|
|
|
ABSTRACT |
The purpose of this study was to evaluate the agreement of the vagal-related heart rate variability index, log-transformed root mean square of successive R-R intervals (lnRMSSD), measured under ultra-short-term conditions (< 60 seconds) with conventional longer term recordings of 5 minutes in collegiate athletes under resting and post-exercise conditions. Electrocardiographic readings were collected from twenty-three athletes within 5-minute segments at rest and at 25-30 minutes of supine recovery following a maximal exercise test. From each 5-minute segment, lnRMSSD was recorded as the criterion measure. Within each 5-minute segment, lnRMSSD was also determined from randomly selected ultra-short-term segments of 10-, 30-, and 60-seconds in length, which were compared to the criterion. When compared to the criterion measures, the significant intraclass correlation (from 0.98 to 0.81, p < 0.05) and typical error (from 0.11 to 0.34) increased as ultra-short-term measurement duration decreased (i.e., from 60 seconds to 10 seconds). In addition, the limits of agreement (Bias ± 1.98 SD) increased as ultra-short-term lnRMSSD duration decreased as follows: 0.00 ± 0.22 ms, -0.07 ± 0.41 ms, -0.20 ± 0.94 ms for the 60-, 30-, and 10-second pre-exercise segments, respectively, and -0.15 ± 0.39 ms, -0.14 ± 0.53 ms, -0.12 ± 0.76 ms for the 60-, 30-, and 10-second post-exercise segments, respectively. This study demonstrated that as ultra-short-term measurement duration decreased from 60 seconds to 10 seconds, the agreement to the criterion decreased. Therefore, 60 seconds appears to be an acceptable recording time for lnRMSSD data collection in collegiate athletes. |
Key words:
Performance, training load, athletic monitoring, autonomic, parasympathetic
|
Key
Points
- The log-transformed root mean square of successive R-R intervals (lnRMSSD) is a vagal-related heart rate variability index that has become a promising method for monitoring individual adaptation to training when measured during resting or post-exercise conditions.
- This study demonstrated that lnRMSSD of the 60-second electrocardiogram segments could likely serve as an alternative to traditional 5-minute measures in resting and exercise recovery conditions.
- Due to the current results in athletes and previous investigation involving non-athletes, the utility of ultra-sound-term lnRMSSD measures, especially 60 seconds in duration, within field setting for monitoring athletes at rest and in response to stress appears promising.
|
An emerging training status metric being used in the realm of sports performance is heart rate variability (HRV) (Buchheit, 2014). This non-invasive marker of cardiovascular-autonomic modulation has recently been viewed as one of the more promising methods of monitoring individual adaptation to training when measured during resting or post-exercise conditions (Buchheit, 2014; Plews et al., 2013; Stanley et al. 2013). For instance, HRV has been shown to be an effective tool for program periodization (Kiviniemi et al., 2007; 2010), reflecting training load and recovery status (Chen et al. 2011; Pichot et al., 2003), aiding in the detection of overtraining (Baumert et al., 2006; Mourot et al., 2004; Le Meur et al. 2013; Plews et al., 2012; Tian et al., 2012), and predicting athletic performance (Chalencon et al., 2012; Di Fronso et al., 2012; Plews et al., 2013). Therefore, routine monitoring of HRV appears to provide an objective measure to consider when planning and adjusting training programs. Of the numerous parameters of HRV, the root mean square of successive R-R intervals (RMSSD) is considered the preferred index for athletic monitoring as it appears to be uninfluenced by respiratory rate (Penttilä et al., 2001; Saboul et al., 2013) and is relatively easy to calculate and interpret (Plews et al., 2013). This marker has been shown to reflect training and non-training-related stress that contributes to non-functional overreaching in athletes (Le Meur et al., 2013; Tian et al., 2012). Taken with other training status variables (e.g. performance, training load, mood states, etc.), changes in RMSSD can be indicative of positive or maladaptive responses to training demands (Chen et al., 2011; Oliveria et al., 2012; Tian et al., 2012). It is recommended that standard procedures for short-term assessment of HRV should involve recordings of at least 5 minutes preceded by a 5 minute stabilization period while the subject rests in a supine position (Task Force of the European Society of Cardiology and the North American Society of Pacing and Electrophysiology, 1996). Time constraints in the applied sport setting make standard HRV recordings difficult. Shorter measurement durations are desirable for daily HRV monitoring to enhance convenience and thus increase its potential use among sports teams in field settings. RMSSD has recently been suggested for athletic monitoring due to it being able to reflect parasympathetic activity over a short time frame (i.e., less than 5 minutes) (Plews et al., 2013). However, that recommendation was based on a study that primarily involved sedentary subjects (Hamilton et al., 2004). In fact, most of the research pertaining to the accuracy of ultra-short-term HRV measures has focused on non-athletic and clinical populations (Katz et al., 1999; Nussinovitch et al., 2011; 2012; Salahuddin et al., 2007; Smith et al., 2013). There are no studies to date that compare ultra-short-term HRV to the recommended recording criterion of 5 minutes in a group of athletes at rest and in response to physical stress (e.g., following exercise). The research in this area is needed specifically in athletes as this population typically displays superior cardiac-vagal modulation and higher HRV compared to non-athletes (Aubert et al., 2003; Esco and Williford, 2011). Higher vagal-related HRV indices may not provide a sufficient number of R-R intervals within ultra-short-term recordings to accurately reflect standard 5-minute recordings. Therefore, the purpose of this study was to determine the agreement between ultra-short-term RMSSD indexes of 10-, 30-, and 60-seconds and conventional longer term recordings of 5 minutes in collegiate male athletes under resting and post-exercise conditions. Due to previous research involving non-athletic and clinical subjects (Katz et al., 1999; Nussinovitch et al., 2011; 2012; Salahuddin et al., 2007; Smith et al., 2013; Thong et al., 2003), it is reasonable to hypothesize that ultra-short-term RMSSD measures would agree with the standard 5-minute recordings in a group of collegiate athletes, but the agreement to the criterion would decrease as ultra-short-term measurement duration decreases.
ParticipantsTwenty three male athletes from the National Association for Intercollegiate Athletics participated in the study and provided written informed consent. The study was approved by the University’s Institutional Review Board for Human Participants. The athletes were recruited from the University’s soccer (n = 12, age = 20.9 ± 1.9 years, height = 178.9 ± 7.5 cm, weight = 74.5 ± 7.5 kg, VO2max = 58.1 ± 4.7 ml·kg-1.min-1) and basketball (n = 11, age = 22.0 ± 2.4 years, height = 189.9 ± 4.9 cm, weight = 87.2 ± 9.4 kg, VO2max = 44.7 ± 4.6 ml·kg-1.min-1) teams. All participants completed health-history questionnaires and were apparently healthy, free from cardiopulmonary, metabolic, and orthopedic disorders. Data collection for each participant occurred on any day of the week during the morning hours as close as possible to awakening from sleep (i.e., from 7:00am to 11:00am). Each participant was required to report to the laboratory following an overnight fast, though the consumption of a moderate amount of water was allowed. Participants were told to refrain from consuming stimulants (e.g., caffeine) or depressants (e.g., alcohol) and to avoid strenuous exercise for 24 hours prior to data collection.
ProceduresEach participant performed a maximal graded exercise test via the Bruce Protocol on a Trackmaster treadmill (Full Vision, Inc., Carrollton, TX). The staged protocol began at 1.7 mph at 10% grade with increasing work rate (speed and grade) every 3 minutes until maximal oxygen consumption (VO2max) was reached. Expired gas fractions (oxygen and carbon dioxide) were collected at the mouth and analyzed with a metabolic cart (ParvoMedics TrueOne® 2400 metabolic cart, Sandy, UT, USA). The criteria for VO2max was predetermined as 2 of the following: if there was a plateau in oxygen consumption despite an increased work (± 2 ml·kg-1.min-1); respiratory exchange ratio of > 1.10; and a heart rate within 10 beats of age-predicted maximum (220-age). Before the exercise test each athlete was instructed to lay supine on an athletic training table in a dimly lit, climate controlled laboratory (i.e., temperature and humidity maintained at approximately 22.2 °C and 50%, respectively) for 10 minutes following accepted recommendations (Task Force of the European Society of Cardiology and the North American Society of Pacing and Electrophysiology, 1996). Following the exercise test, the participants were allowed a 3-minute period to transition from the treadmill before comfortably assuming the supine position again on the athletic training table for a 30-minute period. During the pre- and post-exercise periods of supine rest, the participant’s heart rate and rhythm was assessed via electrocardiogram (ECG) with a modified Lead II arrangement and a Biopac MP100 data acquisition system (Goletta, CA, USA). All data was stored in a Dell PC for analysis. HRV was evaluated following accepted standards for the pre- and post-exercise ECG measures (Task Force of the European Society of Cardiology and the North American Society of Pacing and Electrophysiology, 1996). This involved analyzing the ECG tracings in 300-second (i.e., 5-minute) segments as follows: the last 5 minutes of the 10-minute supine rest period (Pre), and at 25 to 30 minutes post-exercise (Post) of the supine recovery period. No attempt was made to control for respiration as previous research has indicated that RMSSD remains consistent across paced or non-paced breathing patterns (Penttilä et al., 2001; Saboul et al., 2013). The ECG recordings were visually inspected. If any non-sinus beats were found, they were removed and replaced by the adjacent normal cycle. Three or more ectopic beats found within any ECG segment was considered as criteria for exclusion (n = 0). The ECG recordings were converted to a tachogram, which plotted the successive R-R intervals on the y-axis and the number of beats within the 5-minute segment on the x-axis using specialized software (Nevrokard version11.0.2, Izola, Slovenia). From the tachogram, RMSSD was calculated and analyzed for the Pre (RMSSD300Pre) and Post (RMSSD300Post) 5-minute ECG segments. Thereafter, RMSSD parameters were recalculated from within the 5-minute Pre and Post ECG segments at intervals of 60 seconds (RMSSD60Pre and RMSSD60Post, respectively), 30 seconds (RMSSD30Pre and RMSSD30Post, respectively), and 10 seconds (RMSSD10Pre and RMSSD10Post, respectively). The ultra-short-term epochs were randomly selected by drawing numbers that represented each time segment from a cup.
Statistical analysesStatistical analyses were performed using SPSS/PASW software (version 18.0, IBM Corporation, New York, NY, USA). Means and standard deviations (SD) were determined for all the studied variables. Data normality was evaluated with a Shapiro-Wilk test, which determined that the assumption of normality was violated for the absolute RMSSD (p < 0.05). Therefore, natural log (ln) transformations were applied (lnRMSSD). Due to the skewed absolute values and the preference of log-transformed HRV measures for athletic monitoring (Plews et al., 2013), only lnRMSSD values were analyzed within the current study. The difference between the lnRMSSD time segments (i.e., 300-, 60-, 30-, and 10-seconds) was examined with a one-way repeated measures analysis of variance. Bonferroni follow-up tests were used to further explore the differences. Cohen’s d was calculated to determine the effect size of the mean differences (Cohen, 1988) with thresholds considered as trivial (<0.2), small (0.2-0.6), moderate (0.6-1.2), large (1.2-2.0) or very large (>2.0) (Hopkins et al., 2009). Intra-class correlations (ICC) were used to evaluate the agreement between the lnRMSSD parameters. An ICC value between 0 to 0.30 was considered small, 0.31 to 0.49 moderate, 0.50 to 0.69 large, 0.70 to 0.89 very large, and 0.90 to 1.00 near perfect (Hopkins, 2009). The typical error was also determined for each ultra-short-term segment. In addition, Bland-Altman plots were formed to identify the upper and lower limits of agreement: i.e., ± 1.96 SD of the mean difference/bias between the criterion and ultra-short lnRMSSD values (Bland and Altman, 1986). Statistical significance was predetermined as p < 0.05.
Pre-exercise lnRMSSD segmentsTable 1 provides the comparison statistics for the pre-exercise lnRMSSD segments. There were no significant differences between each of the pre-exercise lnRMSSD values and the effect sizes were considered trivial (Cohen’s d = 0.01 for lnRMSSD60Pre, Cohen’s d = 0.12 for lnRMSSD30Pre) to small (Cohen’s d = 0.21 for lnRMSSD10Pre). As measurement duration decreased (i.e., from 60 seconds to 10 seconds), ICC decreased (from 0.98 to 0.81) and typical error increased (from 0.11 to 0.34 ms). The method of Bland-Altman showed that the limits of agreement increased as measurement duration decreased in the pre-exercise condition (Figure 1 and refer to Table 1 for the Bias ± 1.96 SD and upper and lower limits of agreement for each segment).
Post-exercise lnRMSSD segmentsTable 1 also provides the comparison statistics for the post-exercise segments. lnRMSSD60Post (p < 0.001) and lnRMSSD30Post (p = 0.04) were significantly different compared to lnRMSSD300post but the effect sizes weretrivial (Cohen’s d = 0.16 to 0.17). There was no significant difference between lnRMSSD10Post and lnRMSSD300Post (p = 0.23, Cohen’s d = 0.11). As measurement duration decreased in the post-exercise segments (i.e., from 60 seconds to 10 seconds), ICC decreased (from 0.96 to 0.90) and typical error increased (from 0.14 to 0.27 ms). The method of Bland-Altman showed that the limits of agreement increased as measurement duration decreased (Figure 2 and refer to Table 1 for the Bias ± 1.96 SD and upper and lower limits of agreement for each segment).
This investigation evaluated the agreement of ultra-short-term lnRMSSD indexes of 10-, 30-, and 60-seconds with conventional longer term measures of 5 minutes (considered as the criterion) in collegiate male athletes under resting and post-exercise conditions. The main findings were that lnRMSSD of randomly selected 10-, 30-, and 60-seconds ECG strips provided no significant difference and near perfect (lnRMSSD60Pre and lnRMSSD30Pre) to very large (lnRMSSD10Pre) ICC values when compared to the standard 5-minute measurements in the pre-exercise condition. In the post-exercise condition, the criterion was significantly different compared to lnRMSSD60post and lnRMSSD30post. However, the effect size was trivial and the ICCs were considered near perfect. As measurement duration decreased from 60 to 10 seconds in both conditions, the strength of the relationships between the ultra-short-term and criterion measures decreased and the typical error and limits of agreement increased. This was the first study to report acceptable ultra-short-term lnRMSSD values compared to criterion measurement durations in an athletic group of participants. Our results support previous research which demonstrated that ultra-short-term RMSSD parameters (e.g., raw and ln) provided comparable measures to criterion-length segments in non-athletic populations at rest. For instance, Nussinovitch et al., (2011) found good agreement between ultra-short and criterion raw RMSSD in healthy adults. The results showed strong correlations between criterion 5-minute raw RMSSD segments and both 10-second (ICC = 0.96) and 60-second (ICC = 0.91) segments (Nussinovitch et al., 2011). The ICC of the 60-second lnRMSSD compared to the criterion was similar between the current study and Nussinovitch et al. (2011). However, the lnRMSSD10pre segments in our investigation provided lower ICC (i.e., ICC = 0.81 in the current study) than those previously reported in other populations (i.e., ICC = 0.908, Nussinovitch et al., 2012; ICC = 0.909, Nussinovitch et al., 2011). In addition, the 10-second pre-exercise lnRMSSD values showed the largest limits of agreement to the criterion compared to the other ultra-short-term segments of longer duration (i.e., 60-seconds and 30-seconds). The lnRMSSD60pre parameters essentially provided the highest ICC value and tightest limits of agreement compared to the criterion. A possible explanation of the lower relationship between lnRMSSD10pre and criterion measures in our investigation compared to others (Nussinovitch et al., 2011; 2012; Salahuddin et al., 2007) could be due to previous findings of athletes having greater resting HRV compared to non-athletes (Aubert et al., 2003; Esco and Williford, 2011). It is possible that the chance of error was increased in the group of athletes when randomly selecting the 10-second pre-exercise epochs from a longer time segment (i.e., 5-minutes) of higher variability. In addition, athletes tend to have greater vagal tone and lower resting heart rates compared to non-athletes (Dixon et al., 1992). Therefore, it is doubtful that 10-second segments provide a sufficient amount of time to collect an appropriate number of R-R intervals for HRV determination. Since lnRMSSD60pre appeared to provide the highest ICC and tightest limits of agreement to the criterion measure, sport practitioners who plan to use ultra-short-term lnRMSSD in athletes under resting conditions may want to consider recording periods of at least 60 seconds in duration following a stabilization period of 5 minutes (Task Force of the European Society of Cardiology and the North American Society of Pacing and Electrophysiology, 1996; Young and Leicht, 2011). However, it should be noted that other researchers have used pre-recording stabilization time periods of only 1 minute (Plews et al., 2012). Therefore, determining the optimal time course for stabilization preceding lnRMSSD recordings in athletes is an area that warrants further investigation. The maximal exercise test was performed in this study for the purposes of determining if lnRMSSD measures of less than 60 seconds would provide acceptable measures of agreement to criteria during a period of recovery from physical stress. Post-exercise HRV has been utilized for monitoring athletic training status as it could reveal positive adaptation to exercise recovery that may not be fully expressed when evaluating resting conditions alone (Buchheit et al., 2007; 2008; Javorka et al., 2002). Though lnRMSSD60Post and lnRMSSD30Post were significantly different compared to the criterion, the difference was trivial. In addition, near perfect ICCs were observed when comparing each of the post-exercise ultra-short-term lnRMSSD segments to lnRMSSD300Post. Furthermore, the typical error and limits of agreement increased as measurement duration decreased. However, lnRMSSD10Post appeared to provide a slightly more acceptable measure of agreement to the criterion in the post-exercise versus the pre-exercise condition. The discrepancy between the pre and post-exercise 10-second lnRMSSD results is most likely due to less variability across time in R-R intervals following a bout of exercise, since parasympathetic modulation is drastically reduced and heart rate increased compared to rest (Goldberger et al., 2006; Javorka et al., 2002). Due to lower variability and a greater number of R-R intervals within a 5-minute post-exercise epoch, the chance of error within the randomly selected 10-second ultra-short-term time segments is reduced. It has been previously suggested that ultra-short raw RMSSD measures may be useful when steady-state conditions are not maintained (Salahuddin et al., 2007) such as during recovery from exercise. Though the current study is the first to specifically address this hypothesis in athletes, Goldberger et al. (2006) demonstrated that raw RMSSD measured in segments of 60-seconds or less reflected parasympathetic rebound immediately following exercise. In addition, Salahuddin, et al., (2007) demonstrated that raw RMSSD of 60 seconds or less reflected criterion measures when monitoring response to mental stress in mobile situations in non-athletic, healthy adults. Ultra-short-term lnRMSSD measures appear to be valid for monitoring response to stress in athletes. However, as stated with the pre-exercise condition, ultra-short recordings of 60 seconds in duration appear to provide the best agreement with criterion measures. The decision of only analyzing lnRMSSD could be considered a limitation of the current study. However, we chose to evaluate this specific marker for several reasons. First, lnRMSSD has recently been suggested by respected sport scientists as the preferred HRV index for athletic monitoring (Buchheit, 2014; Plews et al., 2013). Second, other time domain measures of HRV, such as the standard deviation of normal-to-normal intervals (SDNN), the number of normal-to-normal intervals differing by 50 msec from the preceding interval (pNN50), and the HRV triangular index, have not been shown to agree with criterion 5-minute segments when measured in ultra-short-term recordings in previous studies (Nussinovitch et al., 2011; 2012; Salahuddin et al., 2007). Thus, lnRMSSD may be the only time-domain measure that is precise during ultra-short-term recordings of equal to or less than 60 seconds (Salahuddin et al., 2007). Third, lnRMSSD involves relatively simple calculations that are not as difficult to comprehend compared to more complex HRV methodology, such as the frequency domain, which may involve expensive specialized equipment and software (Plews et al., 2013). Fourth, lnRMSSD is less influenced by respiration rate compared to frequency domain indices, thus serving as a favorable parameter for ambulatory measures (Penttilä et al., 2001; Saboul et al., 2013). Fifth, lnRMSSD also appears to be an accepted time-domain parameter for assessing parasympathetic modulation, which is important for monitoring athletes (Le Meur et al., 2013). Regarding the latter point from the previous paragraph, two recent studies showed that RMSSD parameters identified overreaching states in elite female wrestlers (Tian et al., 2012) and trained male triathletes (Le Meur et al., 2013). In addition, several studies have suggested that vagal-indexes of HRV provided a superior method of program periodization when compared to traditional approaches (Kiviniemi et al., 2007; 2010). Furthermore, HRV parameters (including lnRMSSD) have been suggested as having potential to predict the achievement of optimal performance (Chalencon et al. 2012; Di Fronso et al., 2012; Manzi et al., 2009). However, the protocols within the aforementioned studies involved HRV assessment of longer durations than 60 seconds. The athletes in the current study were not analyzed over a long-term training season. Therefore, future research should investigate the efficacy of ultra-short HRV measures in its application to monitoring training. Determining whether ultra-short measures reflect training load and recovery status is warranted. Because the 60-second ultra-short-term lnRMSSD provided acceptable levels of agreement to the criterion values in the current study, these measures may be valid for athletic assessment. However, more research is needed before widespread implementation of ultra-short-term HRV is adopted in field and especially laboratory settings.
It is likely that shorter HRV measurement durations may increase the potential use of HRV in field settings. Until the current study it was unclear whether lnRMSSD measured within 60-seconds or less would provide acceptable measures of agreement with recordings that followed the recommended time requirements in athletes. We found very large to near perfect relationships between the ultra-short-term and criterion measures. However, it should be noted that the 60-second segments provided the strongest correlation and tightest limits of agreement in the resting and exercise recovery conditions. Due to these results, the utility of ultra-short-term lnRMSSD measures, especially 60 seconds in duration, within field settings for monitoring athletes at rest and in response to stress appears promising. The lower time requirement may allow for more data collection under less time constraints which could increase the implementation of HRV monitoring in athletes by coaches and sports scientists. The need for frequent recording of HRV in the field for monitoring purposes places a high compliance demand on athletes (Buchheit et al., 2014) thus making ultra-short measures attractive for longitudinal usage. Furthermore, technological advancements have made available more convenient athletic field tools of lnRMSSD that utilize measurement durations of less than the established 5-minute standard (Flatt and Esco, 2013). Therefore, the current findings of the near perfect agreement between 60 seconds and criterion lnRMSSD measurement durations may also be important in the development of technology that enhances the practicality of HRV monitoring among athletes.
|
AUTHOR BIOGRAPHY |
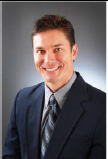 |
Michael R. Esco |
Employment: Associate Professor of Exercise Science Co-Director of the Human Performance Laboratory, Auburn University at Montgomery, Montgomery, AL, USA |
Degree: PhD |
Research interests: Heart rate variability, physiological adaptations to training, sports performance, cross-validation research |
E-mail: mesco@aum.edu |
|
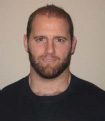 |
Andrew A. Flatt |
Employment: Adjunct Instructor and Visiting Researcher, Human Performance Laboratory, Auburn University at Montgomery Montgomery, AL, USA |
Degree: MS |
Research interests: Heart rate variability, sports performance testing, strength and conditioning |
E-mail: andrew_flatt@hotmail.com |
|
|
|
REFERENCES |
 Aubert A.E., Seps B., Beckers F (2003) Heart rate variability in athletes. Sports Medicine 33, 889-919.
|
 Baumert M., Brechtel L., Lock J., Hermsdorf M., Wolff R., Baier V., Voss A (2006) Heart rate variability, blood pressure variability, and baroreflex sensitivity in overtrained athletes. Clinical Journal of Sport Medicine 16, 412-417.
|
 Baumgartner T.A., Chung H (2001) Confidence limits for intraclass reliability coefficients. Measurement in Physical Education and Exercise Science 5, 179-188.
|
 Bland J.M., Altman D.G (1986) Statistical methods for assessing agreement between two methods of clinical measurement. Lancet i , 307-310.
|
 Buchheit M (2014) Monitoring training status with HR measures: Do all roads lead to Rome. Frontiers in Physiology 27, 73-.
|
 Buchheit M., Laursen P.B., Ahmaidi S (2007) Parasympathetic reactivation after repeated sprint exercise. American Journal of Physiology 293, H133-H141.
|
 Buchheit M., Millet G., Parisy A., Pourchez S., Laursen P., Ahmaidi S (2008) Supramaximal training and postexercise parasympathetic reactivation in adolescents. Medicine and Science in Sports and Exercise 40, 362-371.
|
 Chalencon S., Busso T., Lacour J.R., Garet M., Pichot V., Connes P., Gabel C.P., Roche F., Barthélémy J.C (2012) A model for the training effects in swimming demonstrates a strong relationship between parasympathetic activity, performance and index of fatigue. PloS One 7, e52636-.
|
 Chen J.L., Yeh D.P., Lee J.P., Chen C.Y., Huang C.Y., Lee S.D., Chen C.C., Kuo T., Kao C.L., Kuo C.H (2011) Parasympathetic nervous activity mirrors recovery status in weightlifting performance after training. Journal of Strength and Conditioning Research 25, 1546-1552.
|
 Cohen J (1988) . Statistical Power Analysis for the Behavioral Sciences. Hillsdale (NJ). Lawrence Erlbaum.
|
 Di Fronso S., Delia G., Robazza C., Bortoli L., Bertollo M (2012) Relationship between performance and heart rate variability in amateur basketball players during playoffs. Sport Science and Health 8, 45-.
|
 Dixon E.M., Kamath M.V., McCartney N., Fallen E.L (1992) Neural regulation of heart rate variability in endurance athletes and sedentary controls. Cardiovascular Research 26, 713-719.
|
 Esco M.R., Williford H.N (2011) Cardiovascular autonomic modulation in collegiate male basketball players. Journal of Exercise Physiology 14, 35-42.
|
 Flatt A.A., Esco M.R (2013) Validity of the ithleteTM smart phone application for determining ultra-short-term heart rate variability. Journal of Human Kinetics 39, 85-92.
|
 Goldberger J.J., Le F.K., Lahiri M., Kannankeril P.J., Ng J., Kadish A.H (2006) Assessment of parasympathetic reactivation after exercise. American Journal of Physiology 290, H2446-H2452.
|
 Hamilton R.M., Mckechnie P.S., Macfarlane P.W (2004) Can cardiac vagal tone be estimated from the 10-second ECG. International Journal of Cardiology 95, 109-115.
|
 Hopkins W.G (2009) A scale of magnitude for effect statistics. , -.
|
 Hopkins W.G., Marshall S.W., Batterham A.M., Hanin J (2009) Progressive statistics for studies in sports medicine and exercise science. Medicine and Science in Sports and Exercise 41, 3-12.
|
 Javorka M., Zila I., Balharek T., Javorka K (2002) Heart rate recovery after exercise: relations to heart rate variability and complexity. Brazilian Journal of Medical and Biological Research 35, 991-1000.
|
 Katz A., Liberty I.F., Porath A., Ovsyshcher I., Prystowsky E.N (1999) A simple bedside test of 1-minute heart rate variability during deep breathing as a prognostic index after myocardial infarction. American Heart Journal 138, 32-38.
|
 Kiviniemi A.M., Hautala A.J., Kinnunen H., Nissilä J., Virtanen P., Karjalainen J., Tulppo M (2010) Daily exercise prescription on the basis of HR variability among men and women. Medicine and Science in Sports and Exercise 42, 1355-1363.
|
 Kiviniemi A.M., Hautala A.J., Kinnunen H., Tulppo M.P (2007) Endurance training guided individually by daily heart rate variability measurements. European Journal of Applied Physiology 101, 743-751.
|
 Le Meur Y., Pichon A., Schaal K., Schmitt L., Louis J., Gueneron J., Vidal P.P., Hausswirth C (2013) Evidence of parasympathetic hyperactivity in functionally overreached athletes. Medicine and Science in Sports and Exercise 45, 2061-2071.
|
 Manzi V., Castagna C., Padua E., Lombardo M., D’Ottavio S., Massaro M., Volterrani M., Iellamo F (2009) Dose-response relationship of autonomic nervous system responses to individualized training impulse in marathon runners. American Journal of Physiology 296, H1733-H1740.
|
 Mourot L., Bouhaddi M., Perrey S., Cappelle S., Henriet M.T., Wolf J.P., Rouillon J.D., Regnard J (2004) Decrease in heart rate variability with overtraining: assessment by the Poincare plot analysis. Clinical Physiology and Functional Imaging 24, 10-18.
|
 Nussinovitch U., Cohen O., Kaminer K., Ilani J., Nussinovitch N (2012) Evaluating reliability of ultra-short ECG indices of heart rate variability in diabetes mellitus patients. Journal of Diabetes Complications 26, 450-453.
|
 Nussinovitch U., Elishkevitz K.P., Katz K., Nussinovitch M., Segev S., Volovitz B., Nussinovitch N (2011) Reliability of ultra-short ECG indices for heart rate variability. Annals of Noninvasive Electrocardiology 16, 117-122.
|
 Oliveira R.S., Leicht A.S., Bishop D., Barbero-Álvarez J.C., Nakamura F.Y (2012) Seasonal changes in physical performance and heart rate variability in high level futsal players. International Journal of Sports Medicine 34, 424-430.
|
 Penttilä J., Helminen A., Jartti T., Kuusela T., Huikuri H.V., Tulppo M.P., Coffeng R., Scheinin H (2001) Time domain, geometrical and frequency domain analysis of cardiac vagal outflow: effects of various respiratory patterns. Clinical Physiology 21, 365-376.
|
 Pichot V., Roche F., Gaspoz J.M., Enjolras F., Antoniadis A., Minini P., Costes F., Busso T., La Cour J.R., Barthelemy J.C (2003) Relation between heart rate variability and training load in middle-distance runners. Medicine and Science in Sports and Exercise 2, 1729-1736.
|
 Plews D. J., Laursen P. B., Kilding A. E., Buchheit M (2012) Heart rate variability in elite triathletes, is variation in variability the key to effective training? A case comparison. European Journal of Applied Physiology 112, 3729-3741.
|
 Plews D.J., Laursen P.B., Stanley J., Kilding A.E., Buchheit M (2013) Training adaptation and heart rate variability in elite endurance athletes: Opening the door to effective monitoring. Sports Medicine 43, 773-781.
|
 Saboul D., Pialoux V., Hautier C (2013) The impact of breathing on HRV measurements: Implications for the longitudinal follow-up of athletes. European Journal of Sport Sciences 13, 534-542.
|
 Salahuddin L., Cho J., Jeong M.G., Kim D (2007) Ultra short term analysis of heart rate variability for monitoring mental stress in mobile settings. Conference Proceedings: IEEE Engineering in Medicine and Biology Society 2007, 4656-4659.
|
 Smith A.L., Owen H., Reynolds K.J (2013) Heart rate variability indices for very short-term (30 beat) analysis. Part 2: validation. Journal of Clinical Monitoring and Computing 27, 577-585.
|
 Stanley J., Peake J.M., Buchheit M (2013) Cardiac parasympathetic reactivation following exercise: implications for training prescription. Sports Medicine 43, 1259-1277.
|
 Task Force of the European Society of Cardiology and the North American Society of Pacing and Electrophysiology (1996) Heart rate variability: standards of measurement, physiological interpretation and clinical use. Circulation 93, 1043-1065.
|
 Thong T., Li K., McNames J., Aboy M., Goldstein B (2003) Accuracy of ultra-short heart rate variability measures. Conference Proceedings: IEEE Engineering in Medicine and Biology Society 3, 2424-2427.
|
 Tian Y., He Z.H., Zhao J.X., Tao D.L., Xu K.Y., Earnest C.P., McNaughton L.R (2012) Heart rate variability threshold values for early-warning non-functional overreaching in elite women wrestlers. Journal of Strength and Conditioning Research 27, 1511-1519.
|
 Young F.L., Leicht A.S (2011) Short-term stability of resting heart rate variability: influence of position and gender. Applied Physiology, Nutrition, and Metabolism 36, 210-218.
|
|
|
|
|
|
|