|
|
|
ABSTRACT |
A systems modelling approach can be used to describe and optimise responses to training stimuli within individuals. However, the requirement for regular maximal performance testing has precluded the widespread implementation of such modelling approaches in team-sport settings. Heart rate variability (HRV) can be used to measure an athlete’s adaptation to training load, without disrupting the training process. As such, the aim of the current study was to assess whether chronic HRV responses, as a representative marker of training adaptation, could be predicted from the training loads undertaken by elite Rugby Sevens players. Eight international male players were followed prospectively throughout an eight-week pre-season period, with HRV and training loads (session-RPE [sRPE] and high-speed distance [HSD]) recorded daily. The Banister model was used to estimate vagally-mediated chronic HRV responses to training loads over the first four weeks (tuning dataset); these estimates were then used to predict chronic HRV responses in the subsequent four-week period (validation dataset). Across the tuning dataset, high correlations were observed between modelled and recorded HRV for both sRPE (r = 0.66 ± 0.32) and HSD measures (r = 0.69 ± 0.12). Across the sRPE validation dataset, seven of the eight athletes met the criterion for validity (typical error <3% and Pearson r >0.30), compared to one athlete in the HSD validation dataset. The sRPE validation data produced likely lower mean bias values, and most likely higher Pearson correlations, compared to the HSD validation dataset. These data suggest that a systems theory approach can be used to accurately model chronic HRV responses to internal training loads within elite Rugby Sevens players, which may be useful for optimising the training process on an individual basis. |
Key words:
Cardiac parasympathetic function, monitoring, training load
|
Key
Points
- A systems theory approach can be used to describe the variation in chronic HRV responses to training within elite Rugby Sevens players.
- For the majority of athletes, model parameters can be used to accurately predict future responses to training stimuli.
- Responses that diverge from the predicted values may serve as a useful flag for the investigation of changes in lifestyle factors.
- Internal training load measures (sRPE) markedly outperformed external load measures (HSD) in predicting future HRV responses to training stimuli.
|
Several research groups have applied systems theory approaches to quantify and describe responses to physical training (Busso and Thomas, 2006; Morton, 1997; Mujika et al., 1996). The Banister impulse-response model estimates performance at a given time to be the difference between the ‘fitness’ and ‘fatigue’ effects of prior training loads (Banister et al., 1975). The five adjustable parameters within the model (initial performance level; two time constants that describe fitness and fatigue decay rates; and two gain parameters that describe how daily training impulses determine the amplitude in fitness and fatigue effects) are calibrated against measured performance data to provide individualised training response information (Banister et al., 1975). Based on these relatively simple assumptions, the Banister impulse-response model can explain a substantial proportion (over 90% in some cases) of the variance in performance data (Busso, 2003; Morton, 1997; Wood et al., 2005). However, a major limitation of the Banister model and its extensions is the requirement for frequent maximal performance tests to accurately determine model parameters (Jobson et al., 2009). The use of regular maximal performance testing is especially difficult in team-sport settings with weekly competitive fixtures, given their potential to cause additional fatigue (Nédélec et al., 2013). To date, the need for regular performance testing has limited the broader use of the Banister model in team-sports. Heart rate variability (HRV) is a popular tool for monitoring wellness and training adaptation in athletes (Bellenger et al., 2016). In particular, the parasympathetic activity of the autonomic nervous system, typically represented by the square root of the mean sum of the squared differences between R–R intervals (rMSSD) component of HRV, has been shown to correlate well with variations in performance within both cross-sectional (Kenney, 1985), and longitudinal studies (Chalencon et al., 2015) across multiple sports. In addition, HRV measures are associated with overuse injury risk (Williams et al., 2017) and, more broadly, markers of global health (Adamson et al., 2004; Kiviniemi et al., 2007). As such, rMSSD may be an appropriate representative parameter to describe an athlete’s stress-recovery status (Chalencon et al., 2012), especially given the ease and non-intrusive nature of its collection. Indeed, the emergence of smartphone applications and technologies has dramatically increased the accessibility of HRV measurement, such that it can now be recorded accurately using only a smartphone device (Plews et al., 2017). Recent work in competitive swimmers has demonstrated that HRV measures may be used as a viable substitute for performance measurements for the mathematical modelling of training effects (Chalencon et al., 2012), and could therefore be used to optimally plan and monitor training strategies in an individualised manner (Chalencon et al., 2015). However, this is yet to be applied and evaluated in a team sport context where, as stated previously, regularly monitoring changes in performance is inherently more complex than in individual, endurance-based sports. Rugby Sevens is a format of Rugby Union that has grown in popularity in recent years, and is now included in the Summer Olympic Games. The contact and collision events that are inherent to Rugby Sevens, alongside the high physiological demands (Higham et al., 2014), means that the risk of injury associated with the sport is relatively high (Fuller et al., 2010). In particular, the injury incidence rate associated with elite Rugby Sevens training is substantially higher than the 15-a-side game (West et al., 2017), which is likely a result of the high training loads that are necessary to meet the physiological demands of competition. Therefore, the careful monitoring and management of player workloads on an individual basis is of critical importance, in order to protect players from the negative consequences of training whilst increasing their performance capacity and resilience (Gabbett, 2016). Moreover, a consideration of the most appropriate load measures (e.g., internal versus external) for this setting is also required. Accordingly, the aim of the current study was to assess whether chronic HRV responses, as a representative marker of training adaptation, could be predicted from the training loads undertaken by elite Rugby Sevens players. In addition, we sought to compare the effectiveness of internal (session rating of perceived exertion [sRPE]) versus external (total high speed running distance [HSD]) load measures for this purpose.
Study designEight male international Rugby Sevens players (mean ± SD; age: 27 ± 4 y, height: 1.86 ± 0.07 m, body mass: 93.2 ± 8.6 kg) were followed prospectively throughout an eight-week pre-season period that was undertaken in preparation for the 2016-17 World Rugby Sevens Series. The priority during this phase was to develop central adaptations through the use of extensive intervals, with a linear increase in intensity. The average weekly sRPE and HSD loads across this period were 2947 ± 941 AU and 3389 ± 892 m, respectively. This eight week pre-season period was chosen as each parameter in the model was likely to be emphasised across this preparation phase (Clarke and Skiba, 2013), and periods of 60-90 days are recommended for the mathematical modelling of training and performance, after which parameters should be reset (Banister, 1991). The study was conducted in accordance with the principles of the Declaration of Helsinki (World Medical Association, 2013) and a local university research ethics committee provided ethical approval.
Measures
Heart rate variability
Athletes were instructed to perform a 90 second HRV measurement each morning upon waking whilst breathing spontaneously in a seated position (Esco and Flatt, 2014). A Polar H7 Bluetooth heart rate strap (Polar Electro, Kempele, Finland) paired with a freely available smartphone application (Elite HRV, Ashville, North Carolina, USA) were used for daily HRV acquisition. The rMSSD was the HRV measure used for analysis, as this has been demonstrated to have greater reliability than other spectral indices (Al Haddad et al., 2011). The rMSSD data were log-transformed (Ln) to reduce non-uniformity of error (Plews et al., 2012). The 42-day exponentially-weighted average of this variable (Ln rMSSD42-exp) was then calculated and used in further analyses, as a representative parameter of chronic training adaptation (Chalencon et al., 2015). The Ln rMSSD42-exp calculation was initiated with the mean Ln rMSSD value observed across the first seven days of the monitoring period. The validity of the Elite HRV application for computing Ln rMSSD was established by comparing simultaneous 60 s recordings of the same tools used in this study (i.e., Polar H7 Bluetooth heart rate strap and application) with an electrocardiograph (Biopac MP100, Goletta, California, USA) among 10 collegiate athletes. Procedures and comparison methods from a previous study were replicated (Esco et al., 2017). Measures of Ln rMSSD were acquired in the supine, seated and standing position for each individual. Differences between supine (Elite HRV = 3.70 ± 0.43 ms, ECG = 3.70 ± 0.43 ms) seated (Elite HRV = 3.44 ± 0.62 ms, ECG = 3.43 ± 0.59 ms) and standing (Elite HRV = 2.84 ± 0.52 ms, ECG = 2.85 ± 0.52 ms) measures were not significant (p = 0.80, 0.52 and 0.49, respectively) and the standardized differences were considered trivial (≤ 0.03 for each). The correlations between the application and ECG were near perfect (r = 0.99, p < 0.05 for each position). Additionally, upper and lower limits of agreement were tight (upper and lower limits = 0.03 ms to -0.03 ms for supine, 0.08 ms to -0.10 ms for seated and 0.13 ms to -0.10 ms for standing). These data demonstrate that the validity of the Elite HRV application for computing Ln rMSSD is consistent across supine, standing, and seated positions. Seated measurements were used within the current study to ensure a consistent approach throughout the study period.
Training loadInternal training loads were recorded for all sessions using the sRPE method (Foster, 1998). This approach has been shown to be a valid method for estimating exercise intensity across multiple training modalities (Herman et al., 2006). Player ratings of perceived exertion were recorded 30 min after completing a given session, and were then multiplied by the session duration (mins) to provide a sRPE value in arbitrary units. External training load was represented by the total high-speed distance (distance covered at speeds greater than 5 m/s [HSD]) undertaken during pitch-based sessions, recorded using global positioning system (GPS) devices (STATports® Viper Pod, 10 Hz single constellation). In Rugby Union, 5 m/s is the most commonly used threshold that corresponds to high-speed running (Clarke et al., 2015). The HSD measure was chosen to reflect the high-intensity nature of the sport’s demands (Suarez-Arrones et al., 2012), which is considered an important quality for performance in Rugby Sevens (Higham et al., 2012).
Data analysisThe mathematical relationship between training loads (system input) and Ln rMSSD42-exp (system output) was modelled for each athlete via the two-component impulse-response model (Banister et al., 1975). The model is characterized by two gain terms (k1 and k2), two time constants (𝜏1 and 𝜏2), and an initial performance level (p):
 |
The model parameters were determined by minimizing the Residual Sum of Squares (RSS) between estimated and measured Ln rMSSD42-exp within a customised spreadsheet (Microsoft Excel, Microsoft, Redmond, USA) (Clarke and Skiba, 2013). Model parameters were established using data collected across the first four-week training block (tuning dataset). As per Chalencon et al. (2015), the term ‘tuning dataset’ was used in this study over the more customary expression ‘training dataset’ to avoid ambiguity when discussing physical training. The estimates obtained from the tuning dataset were then used to predict Ln rMSSD42-exp responses across the subsequent four-week training block (validation dataset). The accuracy of models for predicting chronic HRV responses was assessed using an Excel spreadsheet (Hopkins, 2015) designed to calculate the mean bias, typical error of the estimate (in raw units, and expressed as a coefficient of variation [CV, %]), and Pearson correlation coefficient. The magnitude of correlation was defined as trivial (<.10), low (.10-.29), moderate (.30-.49), high (.50-.69), very high (.70-.89), or nearly perfect (.90-.99) (Hopkins, 2015). A CV of less than 3% and Pearson correlation >0.30 (moderate) was set as the criterion for validity, based on the established smallest worthwhile change for Ln rMSSD (Buchheit, 2014). Differences in bias and precision between the internal training load model (sRPE) and external training load model (HSD) were compared using standardised differences, and were interpreted as: <0.20, trivial; 0.20-0.59, small; 0.60-1.19, moderate; 1.20-1.99, large; >2.00, very large (Hopkins, 2010). Magnitude-based inferences were used to provide an interpretation of the real-world relevance of the outcomes. A value equivalent to a standardised difference in means of 0.20 was set as the smallest worthwhile effect threshold (Hopkins, 2010). Effects were classified as unclear if the percentage likelihood that the true effect crossed both positive and negative smallest worthwhile effect thresholds were both greater than 5%. Otherwise, the effect was deemed clear, and was qualified with a probabilistic term using the following scale: <0.5%, most unlikely; 0.5-5%, very unlikely; 5-25%, unlikely; 25-75%, possible; 75-95%, likely; 95-99.5%, very likely; >99.5%, most likely (Hopkins, 2010).
Estimates of model parametersThe mean ± SD values of the gain (k1 and k2) and time decay constants (𝜏1 and 𝜏2) for the sRPE tuning dataset were 0.0000767 ± 0.0000767 AU, 0.0000893 ± 0.0000716 AU, 20 ± 14 d, and 11 ± 7 d, respectively. For the HSD tuning set, the corresponding values were 0.000278 ± 0.000271 AU, 0.000276 ± 0.000263 AU, 32 ± 38 d, and 35 ± 43 d, respectively.
Model fit using tuning datasetsFor the sRPE tuning dataset, the Banister model produced high correlations between modelled and actual Ln rMSSD42-exp (r = 0.66 ± 0.32), with individual r values ranging from -0.08 to 0.96. The HSD tuning dataset produced a similar model fit (r = 0.69 ± 0.12), with r values ranging from 0.57 to 0.92. Figure 1 shows the best-fitting individual model (r = 0.96 for sRPE tuning dataset) for one athlete.
Accuracy of model predictions using validation datasetThe accuracy of the model parameters (estimated from the tuning dataset) in predicting Ln rMSSD42-exp responses across the validation period are displayed in Table 1. The CV was less than 3% for all eight athletes across the sRPE validation dataset, and seven of the eight athletes across the HSD validation dataset. Seven of the eight athletes had at least moderate positive relationships between their predicted and recorded chronic HRV responses across the sRPE validation dataset (range: -0.24 to 0.78), compared to one athlete within the HSD validation dataset (range: -0.87 to 0.33). Overall, seven of the eight athletes met the criterion for validity (CV <3% and Pearson r >0.30) for the sRPE validation data, compared to one athlete in the HSD validation dataset.
Comparison of prediction accuracy between load measuresThe sRPE validation data produced likely lower mean bias values, and most likely higher Pearson correlations, compared to the HSD validation dataset (Table 1). The sRPE models also produced lower typical errors compared to the HSD models, but these differences were unclear.
The primary purpose of this study was to assess whether chronic Ln rMSSD responses, as a representative marker of training adaptation, could be predicted from the training loads undertaken by elite Rugby Sevens players. Across the tuning dataset, high correlations were observed between modelled and measured HRV data. When model parameters estimated from the tuning dataset were used to predict future responses to training loads, seven of the eight athletes met the criterion for validity (CV <3% and Pearson r >0.30) for the sRPE data, compared to one athlete in the HSD validation dataset. The sRPE validation data produced likely lower mean bias values, and most likely higher Pearson correlations, compared to the HSD validation dataset. In the present study, the goodness-of-fit between modelled and measured chronic HRV responses across the tuning period (r = 0.66 and 0.69 for sRPE and HSD model, respectively) was lower than that obtained by Chalencon et al. (2015) in competitive swimmers (r = 0.93). Chalencon et al.’s (2015) use of the high-frequency component of HRV, as opposed to Ln rMSSD used in the current study, may account for the improved relationships, although Ln rMSSD has been proposed as the “most reliable and practically applicable measure for day-to-day monitoring” (Plews et al., 2013). In addition, Chalencon et al. (2015) used weekly measures of nocturnal HRV, as opposed to the daily measurements used in the present study. Rolling averages of Ln rMSSD data may represent a more meaningful assessment of any change in cardiac autonomic nervous system balance, compared with a single day value (Plews et al., 2012). A minimum of three valid data points per week are required when calculating rolling averages (Plews et al., 2014), which supports the use of daily measurements in the current study. Perhaps more importantly, there were considerable differences between the nature of the athletes used in these studies; swimming is an endurance-based sport in which athletes spend the majority of their training time at speeds below the blood lactate accumulation threshold (Mujika et al., 1995). Conversely, Rugby Sevens training is more varied with respect to both the intensity and modalities of training used (Higham et al., 2016), and so chronic HRV responses are likely to be less predictable in comparison to sports with less varied training stimuli. The model predictions produced using the validation dataset resulted in typical errors that were <3%, and Pearson correlations that were moderate and small for the sRPE and HSD validation datasets, respectively. The range of r values across both validation datasets (sRPE: -0.24 to 0.78; HSD: -0.87 to 0.33) implies large inter-individual differences in the utility of the parameter estimates obtained from the tuning dataset for predicting subsequent chronic HRV responses. For those athletes with at least moderate positive relationships and acceptably small (<3%) typical errors (n = 7 in the sRPE validation, and n = 1 in the HSD validation), the tuning dataset could be used to predict future chronic HRV responses to training loads with satisfactory accuracy, and thus be used to optimise their training on an individualised basis. For instance, the parameters obtained from the Banister model could be used to simulate the effects of different periodization schemes (Clarke and Skiba, 2013), to objectively plan training progressions for athletes rehabilitating from injury (Clarke and Skiba, 2013), or to individualise the ‘fitness’ and ‘fatigue’ time-decay constants within acute:chronic workload calculations (Carey et al., 2017). In addition, the Banister model could be used to produce individual influence curves that may inform the optimal taper strategy for each athlete leading into a Sevens tournament (Fitz-Clarke et al., 1991). Such influence curves can be created using freely available spreadsheets (Clarke and Skiba, 2013). However, the optimal HRV response to training overload and pre-competition tapers in elite athletes is yet to be fully understood (Plews et al., 2013). To date, the need for regular maximal performance testing has limited the many potential uses of the Banister model in team sports, but the use of HRV data as a surrogate measure of training adaptation may facilitate the practical application of the Banister model in these settings. Athletes for whom the predictive capacity across the validation period was low, may have experienced changes to their life stressors since the tuning data period that influenced their subsequent chronic HRV responses to training. HRV is known to be influenced by a wide range of factors, including physiological/pathological, neuropsychological, non-modifiable, lifestyle and environmental factors (Fatisson et al., 2016). Thus, chronic HRV responses that diverge from the predicted response to training stimuli compared to a baseline period may serve as a useful (and objective) ‘flag’ for the investigation of life stressors and lifestyle factors in that athlete (Gabbett et al., 2017). However, this concept requires further evaluation via the inclusion of ‘life stress/wellbeing’ measures in future studies. In the present study, sRPE data produced more accurate predictions of future HRV responses when compared to HSD data obtained from GPS devices, with likely lower levels of bias and most likely higher Pearson correlations observed between predicted and measured responses. As stated above, HRV responses may be influenced by a range of lifestyle and/or environmental factors (e.g., sleep quality) (Burton et al., 2010), which can also influence the sRPE internal load measure produced in response to a given external load (Impellizzeri et al., 2004). In contrast, external load measures will not be influenced by such factors, and are instead primarily determined by the workloads prescribed by coaching staff (Impellizzeri et al., 2005). In addition, the sRPE method enables the capture of loads undertaken across all training modalities (e.g., gym or pool-based sessions), whereas HSD could only be recorded for pitch-based sessions. As such, it is perhaps unsurprising that the sRPE measure outperformed the external load measure in predicting HRV responses to training impulses. These findings add to existing literature regarding the importance of monitoring and controlling athletes’ internal training loads, to ensure they are receiving an appropriate training stimulus (Impellizzeri et al., 2004). That being said, workloads are more easily prescribed via external load measures (e.g., by setting a target HSD for a given day), and so external load measures remain important for planning training programmes in this setting (Gabbett et al., 2017). A limitation of the current study is the lack of a true ‘performance’ measure, against which changes in HRV could be validated as a marker of training adaptation. Whilst there is a wealth of evidence to support the fact that the parasympathetic activity of the autonomic nervous system is a good indicator of an athlete’s adaptation to training loads (Adamson et al., 2004; Chalencon et al., 2015; Gisselman et al., 2016; Williams et al., 2017), this remains to be shown in Rugby Sevens athletes. Although it is difficult to define a single performance indicator for Rugby Sevens, as performance is dependent on numerous physical, tactical, psychological, and environmental factors (Higham et al., 2012), the ‘critical velocity’ model may provide a useful framework against which the HRV-performance relationship could be validated in future studies (Jones and Vanhatalo, 2017). The ‘critical velocity’ threshold represents a running velocity that can (theoretically) be maintained indefinitely, whilst a W' constant represents the finite work capacity available to an athlete at velocities greater than their critical velocity threshold (Jones and Vanhatalo, 2017). These parameters can be estimated from a single three minute all-out exercise test (Burnley et al., 2006). Given the significance of high-speed running ability to performance in Rugby Sevens (Higham et al., 2012), these constants are likely to be of substantial importance to overall performance in this setting. Moreover, the widespread use of GPS units in elite Rugby Sevens could theoretically enable the dynamic modelling of W' utilisation during training and matches (Jones and Vanhatalo, 2017).
These data demonstrate that a systems theory approach can be used to describe the variation in chronic HRV responses to training within elite Rugby Sevens players, and thus may be used to optimise training responses in this setting. For the majority of athletes in the sRPE validation dataset, the modelling of training effects also allowed for the accurate prediction of future responses to training stimuli. Responses that diverged from the ‘tuning dataset’ predictions may serve as a useful flag for the investigation of life stressors. The sRPE training load measure provided more accurate predictions of future HRV responses compared to an external load measure (HSD). The mathematical modelling of HRV responses to training loads may enable practitioners to more accurately assess and optimise the training process.
ACKNOWLEDGEMENTS |
The authors would like to acknowledge with considerable gratitude all those who volunteered to take part in this study. All authors contributed to data collection and manuscript preparation. No funding to declare. The authors have no conflicts of interest to declare. All experiments comply with the current laws of the country. |
|
AUTHOR BIOGRAPHY |
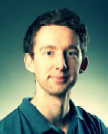 |
Sean Williams |
Employment: Lecturer, Department for Health, University of Bath, Bath, United Kingdom. |
Degree: PhD |
Research interests: Injury surveillance, injury prevention, training load monitoring, applied statistics. |
E-mail: S.Williams@bath.ac.uk |
|
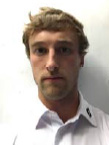 |
Stephen West |
Employment: Ph.D. student, Department for Health, University of Bath, Bath, United Kingdom |
Degree: MSc |
Research interests: Injury surveillance, injury prevention, training load monitoring, applied statistics. |
E-mail: S.West@bath.ac.uk |
|
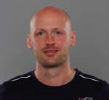 |
Dan Howells |
Employment: Strength and conditioning coach for England Sevens, Rugby Football Union, London, UK. |
Degree: BSc |
Research interests: Strength and conditioning, injury prevention, training load monitoring. |
E-mail: DanHowells@RFU.com |
|
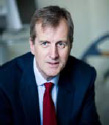 |
Simon P.T. Kemp |
Employment: Medical Services Director, Rugby Football Union, London, UK. |
Degree: MA, MB, MRCGP, MSc(SEM), FFSEM |
Research interests: Sports medicine, injury prevention, training load monitoring. |
E-mail: SimonKemp@RFU.com |
|
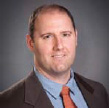 |
Andrew A. Flatt |
Employment: Assistant Professor & Graduate Program Director, Department of Health Science & Kinesiology, Georgia Southern University, Savannah, USA. |
Degree: PhD |
Research interests: Heart rate variability, Sports injuries, training load monitoring. |
E-mail: aflatt@georgiasouthern.edu |
|
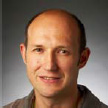 |
Keith Stokes |
Employment: Professor, Department for Health, University of Bath, Bath, United Kingdom, |
Degree: PhD |
Research interests: Injury surveillance, injury prevention, training load monitoring, applied physiology. |
E-mail: K.Stokes@bath.ac.uk |
|
|
|
REFERENCES |
 Adamson P.B., Smith A.L., Abraham W.T., Kleckner K.J., Stadler R.W., Shih A., Rhodes M.M. (2004) Continuous autonomic assessment in patients with symptomatic heart failure: prognostic value of heart rate variability measured by an implanted cardiac resynchronization device. Circulation 110, 2389-2394.
|
 Al Haddad H., Laursen P., Chollet D., Ahmaidi S., Buchheit M. (2011) Reliability of resting and postexercise heart rate measures. International Journal of Sports Medicine 32, 598-605.
|
 Banister E (1991) Modeling elite athletic performance. Physiological testing of elite athletes , 403-424.
|
 Banister E., Calvert T., Savage M., Bach T. (1975) A systems model of training for athletic performance. Australian Journal of Sports Medicine 7, 57-61.
|
 Bellenger C.R., Fuller J.T., Thomson R.L., Davison K., Robertson E.Y., Buckley J.D. (2016) Monitoring athletic training status through autonomic heart rate regulation: a systematic review and meta-analysis. Sports Medicine 46, 1461-1486.
|
 Buchheit M (2014) Monitoring training status with HR measures: do all roads lead to Rome?. Frontiers in physiology 5, 1-19.
|
 Burnley M., Doust J.H., Vanhatalo A. (2006) A 3-min all-out test to determine peak oxygen uptake and the maximal steady state. Medicine and Science in Sports and Exercise 38, 1995-2003.
|
 Burton A., Rahman K., Kadota Y., Lloyd A., Vollmer-Conna U. (2010) Reduced heart rate variability predicts poor sleep quality in a case–control study of chronic fatigue syndrome. Experimental Brain Research 204, 71-78.
|
 Busso T (2003) Variable dose-response relationship between exercise training and performance. Medicine and Science in Sports and Exercise 35, 1188-1195.
|
 Busso T., Thomas L. (2006) Using mathematical modeling in training planning. International Journal of Sports Physiology and Performance 1, 400-405.
|
 Carey D.L., Blanch P., Ong K.-L., Crossley K.M., Crow J., Morris M.E. (2017) Training loads and injury risk in Australian football—differing acute: chronic workload ratios influence match injury risk. British Journal of Sports Medicine 51, 1215-1220.
|
 Chalencon S., Busso T., Lacour J.-R., Garet M., Pichot V., Connes P., Gabel C.P., Roche F., Barthélémy J.C. (2012) A model for the training effects in swimming demonstrates a strong relationship between parasympathetic activity, performance and index of fatigue. PLoS ONE 7, e52636.
|
 Chalencon S., Pichot V., Roche F., Lacour J.-R., Garet M., Connes P., Barthélémy J.C., Busso T. (2015) Modeling of performance and ANS activity for predicting future responses to training. European Journal of Applied Physiology 115, 589-596.
|
 Clarke A.C., Anson J., Pyne D. (2015) Physiologically based GPS speed zones for evaluating running demands in Women’s Rugby Sevens. Journal of Sports Sciences 33, 1101-1108.
|
 Clarke D.C., Skiba P.F. (2013) Rationale and resources for teaching the mathematical modeling of athletic training and performance. Advances in physiology education 37, 134-152.
|
 Esco M.R., Flatt A.A. (2014) Ultra-short-term heart rate variability indexes at rest and post-exercise in athletes: evaluating the agreement with accepted recommendations. Journal of Sports Science & Medicine 13, 535-541.
|
 Esco M.R., Flatt A.A., Nakamura F.Y. (2017) Agreement between a smartphone pulse sensor application and electrocardiography for determining lnRMSSD. The Journal of Strength & Conditioning Research 31, 380-385.
|
 Fatisson J., Oswald V., Lalonde F. (2016) Influence diagram of physiological and environmental factors affecting heart rate variability: an extended literature overview. Heart International 11, e32.
|
 Fitz-Clarke J.R., Morton R., Banister E. (1991) Optimizing athletic performance by influence curves. Journal of Applied Physiology 71, 1151-1158.
|
 Foster C (1998) Monitoring training in athletes with reference to overtraining syndrome. Medicine and Science in Sports and Exercise 30, 1164-1168.
|
 Fuller C.W., Taylor A., Molloy M.G. (2010) Epidemiological study of injuries in international rugby sevens. Clinical Journal of Sport Medicine 20, 179-184.
|
 Gabbett T.J. (2016) The training-injury prevention paradox: Should athletes be training smarter and harder?. British Journal of Sports Medicine 50, 273-280.
|
 Gabbett T.J., Nassis G.P., Oetter E., Pretorius J., Johnston N., Medina D., Rodas G., Myslinski T., Howells D., Beard A. (2017) The athlete monitoring cycle: a practical guide to interpreting and applying training monitoring data. British Journal of Sports Medicine 15, 1451-1452.
|
 Gisselman A.S., Baxter G.D., Wright A., Hegedus E., Tumilty S. (2016) Musculoskeletal overuse injuries and heart rate variability: Is there a link?. Medical Hypotheses 87, 1-7.
|
 Herman L., Foster C., Maher M., Mikat R., Porcari J. (2006) Validity and reliability of the session RPE method for monitoring exercise training intensity: original research article. South African Journal of Sports Medicine 18, 14-17.
|
 Higham D.G., Hopkins W.G., Pyne D.B., Anson J.M. (2014) Relationships between rugby sevens performance indicators and international tournament outcomes. Journal of Quantitative Analysis in Sports 10, 81-87.
|
 Higham D.G., Pyne D.B., Anson J.M., Eddy A. (2012) Movement patterns in rugby sevens: effects of tournament level, fatigue and substitute players. Journal of Science and Medicine in Sport 15, 277-282.
|
 Higham D.G., Pyne D.B., Anson J.M., Hopkins W.G., Eddy A. (2016) Comparison of activity profiles and physiological demands between international rugby sevens matches and training. The Journal of Strength & Conditioning Research 30, 1287-1294.
|
 Hopkins W.G. (2010) Linear models and effect magnitudes for research, clinical and practical applications. Sportscience 14, 49-57.
|
 Hopkins W.G. (2015) Spreadsheets for analysis of validity and reliability. Sportsscience 19, 36-42.
|
 Impellizzeri F.M., Rampinini E., Coutts A.J., Sassi A., Marcora S.M. (2004) Use of RPE-based training load in soccer. Medicine and Science in Sports and Exercise 36, 1042-1047.
|
 Impellizzeri F.M., Rampinini E., Marcora S.M. (2005) Physiological assessment of aerobic training in soccer. Journal of Sports Sciences 23, 583-592.
|
 Jobson S.A., Passfield L., Atkinson G., Barton G., Scarf P. (2009) The analysis and utilization of cycling training data. Sports Medicine 39, 833-844.
|
 Jones A.M., Vanhatalo A. (2017) The ‘Critical Power’concept: Applications to sports performance with a focus on intermittent high-intensity exercise. Sports Medicine 47, 65-78.
|
 Kenney W.L. (1985) Parasympathetic control of resting heart rate: relationship to aerobic power. Medicine and Science in Sports and Exercise 17, 451-455.
|
 Kiviniemi A.M., Tulppo M.P., Wichterle D., Hautala A.J., Tiinanen S., Seppänen T., Mäkikallio T.H., Huikuri H.V. (2007) Novel spectral indexes of heart rate variability as predictors of sudden and non-sudden cardiac death after an acute myocardial infarction. Annals of Medicine 39, 54-62.
|
 Morton R (1997) Modelling training and overtraining. Journal of Sports Sciences 15, 335-340.
|
 Mujika I., Busso T., Lacoste L., Barale F., Geyssant A., Chatard J.-C. (1996) Modeled responses to training and taper in competitive swimmers. Medicine and Science in Sports and Exercise 28, 251-258.
|
 Mujika I., Chatard J.-C., Busso T., Geyssant A., Barale F., Lacoste L. (1995) Effects of training on performance in competitive swimming. Canadian Journal of Applied Physiology 20, 395-406.
|
 Nédélec M., McCall A., Carling C., Legall F., Berthoin S., Dupont G. (2013) Recovery in soccer. Sports Medicine 43, 9-22.
|
 Plews D.J., Laursen P.B., Kilding A.E., Buchheit M. (2012) Heart rate variability in elite triathletes, is variation in variability the key to effective training? A case comparison. European Journal of Applied Physiology 112, 3729-3741.
|
 Plews D.J., Laursen P.B., Le Meur Y., Hausswirth C., Kilding A.E., Buchheit M. (2014) Monitoring training with heart-rate variability: How much compliance is needed for valid assessment?. International Journal of Sports Physiology & Performance 9, 783-790.
|
 Plews D.J., Laursen P.B., Stanley J., Kilding A.E., Buchheit M. (2013) Training adaptation and heart rate variability in elite endurance athletes: opening the door to effective monitoring. Sports Medicine 43, 773-781.
|
 Plews D.J., Scott B., Altini M., Wood M., Kilding A.E., Laursen P.B. (2017) Comparison of heart rate variability recording with smart phone photoplethysmographic, Polar H7 chest strap and electrocardiogram methods. International Journal of Sports Physiology and Performance , 1-17.
|
 Suarez-Arrones L.J., Nuñez F.J., Portillo J., Mendez-Villanueva A. (2012) Running demands and heart rate responses in men rugby sevens. The Journal of Strength & Conditioning Research 26, 3155-3159.
|
 West S., Williams S., Cross M., Howells D., Mobed R., Kemp S., Stokes K. (2017) Workload spikes combined with high cumulative load is associated with increased injury risk in elite rugby sevens players. British Journal of Sports Medicine 51, 408-408.
|
 Williams S., Booton T., Watson M., Rowland D., Altini M. (2017) Heart rate variability is a moderating factor in the workload-injury relationship of competitive CrossFit athletes. Journal of Sports Science & Medicine 16, 443-449.
|
 Wood R.E., Hayter S., Rowbottom D., Stewart I. (2005) Applying a mathematical model to training adaptation in a distance runner. European Journal of Applied Physiology 94, 310-316.
|
 World Medical Association (2013) World Medical Association Declaration of Helsinki. Ethical principles for medical research involving human subjects. JAMA 310, 2191-2194.
|
|
|
|
|
|
|