as well as HRR-related variables for each time period, were as follows: Rc = 0.98 (p = 0.626) for the pre-match period; Rc = 0.96 (p = 0.014) for the in-match period; Rc = 0.69 (p = 0.258) for the in-match rest periods and Rc = 0.98 (p = 0.085) for the post-match period. Therefore, three time periods delivered strong non-significant canonical correlations of between 0.69 and 0.98 for relationships between above-mentioned variables whereas a significant canonical correlation of 0.98 was found for the in-match period. Consequently, canonical functions accounted for between 47.89% and 96.43% of the total variation between the two canonical roots. The novelty of this study lies in the fact that there are no available studies investigating the relationship of HRV and HRR with several subjective indicators of recovery status in male, elite, African, singles badminton players. Additionally, this is also the first study to investigate relationships between muscle soreness, hydration status, sleep quality and quantity, as well as pre-competition mood states and the ANS (through HRV and HRR) during different periods of badminton matches (pre-match, in-match and post-match). Although researchers expected recovery indicators to be significantly related to HRV- and HRR-related measures, only the in-match period delivered significant results. HRV and HRR are tools with which ANS status can be evaluated i.e. whether an athlete’s ANS is currently in a state of parasympathetic or sympathetic predominance (Plews et al., 2014). Several studies have recommended the use of psychometric and recovery related measures in conjunction with HRV and HRR to more effectively gauge the ANS in sport and exercise settings (Buchheit, 2014; Mclean et al., 2010). In this regard, Buchheit et al. (2013), who investigated the relationship between recovery indicators and HRV in Australian football players during a pre-season training camp, showed that a significant relationship (r = 0.53) existed between muscle soreness and HRV (ln-SD1). However, none of the other components of the questionnaire that determined recovery indicators (e.g., perceived fatigue, sleep quality, and overall stress level) obtained significant results (Buchheit et al., 2013). Similarly, a study on competitive long-distance runners who were monitored over a competition period of 12 weeks, found a significant overall relationship (r = 0.65) between HF components of HRV, and sleep quality and quantity (Leti and Bricout, 2013). On the other hand, fatigue (as determined through the state of fatigue and muscle soreness [SFMS] questionnaire) and mood states (as determined through the POMS questionnaire) were not significantly related to HRV (Leti and Bricout, 2013). We also did not find significant relationships between several recovery indicators and HRV-related measures in badminton players for most of the match periods. A possible reason for the lack of significance in relationships is the fact that the recovery indicators showed conflicting trends. For example, players reported below average sleeping hours (6.64 ± 1.38 hours per night), above-average levels of muscle soreness (1.74 ± 0.68 on a scale of 1 to 3) and scored higher on the “tension” (4.80 ± 3.50) mood state of the STEMS which are all indicators of psychological and/or physiological stress (Tavernier and Willoughby, 2014; Mateo et al. 2012; Spira et al., 2012; Grobbelaar et al., 2011; Blasquez and Ortis, 2009). We would expect higher tension and muscle soreness as well as less sleeping hours to have shifted players’ ANS towards sympathetic dominance as increased muscle soreness, inadequate sleeping hours and negative or stress related mood states can induce a state of sympathetic predominance (Blasquez and Ortis, 2009; Buchheit et al., 2013; Oliveira et al., 2011; Vaara et al., 2009). However, players were not dehydrated (2.76 ± 1.08 on a scale of 1 to 5) which suggests that some factors did not influence players’ ANS status (Buchheit et al., 2013; Lew et al., 2010; Oliveira et al., 2011; Trost et al., 2012). On the other hand, HRV-related variables showed values that are commonly associated with competition participation (Buchheit, 2014; Garrido-Esquivel et al., 2011). The time (Ln-SDNN and Ln-RMSSD) and non-linear (Ln-SD1 and Ln-SD2) HRV variables exhibited a decrease (compared to pre-match values) during matches and slight increases during in-match rest and post-match periods. Frequency domain HRV variables also showed normal trends as Ln-HFnu decreased as matches progressed along with the Ln-LFnu/Ln-HFnu ratio. Mentioned changes in HRV-related values over match duration would indicate that players experienced parasympathetic withdrawal together with sympathetic activation which were probably caused by an increase in metabolic demands as matches progressed (Buchheit, 2014; Reyes del Paso et al., 2013). However, most of the HRV and HRR parameters that were included in the Canonical Correlation Analysis for the in-match period are representative of parasympathetic activity (HRR rest, Ln-RMSSD, Ln-HFnu Power and an average Ln-LFnu/Ln-HFnu ratio of under 1) (Burr, 2007; Hottenrott et al., 2006). Therefore, the significant positive canonical correlation would imply that increases in the values of the recovery indicators would give rise to increases in the values of the parasympathetic activity-related HRV. High match loads combined with insufficient recovery will provoke higher sympathetic activity whereas sufficient recovery (as indicated by the higher recovery indicator scores), would lead to higher parasympathetic activity (Morales et al., 2014). This change in ANS functioning due to recovery will probably be the most pronounced during the match period as this is the period during which sympathovagal balance is challenged the most compared to other periods (pre-match, in-match rest and post-match). Despite the above-mentioned assumption, players exhibited below average in-match rest (12.90% ± 6.31) and post-match HRR values (14.30% ± 4.80), when categorised according to the average value of 38% that researchers propose for trained athletes (Javorka et al., 2002). Low HRR may be an indication that parasympathetic reactivation was delayed during rest periods (Boullosa et al. 2013; Daanen et al., 2012). This is surprising as parasympathetic reactivation is the accepted physiological response of the ANS after exercise or competition participation (Leti and Bricout, 2013; Oliveira et al., 2013). Differences between the stress levels of individual players during a competitive period may cause some players to shift towards sympathetic predominance due to the high pressure of match play and outcomes, which would explain why players in this study showed a delayed HRR response (D'Ascenzi et al., 2014; Garrido-Esquivel et al., 2011). This anomaly combined with the opposite trends in recovery related data most likely contributed to the lack of significant relationships for the majority of time periods. It is interesting to note that only the in-match time period delivered a significant canonical correlation coefficient for the two categories of variables. This is a promi-sing and unanticipated finding on the grounds that HRV measured during exercise is inherently complicated to analyse due to its exercise-intensity dependency (Buchheit, 2014). Every competitive badminton match will show a distinctive intensity level due to differences in player tactics, opponents’ playing level and over-all match characteristics, all factors that will directly influence players’ HRV (Laffaye et al., 2015; Mcnarry and Lewis, 2012). This finding is also in direct contrast to what others have found. For example, Pinna et al. (2007) observed that players’ mood, alertness and mental activity are minimised during exercise and as such will have less influence on a player’s over-all HRV profile. However statistically, the majority of HRV-related variables obtained much smaller standard deviation values for this time period compared to resting values, which would indicate that data distribution was narrower. A narrow data distribution would benefit the strength of the correlation coefficient and provide an explanation for the significant result. None the less, our results substantiate the significant influence that recovery-related variables have on HRV measures in a competitive badminton environment. As such, coaches and sport scientists should consider and correct for these recovery-related variables when measuring HRV during competition participation. Additionally, for 3 out of 4 periods, the canonical functions accounted for more than 90% of the total variation between the two canonical variants, which is consi-dered to be a very high value (Statsoft, 2015). However, for in-match rest periods, the canonical function accoun-ted for only 48% of the total variation between the two canonical variants, which is considered to be a moderately low value (Statsoft, 2015). Furthermore, average total redundancy scores, which is useful for assessing the practical significance of canonical roots (Statsoft, 2015), were much higher for the pre-, in- and post-match periods (between 32.53 and 58.37%) compared to the in-match rest periods (26.91% and 47.27%). Therefore, HRV-related measures seem to be more influenced by recovery indicators before, during and after matches that during in-match rest periods. These results are unexpected as two sets of HRV measurements were taken during rest periods (in- and post-match) after the completion of badminton-related actions and activities at the exact same time interval (60 sec post-match or set). The possible explanation for the discrepancy between results for last-mentioned periods may lie in the fact that parasympathetic activity was more suppressed during the in-match rest compared to the post-match periods. Proof for this contention is provided by the lower mean R-R values (Table 2) that were obtained during the in-match rest periods (364.07 ± 46.12) compared to the post-match periods (381.49 ± 52.15). Low variations in R-R intervals or a shorter time duration of R-R intervals is an indication of sympathetic predominance, which occurs when parasympathetic acti-vity is more suppressed (Dong, 2016; Aubert et al., 2003). On the other hand, high variations in R-R intervals or a longer time duration of R-R intervals is usually an indi-cation of parasympathetic predominance which occurs when parasympathetic activity is not suppressed) (Tarvainen et al., 2014). Consequently, players’ ANS may have been under more pressure during in-match rest periods due to among other things, the superseding sets and match play activities compared to the post-match period during which players could rest. A further analysis of canonical weights revealed that Ln-HFnu was the primary HRV-related variable and energy index as well as vigour STEMS mood states the primary recovery indicators to contribute to relationships during all match periods. This finding was not surprising due the fact that positive psychological mood states (such as vigour and energy index) are known to directly influence athletes’ ANS status (Shiota et al., 2011), which would explain last-mentioned results. In addition, Tulppo et al. (1998) found that HFnu is a reliable measure of HRV during training conditions due to the fact that HFnu values did not differ significantly between resting and exercise conditions in physically active adults during a maximal bicycle oxygen uptake test. What above mentioned findings suggest is that coaches and sport scientists should primarily correct for mood state related variables when interpreting HRV results. This would increase the validity and accuracy of HRV measures.
Practical applicationsThis study proved that recovery indicators do in fact influence short-term HRV and HRR values measured du-ring a real badminton competitive environment. Practitioners should therefore incorporate recovery indicators in their protocols when evaluating HRV and HRR in similar conditions. Failure to do so will most likely result in clouded and obscure results. Additionally, mood state-related variables such as energy index and vigour as measured by Likert scale-based questionnaires should be incorporated as recovery indicators as they have the biggest influence on HRV-related values during badminton competition participation.
The primary aim of this study was to determine if HRV and HRR are related to several subjective indicators of recovery status for different match periods in male, elite, African, singles badminton players. The novel finding of the study was that strong, non-significant relationships existed between HRV, HRR and several subjective indicators of recovery status for the majority of match periods. These results compelled researchers to reject the hypothesis that significant relationships exist between HRV, HRR and above-mentioned subjective indicators of recovery status for different match periods in badminton players. Only in-match HRV values were significantly influenced by recovery indicators, although very strong non-significant relationships existed for pre- and post-match time periods. On the other hand, the canonical correlation coefficient for rest periods during matches (service breaks) obtained a non-significant, low value. Furthermore, Ln-HFnu, the energy index and vigour STEMS mood states were identified as the primary variables that influenced relationships between the two categories of variables. Strong canonical correlation coefficients as well as rather high total redundancy values suggest that recovery indicators directly influence HRV and HRR values, especially during pre-, in- and post-match periods. Therefore, coaches and sport scientists should consider and correct for recovery indicators when employing HRV and HRR in their protocols. Secondly, the relationship between HRV and recovery indicators appears to be the most relevant during matches due to the fact that the strongest correlation was obtained for this period. On the other hand, HRV and HRR during in-match rest periods seem to be the least affected by recovery indicators. Therefore, coaches and sport scientists who wish to evaluate ANS status during this time period will be able to do so without correcting for recovery indicators. Thirdly, findings would suggest that mood state-related variables (i.e. energy index and vigour) influence the parasympathetic mediated HRV variables such as Ln-HFnu more than other recovery indicators. Mood state-related variables should therefore be considered when measuring HRV in sport and exercise settings. However, in view that this was an exploratory study, future researcher should investigate these relationships to confirm results. Furthermore, researchers should consider the following limitations when interpre-ting results: Players’ HRV and HRR values were only measured over short time periods. According to Plews et al. (2013) longitudinal monitoring of HRV is needed to better understand an athlete’s optimal HRV fingerprint. A true baseline measurement would also aid in the understanding of how the ANS reacts to competitive conditions. Lastly, future researchers should also measure recovery related indicators quantitatively in order to verify the qualitative results of questionnaires. Despite these recommendations, time and logistical constraints, especially during matches and competitions make it difficult to meet all recommendations.
|
AUTHOR BIOGRAPHY |
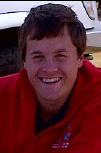 |
Christo A. Bisschoff |
Employment: PhD candidate in the Physical activity, Sport and Recreation Research Focus Area, Faculty of Health Sciences, North-West University, Potchefstroom Campus, Potchefstroom, South Africa |
Degree: MSc |
Research interests: Heart rate variability, exercise physio-logy and elite conditioning in rugby union |
E-mail: christo.bisschoff8@gmail.com |
|
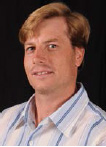 |
Ben Coetzee |
Employment: Associate Professor, Physical activity, Sport and Recreation Research Focus Area, Faculty of Health Sciences, North-West University, Potchefstroom Campus, Potchefstroom, South Africa |
Degree: PhD |
Research interests: Heart rate variability, exercise physiology, strength and conditioning |
E-mail: ben.coetzee @nwu.ac.za |
|
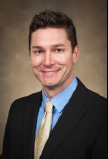 |
Michael R. Esco |
Employment: Associate Professor of Exercise Physiology, Department of Kinesiology, The University of Alabama, Tuscaloosa, AL, USA |
Degree: PhD |
Research interests: Heart rate variability, physiological adaptations to training, strength and conditioning, athletic monitoring |
E-mail: mresco@ua.edu |
|
|
|
REFERENCES |
 Aubert A.E., Seps B., Beckers F. (2003) Heart rate variability in athletes. Sports Medicine 33, 889-919.
|
 Blasquez J.C.C., Ortis L.C. (2009) Heart rate variability and pre-competitive anxiety in swimmers. Psicothema Revista Anual de Psicologia 21, 531-536.
|
 Borresen J., Lambert M.I. (2008) Autonomic control of the heart rate during and after exercise. Sports Medicine 38, 633-646.
|
 Boullosa D.A., Abreu L., Nakamura F.Y., Munoz V.E., Dominguez E., Leicht A.S. (2013) Cardiac autonomic adaptations in elite Spanish soccer players during preseason. International Journal of Sports Physiology and Performance 8, 400-409.
|
 Buchheit M (2014) Monitoring training status with HR measures: do all roads lead to Rome. Frontiers in Physiology 27, 73-91.
|
 Buchheit M., Papelier Y., Laursen P.B., Ahmaidi S. (2007) Non-invasive assessment of cardiac parasympathetic function: postexercise heart rate recovery or heart rate variability?. American Journal of Physiology Heart and Circulatory Physiology 293, H8-10.
|
 Buchheit M., Racinais S., Bilsborough J.C., Bourdon P.C., Voss S.C., Hocking J., Cordy J., Mendez-Villanueva A., Coutts A.J. (2013) Monitoring fitness, fatigue and running performance during a pre-season training camp in elite football players. Journal of Science and Medicine in Sport 16, 550-555.
|
 Burr R.L. (2007) Interpretation of normalized spectral heart rate variability indices in sleep research: a critical review. Sleep 30, 913-919.
|
 D'Ascenzi F., Alvino F., Natali B.M., Cameli M., Palmitesta P., Boschetti G., Bonifazi M., Mondillo S. (2014) Pre-competitive assessment of heart rate variability in elite female athletes during play-offs. Clinical Physiology and Functional Imaging 34, 230-236.
|
 Daanen H.A., Lamberts R.P., Kallen V.L., Jin A., Van Meeteren N.L. (2012) A systematic review on heart rate recovery to monitor changes in training status in athletes. Journal of Sports Physiology and Performance 7, 251-260.
|
 Dong J.G. (2016) The role of heart rate variability in sports physiology. Experimental and Therapeutic Medicine 11, 1531-1536.
|
 Esco M.R., Olson M.S., Williford H.N., Blessing D.L., Shannon D., Grandjean P. (2010) The relationship between resting heart rate variability and heart rate recovery. Clinical Autonomic Science 20, 33-38.
|
 Gamelin F.X., Berthoin S., Bosquet L. (2006) Validity of the Polar S810 heart rate monitor to measure R-R intervals at rest. Medicine and Science in Sport and Exercise 38, 887-893.
|
 Garrido-Esquivel A., Torres B., Corrales M.M., Garrido-Salazar M.A., Orellana J.N. (2011) Heart rate variability after three badminton matches. Are there gender differences. Archivos Medi-cina in Adeldeporte 28, 257-264.
|
 Garrido-Esquivel A., Torres B., Corrales M.M., Garrido-Salazar M.A., Orellana J.N. (2011) Heart rate variability after three badminton matches. Are there gender differences?. Archivos De Medicina Del Deporte 28, 257-264.
|
 Gastin P.B., Meyer D., Robinson D. (2013) Perceptions of wellness to monitor adaptive responses to training and competition in elite Australian football. The Journal of Strength and Conditioning Research 27, 2518-2526.
|
 Gocentas A., Landor A., Krisciunas A. (2011) Heart rate recovery changes during competition period in high-level basketball players. Education Physical Training Sport 80, 11-16.
|
 Grobbelaar H.W., Malan D.D.J., Steyn B.J.M., Ellis S.M. (2011) The relationship between burnout and mood state among student rugby union players. African Journal for Physical Health Education, Recreation and Dance 17, 647-664.
|
 Hottenrott K., Hoos O., Esperer H.D. (2006) Heart rate variability and physical exercise. Current status. Herz 31, 544-552.
|
 Javorka M., Zila I., Balharek T., Javorka K. (2002) Heart rate recovery after exercise: relations to heart rate variability and complexity. Brazilian Journal of Medicine and Biology Research 35, 991-1000.
|
 Kenttä G., Hassmén P., Raglin J.S. (2006) Mood states monitoring of training and recovery in elite kayakers. European Journal of Sport Science 6, 245-253.
|
 Laffaye G., Phomsoupha M., Dor F. (2015) Changes in the Game Characteristics of a Badminton Match: A Longitudinal Study through the Olympic Game Finals Analysis in Men’s Singles. Journal of Sports Science and Medicine 14, 584-590.
|
 Leti T., Bricout V.A. (2013) Interest of analyses of heart rate variability in the prevention of fatigue states in senior runners. Autonomic Neuroscience 173, 14-21.
|
 Lew C.H., Slater G., Nair G., Miller M. (2010) Relationship between changes in upon waking urinary indices of hydration status and body mass in adolescent Singaporean athletes. International Journal of Sport Nutrition 20, 330-335.
|
 Mateo M., Blasco-Lafarga C., Martínez-Navarro I., Guzmán J.F., Zabala M. (2012) Heart rate variability and pre-competitive anxiety in BMX discipline. European Journal of Applied Phy-siology 112, 113-123.
|
 McLean B.D., Coutts A.J., Kelly V., McGuigan M.R., Cormack S.J. (2010) Neuromuscular, endocrine and perceptual fatigue responses during different length between-match micro cycles in professional rugby league players. International Journal of Sports Physiology and Performance 5, 367-383.
|
 McNair D.M., Lorr M., Droppleman L.F. (1971) Manual for the profile of mood states.. San Diego, CA. Educational and industrial testing services.
|
 McNarry M.A., Lewis M.J. (2012) Heart rate variability reproducibility during exercise. Physiological Measurement 33, 1123-1133.
|
 Molina G., Fontana K., Porto L., Junqueira L. (2016) Clinical Autonomic Research. Post-exercise heart-rate recovery correlates to resting heart-rate variability in healthy men. Electronically published.
|
 Morales J., Alamo J. M., García-Massó X., Buscà B., López J. L., Serra-Añó P., González L. (2014) Use of heart rate variability in monitoring stress and recovery in judo athletes. Journal of Strength and Conditioning Research 28, 1896-1905.
|
 Nakamura F.Y., Soares-Caldeira L.F., Laursen P.B., Polito M.D., Leme L.C., Buchheit M. (2009) Cardiac autonomic responses to repeated shuttle sprints. International Journal of Sports Medicine 30, 808-813.
|
 Nunan D., Jakovljevic D., Donovan G., Singleton L., Sandercock G., Brodie D. (2010) Resting autonomic modulations and the heart rate response to exercise. Clinical Autonomic Research 20, 213-221.
|
 Oliveira T.P., Ferreira R.B., Mattos R.A., Silva J.P., Lima J.R.P. (2011) Influence of water intake on post-exercise heart rate variability recovery. Journal of Exercise Physiology Online 14, 97-105.
|
 Perandini L.A., Chimin P., Okuno N.M., Perrout de Lima J.R., Buchheit M., Nakamura F.Y. (2009) Parasympathetic withdrawal during the 30-15 intermittent fitness test correlates with its’ maximal running speed in male handball players. Journal of Exercise Physiology Online 12, 29-38.
|
 Pinna G.D., Maestri R., Torunski A., Danilowics-Szymanowics L., Szwoch M., La Rovere M., Raczak G. (2007) Heart rate variability measures: a fresh look at reliability. Clinical Science 113, 131-140.
|
 Plews D.J., Laursen P.B., Kilding A.E., Buchheit M. (2012) Heart rate variability in elite triathletes, is variation in variability the key to effective training? A case comparison. European Journal of Applied Physiology 112, 3729-3741.
|
 Plews D.J., Laursen P.B., Le Meur Y., Hausswirth C., Kilding A.E., Buchheit M. (2014) Monitoring training with heart rate variability: how much compliance is needed for a valid assessment. International Journal of Sports Physiology and Performance 9, 783-790.
|
 Plews D.J., Laursen P.B., Stanley J., Kilding A.E., Buchheit M. (2013) Training adaptation and heart rate variability in elite endurance athletes: opening the door to effective monitoring. Sports Medicine 43, 773-791.
|
 Razavi A.R., Gill H., Stal O., Sundquist M., Thorstenson S., Ahlfeldt H., Shahsavar N., the South-East Swedish Breast Cancer Study Group (2005) Exploring cancer register data to find risk factors for recurrence of breast cancer - application of Canonical Correlation Analysis. BMC Medical Informatics and Decision Making 5, 1-7.
|
 Reyes del Paso G.A., Langewitz W., Mulder L.J., Roon A., Duschek S. (2013) The utility of low frequency heart rate variability as an index of sympathetic cardiac tone: a review with emphasis on a reanalysis of previous studies. Psychophysiology 50, 477-487.
|
 Roy S., McCrory J. (2015) Validation of maximal heart rate prediction equations based on sex and physical activity status. International Journal of Exercise Science 8, 318-330.
|
 Saw A.E., Main L.C., Gastin P.B. (2015) Monitoring the athlete training response: subjective self-reported measures trump commonly used objective measures: a systematic review. Bri-tish Journal of Sports Medicine 1, 1-13.
|
 Shiota M.N., Neufeld S.L., Yeung W.H., Moser S.E., Perea E.F. (2011) Feeling good: autonomic nervous system responding in five positive emotions. Emotion 11, 1368-1378.
|
 Spira A.P., Beaudreau S.A., Stone K.L., Kezirian E.J., Lui L., Redline S., Acoli-Israel S., Ensrud K., Stewart A. (2012) Reliability and validity of the Pittsburgh sleep quality index and the epworth sleepiness scale in older men. Journal of Geronto-logy: Medical Sciences 67, 433-439.
|
 Stanley J., Peake J.M., Buchheit M. (2013) Consecutive days of cold water immersion: effects on cycling performance and heart rate variability. European Journal of Applied Physiology 113, 371-384.
|
 Statsoft Inc. (2015) STATISTICA (Data analysis software system), version 12.5.. http://www.statsoft.com.
|
 Stewart A., Marfell-Jones M., Olds T., De Ridder H. (2011) International standards for anthropometric assessment. Lower Hutt, New Zealand. ISAK.
|
 Swann C., Moran A., Piggott D. (2015) Defining elite athletes: Issues in the study of expert performance in sport psychology. Psychology of Sport and Exercise 16, 3-14.
|
 Tarvainen M.P., Niskanen J.P., Lipponen J.A., Ranta-Aho P.O., Karjalainen P.A. (2014) Kubios HRV-heart rate variability analysis software. Computer methods and Programs in Biome-dicine 113, 210-220.
|
 Tavernier R., Willoughby T. (2014) Bidirectional associations between sleep (quality and duration) and psychosocial functioning across the years. Developmental Psychology 50, 674-783.
|
 Terry P.C., Lane L.M., Fogarty G.J. (2003) Construct the validity of the Profile of Mood States – adolescents for use with adults. Psychology of Sport and Exercise 4, 125-139.
|
 Trost Z., France C.R., Sullivan M.J., Thomas J.S. (2012) Pain-related fear predicts reduced spinal motion following experimental back injury. Pain 153, 1015-1021.
|
 Tulppo M.P., Mäkikallio T.H., Seppänen T., Laukkanen R.T., Huikuri H.V. (1998) Vagal modulation of heart rate during exercise: effects of age and physical fitness. American Journal of Physiology-Heart and Circulatory Physiology 274, 424-429.
|
 Vaara J., Kyrolainen H., Koivu M., Tulppo M., Finni T. (2009) The effect of 60-h sleep deprivation on cardiovascular regulation and body temperature. European Journal of Applied Physiology 105, 439-444.
|
|
|
|
|
|
|