|
|
|
ABSTRACT |
One-Day cricket’s eternal problem is how to fairly account for an interruption that occurs during a team’s innings. Several methods have been applied in the past, some more successfully than others. Numerous articles have been written about different target resetting methods applicable in one-day international cricket and how they “favour ”one team over another. In this paper we use an alternative approach looking at the psychic ability of four target resetting methods and compare how well they predict the final score based on the present state of the first innings. We attempt to convert each of methods we investigate into a ball-by-ball predictive tool. We introduce a terminal interruption to the first innings at every ball and compute the predicted final score. We ascribe a nominal value to the difference between the final achieved score and the prediction given by each method. We compute our own ‘Psychic Metric’ to enable a comparison between the four methods. We also develop a computer package to manipulate the data from matches in which the first innings was completed. |
Key words:
Cricket, predicting scores, psychic abilities
|
Key
Points
- Predicting the final score.
- Creation of a method of comparison.
|
Many papers have been written about mathematics and cricket, on topics such as optimal batting strategies (Clarke, 1988), player performance measurements (Allsopp and Clarke, 2000; Lewis, 2004) and target resetting methods (Armstrong, 1994; Bhogle, 1999; Gurram and Narayanan, 2004, Jayadevan, 2002; Lewis, 1998; 2004). Several of the target resetting papers used historical data to build the method. We borrow this approach to evaluate the ability of four methods to predict the score achieved by the first batting team (Team 1), using data from 173 matches, some of which involved stoppages. Given that Team 2’s target in an uninterrupted match is dependent on Team 1’s final score, any target resetting method would need to have a reasonably good estimate of what Team 1 is likely to score. Our intention is to formulate a metric that could be used to assess the predictive ability of a target resetting method.
The “Fairness Metric”Gurram and Narayanan’s (2004) paper addresses the fundamental issue of how “fair ”some of the better-known target resetting methods are. Additionally, it provides some of the motivation for our work and as such, it is worthwhile considering issues raised by the paper. Firstly, in attempting to quantify how “fair ”the chosen methods are, the paper fails to address some very important issues in relation to how the game is played. Gurram and Narayanan’s (2004) fairness metric was only applied to games that went beyond the 25th over in the second innings (according to ICC rules when the paper was written, a result would only be recorded if an interrupted match lasted for more than 25 overs per side). Although the paper states that only games with “no interruption ”were examined, “no interruption ”was defined as a game consisting of two innings, where the innings concluded only when all balls had been bowled or all wickets had been lost. Consequently, this definition fails to acknowledge games that were interrupted, but resumed with no overs lost; and those games in which an interruption “threatened”, but did not eventuate. By not taking these “interruptions ”into account and given that Gurram and Narayanan only dealt with the second innings, one of the most important factors of one-day cricket is completely disregarded - the psychology of the game. Secondly, Gurram and Narayanan, 2004 originally found that the Average Run Rate method was “fairest ”(with a fairness metric of 0.708). By their own admission (in section 2.1.1 of the paper), ARR has many downfalls, particularly the fact that the wickets remaining are not taken into account. Although ARR was once used in One Day International Cricket, it was eventually dismissed, due its many shortcomings, including the fact that it leads to an unfair advantage to Team 2 (as discussed in Ovens, 2004). Consequently, it is worrying to see that ARR is still favourably viewed even when 20% of the “mismatches ”are forgiven. It should also be noted that Gurram and Narayanan have no clearly defined rule to determine which mismatched overs should be removed; leading to the suspicion that one is able to take out particular overs in order to make one method perform better than another. On top of all this, their fairness metric asserts that of all the methods reviewed, Jayadevan’s (2002) is the fairest, although the paper (like many others) states that one of the shortcomings of the Jayadevan method is that it fails to sufficiently address the issue of fallen wickets. The Duckworth/Lewis method appears to adequately take into account the effect of wicket loss, leading one to assume that this method would be “fairer ”(Duckworth and Lewis, 1996). Over-all, Gurram and Narayanan’s concept of computing a fairness metric as a way of comparing target resetting methods is laudable, but perhaps there are other factors that should be taken into account, e.g. the psychology of batting second. This provides motivation for our undertaking to formulate a metric that addresses these factors.
The four methods chosen were Average Run Rate, PARAB, Duckworth/Lewis and Jayadevan. Average Run Rate (ARR) and PARAB (P) methods were chosen as they are easily adapted to predict a score achieved by the conclusion of an innings, using only the present runs scored and balls bowled. Duckworth/Lewis (D/L) was chosen as it is the current rain-rule used in One-Day international cricket. Jayadevan’s method (J) was chosen as a potential alternative rain-rule that could be used to replace D/L (as discussed in Ovens, 2004). These last two methods both required manipulation to be able to be turned into predictive tools. In order to use D/L as a predictive tool, we adapted the target formula (Equation 1) to the form shown in Equation 2. In the case of a stoppage occurring during Team 1’s innings, Jayadevan’s method is applied as follows: To convert Jayadevan’s method into a predictive tool, we note that step 3 gives us 0% overs remaining, which in turn means that steps 4, 5 and 6 are unnecessary and the effective normal score is the normal score obtained in step 2. Thus, the result obtained in step 9 would be the target for Team 2, consequently the predicted score for Team 1 is one run less. It is worth noting that if Team 1’s score is zero then this method results in a predicted score of zero. Further scrutiny of Jayadevan’s method also indicates that, when using this as a predictive tool, the multiplication factor from step 8 will always be less than 1. Software was written so that predictions using each method could be easily calculated from the present state of the match. Using the data provided by Champion Data we computed the predictions for each method on each ball of the first innings of the 173 matches. Figure 1 shows a screen shot of the predictions being computed ball by ball for ODI #1620. We then defined OverProjijk as the difference between the prediction (on ball i, in match j, using method k) and the actual runs scored on ball i, match j. This OverProjijk is then used to compute four different alternative Psychic Metrics; by ball, by delta, by delta/ball and by arbitrary. We define the four Psychic Metrics as follows: By observing equation 3, one can clearly see that the by ball method gives a squared difference, weighted by ball, an approach similar to that used to compute variance. Equation 4 (by delta) subtracts the squared proportion (of over projection divided by total) from 1 where a result closer to 1 indicates a better prediction. Equation 5 then weights this method by ball, such that a result closer to i indicates a better prediction. Equation 6 allows for a nominal value to be ascribed to a range of differences (which are able to be defined by the user). Using the four psychic metrics, we were able to come up with scores scaled for each method.
We present examples using two of the psychic metrics to demonstrate the results obtained from the work undertaken in this paper. Psychic scale was presented in Table 1. 1. An example of the Arbitrary Psychic Metric To illustrate how the arbitrary psychic metric could be used, we have, for each ball, scored the absolute difference between the actual and predicted runs, according to the following scale. This score was then multiplied by the balls remaining in the innings. Summing over the entire innings gives the following representation for Match j, Method k. From this representation, it is clear that the maximum possible raw score for any 50 over match would be 451,500 and thus: As can be seen from Table 2, the mean for D/L is maximal over the four methods, although equally has the highest standard deviation. J is by far the most stable but also consistently less able to predict the final score. It is also interesting to note that none of the computed confidence intervals overlap that of the D/L method, indicating that there is a significant difference at the 5% level. Figure 2 shows the average differences for each method, over the course of 300 balls. As can be seen in the above graph, the Jayadevan, PARAB and Average Run Rate methods do poorly when compared to the Duckworth/Lewis method. One expects that as we get closer to the end of the game, the predictions will improve for all methods and this is evidenced in the above graph. The following table shows a comparison between the four methods with 10 overs remaining. Table 3 shows that, at this point in the innings, ARR predicts, on average, 17.29 runs below the actual score, compared with D/L, 4.50 runs below, Jayadevan, 31.13 runs below and PARAB, 41.60 runs below. It is interesting to note that the minimum and maximums for D/L are almost symmetrical about zero, whereas the other three methods are asymmetrical about zero being further on the negative side. 2.An example using the By Delta/Ball Psychic Metric As can be seen from Table 4, the means of ARR and D/L are both significantly close to one another and reasonably close to the ‘ideal’ average final score (150). We have not computed the confidence levels, as these values are bounded above. Table 5 shows a comparison between the four methods when the last 10 overs are being played. At this stage of the game, the Duckworth/Lewis method’s average predicted final score (267.66) is closest to the ‘ideal’ average score, closely followed by Average Run Rate (268.26), Jayadevan (264.24) and PARAB (258.94). The ideal average is 270.5 runs. Due to the definition of the metric, no minimum or maximum values have been given. The potential minimum is for all methods is 0 and the potential maximum is 300, therefore no further information would be gained by including these values. The software used to obtain these results was adapted to allow us to check for any games that may have heavily influenced the results. This was done by visual inspection, using graphs plotted by the software.
We expected to find that both the Average Run Rate and PARAB methods would not perform well when compared to other methods, as it has been demonstrated time and time again that they have potentially serious shortcomings as target resetting methods. These shortcomings imply that the methods will not perform well over extended periods, however they were included to aid in comparison. Furthermore, we also expected that Jayadevan’s method would not perform well, as it is a method that is not designed to predict scores but rather to reset the target, yet, as mentioned earlier, a target resetting method should have a reasonably accurate estimate of Team 1’s expected final score. The intention of this work was to create a metric that could be used to assess the accuracy of a target resetting method in computing Team 1’s expected final score. This leads us to conclude that Jayadevan’s method would work best only when Team 1 has completed its innings. As the Duckworth/Lewis method is readily adaptable as both a predictive and a target resetting tool, it met our expectations to surpass the other methods. Assessing the four methods with our various psychic metrics, we conclude that the Duckworth/Lewis method is the most reliable in computing the expected final score of Team 1 and therefore should be chosen above other potential target resetting methods. In attempting to create a metric to assess a target resetting method, we have inadvertently introduced potential weaknesses. Firstly, the data set we used, kindly supplied by Champion Data, consisted of only 173 matches, most of which came from series in which Australia was involved. Consequently, it was not a truly random sample. A more accurately representative data set, consisting of matches from various series and between various teams would allow us to address this problem. Secondly, in order to measure the methods against our psychic metric “ruler”, we needed to adapt each of the methods to produce a 50 over score. For ARR, PARAB and D/L this is readily achieved, but for J this produces problems, as one of the assumptions underlying the method is that Team 2 cannot possibly face more overs than Team 1. Whilst this is true, it is a technical deficiency of the J method that restricts it from providing a prediction of Team 1’s final score. Thirdly, in this work, we have only addressed terminal stoppages, which may have biased the results. In the future, we plan to look at multiple stoppages, to see whether they affect a team’s predicted final score. Another area that could be looked at would be to give such metrics the ability to be classified by both country and ICC ranking. This would allow one to deal with inefficiencies stemming from the issue of low scoring teams playing high scoring teams and the problems this causes when target resetting methods are applied. An additional aspect to consider for possible future research would be to investigate if suggested new rules affect how a team plays its innings and if this in turn affects the final score prediction. As an extension of this, one could look at if and how the batting order affects a final score prediction (like Bukiet et al., 1997 and 2006).
ConclusionsOverall, in our opinion, D/L is presently the best available target resetting method and is the most accurate at predicting Team 1’s final score. We also believe that a single number cannot accurately summarise a target resetting method, rather a suite of measures are required. We see the opportunity for potential future research in order to investigate multiple stoppages to see how they affect the ability to predict a team’s final score.
|
AUTHOR BIOGRAPHY |
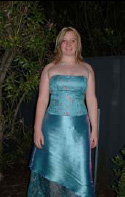 |
Barbara J. O’Riley |
Employment: Web Mistress, Eureka! Beads. |
Degree: Currently completing BA/BSci |
Research interests: Languages and mathematics, mathematics in sports. |
E-mail: bori1@students.monash.edu.au |
|
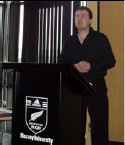 |
Mathew Ovens |
Employment: LMS Training Officer, Monash University. |
Degree: B.Sc(Hons) |
Research interests: Mathematics in cricket, mathematics education and online learning management systems. |
E-mail: matthew.ovens@its.monash.edu.au |
|
|
|
REFERENCES |
 Allsopp P., Clarke S., Cohen G., Langtry T. (2000) Methods for quantifying performances in One-Day Cricket. Abstract Book of 5th Australian Conference on Maths and Computers in Sport , 28-36.
|
 Armstrong J. (1994) Computer models in cricket. Master Thesis , -.
|
 Bhogle S. (1999) . Rediff ont net , -.
|
 Bukiet B., Harold E., Palacios J. (1997) A Markov chain approach to baseball. Operations Research 45, 14-23.
|
 Bukiet B., Ovens M., Hammond J, de Mestre N. (2006) A mathematical modelling approach to One-Day Cricket Batting Orders. Proceedings of 8th Conference on Mathematics and Computers in Sport , 58-67.
|
 Clarke S. R. (1988) Dynamic programming in one-day cricket - optimal scoring rates. Journal of the Operational Research Society 39, 331-337.
|
 Duckworth F., Lewis T., Cohen G., Langtry T. (2002) Can the probability of winning a One-day Cricket Match be maintained across a stoppage? MathSport. 6, 141-154.
|
 Duckworth F., Lewis T., Cohen G., Langtry T. (2002) Review of the application of the Duckworth/Lewis method of target resetting in One-Day Cricket. 6, 127-140.
|
 Duckworth F., Lewis T., de Mestre N. (1996) A fair method for resetting the target in interrupted one-day cricket matches. Proceedings of Third Conference on Mathematics and Computers in Sport , 51-68.
|
 Gurram M., Narayanan S., Morton H., Ganesalingam S. (2004) Comparison of the methods to reset targets for interrupted One-Day Cricket Matches. Proceedings of 7th Australasian Conference on Mathematics and Computers in Sport , 165-171.
|
 Jayadevan V. (2002) A new method for the computation of target scores in interrupted, limited-over cricket matches. Current Science 83, 577-586.
|
 Lewis T., Duckworth F., de Mestre N., Kumar K. (1998) Developments in the Duckworth-Lewis (D/L) method of target-resetting in one-day cricket matches. Proceedings of Fourth Conference on Mathematics and Computers in Sport , 131-151.
|
 Lewis T., Morton H., Ganesalingam S. (2004) Steps towards fairer One-Day Cricketing measures of performance. Proceedings of 7th Australasian Conference on Mathematics and Computers in Sport , 198-213.
|
 Ovens M, Morton H., Ganesalingam S. (2004) If it rains, do you still have a sporting chance?. Proceedings of 7th Australasian Conference on Mathematics and Computers in Sport , 242-252.
|
|
|
|
|
|
|