|
|
|
ABSTRACT |
The search for monitoring tools that provide early indication of injury and illness could contribute to better player protection. The aim of the present study was to i) determine the feasibility of and adherence to our monitoring approach, and ii) identify variables associated with up-coming illness and injury. We incorporated a comprehensive set of monitoring tools consisting of external load and physical fitness data, questionnaires, blood, neuromuscular-, hamstring, hip abductor and hip adductor performance tests performed over a three-month period in elite under-18 academy soccer players. Twenty-five players (age: 16.6 ± 0.9 years, height: 178 ± 7 cm, weight: 74 ± 7 kg, VO2max: 59 ± 4 ml/min/kg) took part in the study. In addition to evaluating adherence to the monitoring approach, data were analyzed using a linear support vector machine (SVM) to predict illness and injuries. The approach was feasible, with no injuries or dropouts due to the monitoring process. Questionnaire adherence was high at the beginning and decreased steadily towards the end of the study. An SVM resulted in the best classification results for three classification tasks, i.e., illness prediction, illness determination and injury prediction. For injury prediction, one of four injuries present in the test data set was detected, with 96.3% of all data points (i.e., injuries and non-injuries) correctly detected. For both illness prediction and determination, there was only one illness in the test data set that was detected by the linear SVM. However, the model showed low precision for injury and illness prediction with a considerable number of false-positives. The results demonstrate the feasibility of a holistic monitoring approach with the possibility of predicting illness and injury. Additional data points are needed to improve the prediction models. In practical application, this may lead to overcautious recommendations on when players should be protected from injury and illness. |
Key words:
Football, artificial intelligence, injury prevention, load management, load monitoring
|
Key
Points
- A comprehensive monitoring approach was feasible and did not lead to adverse events, such as injuries.
- A machine learning approach has shown promise for injury and illness prediction as well as illness detection.
- The analysis was limited by the low number of injuries and illnesses during the study period.
- Future studies should include longer study periods to further improve machine learning models.
|
Load management (i.e., the prescription, monitoring, and adjustment of workload) is intended to objectify the athlete's workload, protect players from injury and illness and maximize their performance (Schwellnus et al., 2016; Soligard et al., 2016). An emerging area of research addresses the potential of load monitoring tools, such as tracking devices or questionnaires, to help predicting injuries or other undesirable events to better protect athletes (Van Eetvelde et al., 2021). In particular, machine learning models have potential application for injury prediction, physical performance prediction, training load and monitoring, players’ career trajectory, club performance, and match attendance (Nassis et al., 2023). However, the predictive accuracy of these machine learning models, e.g., in terms of area under the curve, was not adequate in all studies (Nassis et al., 2023). In soccer, Rossi et al. (2018) conducted a study over a duration of 23 weeks, using global-positioning-system-based training load data to examine the relationship between load and injury occurrence. By generating a training data set and validating their model with a decision tree, the authors demonstrated that certain cut-off values of the exponential weighted moving average (EWMA) of total distance and high-speed running, particularly in players that recently returned to play after injury, were associated with an increased injury risk. Interestingly, other studies in team sports have found that different variables contribute to the prediction of injury. While Rossi et al. (2018) used only tracking data and corresponding ratios, such as the acute:chronic workload ratio (ACWR (Gabbett, 2018)), other author groups added additional variables to their data sets, such as pre-season screening (Ayala et al., 2019), genetic variables (Rodas et al., 2020), physical fitness, motor coordination and anthropometric data (Rommers et al., 2020), age, previous injury, and hamstring strength (Ruddy et al., 2018) resulting in potentially different outcomes. These differences in study design hamper between-study comparisons. Likewise, some of the studies also evaluated different types of injuries ((Van Eetvelde et al., 2021) for review). In addition, measurements performed irregularly, or only in the pre-season, may be insufficient to capture the dynamic nature of the measurement variables. Given the multifactorial nature of non-contact injuries, incorporating additional variables can potentially enhance machine learning models. Such variables include training load data, metrics on physical fitness, objective training/game data, and corresponding physiological responses (e.g., measured via blood-based biomarkers, questionnaires, or neuromuscular performance tests). The inclusion of these different variables may be of importance in view of, i) weak physical fitness is discussed to be a risk factor for illness and injury (Malone et al., 2018; Watson et al., 2017), ii) questionnaires (Saw et al., 2016) and certain vertical jump variables (e.g., counter movement jump (CMJ)) are sensitive to changes in training load, albeit with uncertainty regarding most fatigue-related variables (Claudino et al., 2017; Gathercole et al., 2015) and iii) blood biomarkers decipher various physiological domains such as the immune response and inflammatory response to training load (Haller et al., 2023a; 2023b). Based on the approach and experience of a four-week pilot study (Haller et al., 2022), we aimed to evaluate the feasibility (in terms of injuries and dropouts) of a comprehensive monitoring approach using a variety of monitoring tools, i.e., i) training and game data, ii) blood-based biomarkers covering different physiological domains such as hormonal responses or inflammation, iii) CMJ as surrogate for neuromuscular performance, iv) strength performance tests, i.e. hamstring and hip adductor/abductor strength, and v) questionnaires to assess how well these tools alone or in combination are able to assess and predict injury and illness in a cohort of elite youth soccer players over a three-month period using a linear support vector machine (SVM).
Ethical approvalThe local human ethics committee in Salzburg (GZ 02/2021) approved the experimental design. All procedures were in accordance with the standards of the Declaration of Helsinki of the World Medical Association. Participants were informed about the study both verbally and in writing and gave their written informed consent.
Participants and settingTwenty-five male players (age: 16.6 ± 0.9 years, height: 178 ± 7 cm, weight: 74 ± 7 kg, VO2max: 59 ± 4 ml/min/kg) of an elite European youth soccer team (first national league, UEFA Youth League participant) were included in this study. Following one familiarization session in which participants were informed about the objectives of the study, data were collected over a three-month period from September to December during the 2021/2022 regular season. During this process, the researchers had no influence on the training program, and the coaching staff did not receive feedback on the preliminary results before the study was completed. Figure 1 outlines the study design. A standardized set-up with test stations was used each week to ensure consistency and comparability of measurements. The training focus and number of training sessions per day were also identical across all weeks, with small fluctuations when additional matches were scheduled in midweek. All testing was integrated into the regular training schedule replicating a real-life scenario of an entire soccer team. Specifically, participants were asked to complete questionnaires each morning (AM) and evening (PM). Strength and conditioning (S&C) training was performed two mornings each week, with hamstring and abductor/adductor performance tests as part of the S&C training on match day (MD) -4 (days). Twice a week, venous blood was drawn under resting conditions, prior to training, in a fasted state (MD -4, and -2), followed by CMJ testing. Players had previous experience with the procedures used in the study (i.e., hamstring and abductor/adductor, CMJ performance tests, questionnaires, but not venous blood sampling) prior to the start of the study. Team soccer training and matches were consistently monitored with a local positioning system (LPS).
MeasuresPerformance, injury, and illnessPerformance data (e.g., distance covered, heart rate, high metabolic power distance (HMPD), training impulse (TRIMP) (Stagno et al., 2007), total number of sprints, accelerations, decelerations) were recorded using a 100 Hz LPS (Kinexon Precision Technologies, Munich, Germany) during matches and training. Injury statistics, in form of both time-loss and medical attention, were collected daily by the team's medical staff and physiotherapists in accordance with established guidelines (Fuller et al., 2006). Only non-contact injuries were included in the current analysis. Similarly, illness statistics were collected daily by staff members. Additional measures due to COVID-19 included a daily questionnaire, covering of illness, such as cough, pain in the limbs, breathing difficulties, and loss of taste or sense of smell, along with daily body temperature measurements.
Physiological exercise testing prior to season startPlayers performed physiological exercise testing prior to the season to determine maximal oxygen uptake (VO2max), peak running speed (Vpeak) and lactate threshold using a 2-phase (submaximal step-wise and maximal ramp) test as previously described (Stöggl et al., 2022).
QuestionnairesUsing cluster analysis of our pilot study data (Haller et al., 2022), psychologists designed a questionnaire: specifically, from 23 (AM) and 8 (PM) questions conducted prior to the present study, the number of items (redundancy addressing the same psychological domain) was refined to 5 (AM) and 5 (PM) questions for the present study. Questionnaires were administered via a smartphone app (Trayn, Sunnyvale, CA, United States) and completed by players daily, in both the morning and evening. The AM questionnaire items were related to sleep, muscular fatigue, and energy level. The PM questions were related to perceived fatigue from training/game, stress, satisfaction, and mental strength. Questionnaires were completed on a Likert scale from 0-10, except for sleep and wake times, where the time was to be reported. The complete questionnaire can be found in Appendix 1.
Nordic hamstring strengthEccentric hamstring strength was measured with the Nordic hamstring exercise on the Nordbord device (Vald Performance, Albion, Australia) (Opar et al., 2013) during S&C training on MD -4 (Figure 1). Players knelt on the device with the fixation hooks oriented vertically and positioned just above the ankles (standardized position). Three repetitions were performed at maximal effort, with 5 s of rest in between trials. The experimenter instructed the players to keep their bodies straight and resist falling for as long as possible. Verbal encouragement in the form of "hold, hold, hold" was given. The mean value of the maximum force (Fmax) of the left and right leg of each trial was included in the statistical analysis.
Hip abduction, adduction strengthIsometric force of hip abduction and hip adduction was measured using the ForceFrame device (Vald Performance, Albion, Australia) on MD -4. After a general warm-up of 12-15 min, players were barefoot in the supine position, with their arms crossed in front of the chest. The hips were positioned in 0° flexion and neutral rotation. The medial malleoli were centered over the inner load cells for adduction, and the lateral malleoli over the outer load cells to test abduction. A single repetition at 100% was performed in both abduction and adduction. As shown in our pilot study the maximum values for both abduction and adduction occur in the vast majority of cases in the first repetition. Each repetition was held for 5 s, with a 10 s break between repetitions. Verbal encouragement in the form of, “3, 2, 1 push, push, push“ was given (Haller et al., 2022).
Neuromuscular performanceThe CMJ as a proxy of neuromuscular performance was performed on a split force plate (Forcedecks, VALD Performance, Albion, Australia), with arms fixed at the hip. To save time, the jumps were integrated into the 15-min team warm-up treadmill running session in which the players rotated to perform the jumps and then continued treadmill running. The order of the players to perform the jumps remained the same throughout the study period. Following two warm-up jumps (while waiting for the jumps on the force plate), two maximal jump attempts were performed in a standardized order (Gathercole et al., 2015; Twist and Highton, 2013; Watkins et al., 2017). Participants were instructed to jump as high as possible in each trial, with the depth of the CMJ chosen by players themselves (Haller et al., 2022).
Blood collectionVenous blood (~ 3-5 ml) was collected at rest, in a fasted condition, on days MD -4 and -2 by certified medical staff, and analyzed for, i) cell-free DNA (cfDNA) levels, ii) hematological blood count and iii) further established blood parameters. For cfDNA analyses, blood was immediately centrifuged after collection at 1600 x g for 10 min. The plasma was then stored at < -20° C. Briefly, plasma was diluted 1:10 in H2O and served as a template for qPCR based on amplification of a 90-base pair sequence within the L1PA2 transposon. A CFX384 Touch™ real-time PCR system (Bio-Rad, Munich, Germany) was used to analyze the blood samples according to the following protocol: Denaturation at 98° C for 2 min, 35 cycles of melting at 95° C for 10 s, annealing at 64° C for 10 s, followed by a melting curve (Neuberger et al., 2021). Differential blood count and further biochemical variables were determined using whole blood by the Mythic 22 Haematology Analyzer (Orphée, Geneva, Switzerland). An overview of all blood-based biomarkers is presented in Appendix 2. .
Statistical analysisFeasibility was determined by the number of adverse events and discontinuations during the study period. Adherence, which was calculated using the following formula: the number of completed tests or questionnaires performed divided by the total number of scheduled tests or questionnaires (i.e., (completed/scheduled) x 100 to express as percentage). In addition, we targeted three classification tasks: We evaluated the ability to predict a non-contact injury (yes/no), based on data from the most recent monitoring session. Second, we evaluated the ability to predict illness (yes/no) based on the most recent blood data. Third, we evaluated the association between illness (yes/no) with blood data from the same day, to determine whether current illness can be identified via the blood variables. For all three classification tasks, we excluded two participants due to missing data (one player was injured during the entire study period; for another player, only 2 weeks of data were available due to injury and illness), resulting in a total number of 23 participants. For the analysis, 18 participants were randomly selected as the training data set and 5 participants were selected as the test data set. The allocation of training and test data sets remained the same for all three tasks, to facilitate comparison of the results between tasks. The training data set for injury prediction included seven data points (indicating the presence or absence of an injury/illness) with and 1078 without an injury, while the test data set has four data points with and 296 without an injury. The training data set for illness prediction consists of 11 data points with and 272 without an illness, while the test data set has one data point with and 59 data points without an illness. For illness determination, the training data set contained 9 data points with and 168 without an illness, while the test data set has one data point with and 41 data points without an illness, which leads to a highly imbalanced data set (Table 1).
OversamplingIn imbalanced data sets standard classification methods tend to ignore the minority class and may be dominated by the majority class (Guo et al., 2008). Basically, there are two main methods discussed in the literature to solve the problem of imbalanced data, i.e., Undersampling and Oversampling. Undersampling methods randomly eliminate observations from the majority class, resulting in a loss of data (Kotsiantis et al., 2006). Oversampling, on the other hand, randomly replicates or generates observations from the minority class, which can lead to overfitting (Weiss and Provost, 2001). We chose oversampling techniques that circumvent the disadvantage of possible overfitting by generating synthetic copies of minority-class observations rather than exact copies. In view of the mixed variable types (categorical and numerical variables) in injury prediction, we applied the Synthetic Minority Over-sampling Technique-Nominal Continuous (SMOTE-NC) (Chawla et al., 2002) algorithm to create a balanced training data set. The number of nearest neighbors (smallest Euclidean distance between feature vectors) used to create the new sample was set to five. The percentage that the minority class (e.g., fewer injuries than non-injuries; therefore, in this case, the injury is the minority class) should have in the new data set compared to the majority class was set at 25%. The training data set thus consists of 1078 data points without injury and 269 with injury. We have opted for the Adaptive Synthetic Sampling Approach for Imbalanced Learning (ADASYN) algorithm (He et al., 2008) in view of the numerical data for illness prediction and determination. During the sampling process, we set the number of nearest neighbors to two, to generate the training data. As a result, we included 271 data points with illness and 272 data points without illness in our training data set for illness prediction. For illness determination, we included 169 data points with illness and 168 data points without illness in our training data set.
ClassificationFor the classification purpose, we applied several machine-learning algorithms such as tree-based methods, naive bayes, or neural networks. Ultimately, a simple linear SVM (Hearst et al., 1998) demonstrated the best results (in terms of accuracy and Cohens Kappa) for all three classification tasks. SVM uses a simple mathematical model and manipulates it in such a way that a linear division of the domain is possible. Basically, SVMs can be distinguished between linear and nonlinear models (Hastie et al., 2009; Suthaharan, 2016). In our case, a linear SVM is used, which divides the data domain linearly into individual classes. To assess the importance of variables for each classification task, we employed the caret package (Kuhn, 2022) with a Receiver Operating Characteristic (ROC) curve analysis to analyze variable importance for SVMs to identify the most important variables. To calculate the variable importance, the sensitivity and specificity values are calculated for different cut-offs of the predictor data. From this, the ROC is calculated using the trapezoidal rule, where the area under the ROC is used to interpret the variable importance (Kuhn, 2022). Table 1 displays an overview of the classification task, model, types, and number of variables, as well as sizes of the training and test data sets. To train the algorithm, we used a two-fold cross-validation and tried 10 different values per algorithm parameter and chose the parameters that showed the highest area under the ROC curve. The best model was finally used to evaluate the test data. To create the balanced data set, we used the RSBID package (Wu, 2022) to apply the SMOTE-NC algorithm and the smotefamily package (Siriseriwan, 2019) for the ADASYN algorithm from the statistical software R (R Foundation for Statistical Computing, Vienna, Austria). The models were trained with the caret package (Kuhn, 2022), which also was used to calculate variable importance.
Performance metricsAs metrics to evaluate the classification tasks we chose accuracy, Cohen’s Kappa, precision, and recall. Accuracy is the ratio between the sum of true-positive and true-negative predictions, divided by the sum of positive and negative observations. Precision represents the ratio between the sum of true-positives divided by the sum of predicted-positives, while recall shows the sum of true-positives divided by the sum of positive observations. Cohen’s Kappa (Cohen, 1960) allows comparison between model results and random results. The value is the ratio between the accuracy of the prediction minus the random accuracy divided by one minus random accuracy and can be interpreted as the proportion of the accuracy where random accuracy is excluded. This metric can range from -1 to +1. A perfect classification would give a value of +1, 0 for a result equal to a random classification and -1 for a result worse than a random classification (Cohen, 1960). As the primary task of our models is to detect injury or illness, we defined the occurrence of an injury or an illness as the positive class.
Data pre-processingFor the evaluation of the injury prediction task, we used a total of 65 training load variables (all 65 variables are outlined in Appendix 3). To better detect anomalies of the respective participants, we scaled the load variables per participant. We also took the EWMA of the last two training sessions of each participant where the player was not injured. In addition, we calculated the ACWR, i.e., the ratio between the mean value of the respective load variables of the last seven days (x7) and the mean value between the eighth and the 28th day (x8-28) of a respective date. For the evaluation, we also took the values of x7 and x8-28 into account. Due to missing data, it was not possible to calculate EWMA, x7, x8-28 and ACWR (Gabbett, 2018; Murray et al., 2017) for all load variables. Thus, in total 237 load variables could be used for the injury prediction task. In addition, we used two performance parameters (Vpeak, VO2max), three items of the questionnaires (sleep quality, sleep duration, and muscle fatigue), two blood variables (CK and cfDNA), two jump variables (jump height impulse max, concentric peak force max) and a dummy whether the participant had a physiotherapist treatment on the respective day. Since these data were collected less frequently than the training load variables covered by LPS, we could not simply merge these data sets. Therefore, we used two different approaches to solve this problem by including these variables via clustering approaches inspired by Rossi et al. (2023). For blood, questionnaire and jump data, we applied a longitudinal clustering algorithm that divided the participants into specific groups. We included these groups in our model via factor variables. These factor variables were calculated with the kml algorithm (Genolini et al., 2015), a k-means algorithm for longitudinal data, where clusters were determined for each variable separately. For the calculation, all values were aggregated using the mean values per week and missing values were imputed using the "CopyMean" function of the kml package. Due to the small number of participants, we choose to set the number of cluster groups to two. However, there was an outlier in the data for the two blood variables and sleep duration, so we decided to have three cluster groups for these variables. As information on performance parameters was only available for two time points (before and after the study period), a simple k-means algorithm of the R package factoextra (Kassambara and Mundt, 2020) was used to calculate two cluster groups, for VO2max and Vpeak respectively, by taking the mean of pre and post.
Aspects of feasibilityNo adverse events in the form of injury or dropout were noted during the study period, although some players had concerns about repeated blood sampling. Figure 2 shows the sleep quality, as one example of the questionnaire data, for each player as a mean value per week over the study period. The figure illustrates declining adherence to the questionnaire across the study period. It appears that most players accepted the questionnaire until about halfway through the study duration, but thereafter acceptance decreased. We noted a decline in adherence from 95.7% in the first week to 73.9% in the seventh week to 21.7% in the fourteenth week.
Results of prediction tasksTable 2 displays the results for all three classification tasks for the test data set: injury prediction, illness prediction, and illness determination. The linear SVM was able to predict 96.3% of data points (injury yes/no) correctly. The recall score of 25% indicates that one of the four injuries in the test set was detected by the model. A precision of 11.1% shows that one out of nine predicted injuries was actually an injury. In the case of illness prediction, the linear SVM predicted 66.7% of the data points correctly. A recall value of 100% shows that the one illness present in the test set was detected. A precision of 4.8% indicates that out of 21 predicted illnesses, one was actually an illness. The model to determine illness detects all data points in the test set correctly, which resulted in an accuracy, precision, and recall value of 100% each and a Cohens Kappa of 1. To investigate the most important variables for classification of each task Figure 3 visualizes the ten most important variables according to ROC curve variable importance. Regarding variable importance for injury prediction, four out of the ten most important variables represent factor variables out of the longitudinal cluster approach (sleep quality, jump height, cfDNA, and sleep duration). Sleep quality was detected to be most important, followed by the ACWR of tempo runs (> 19.8 km/h) and CMJ jump height. For illness prediction and determination, five blood variables were in the top ten for both classification tasks (total ferritin, total c-reactive Protein (CRP), percentage and total eosinophils (EOS) and glucose (GLU)), with total CRP and percentage EOS being two variables in the top three most important variables. In general, illness determination task demonstrated the highest ROC curve variable importance values, followed by the injury and illness prediction variables, which would have been expected according to the results of the model evaluation.
The overall goals of this study were to i) demonstrate the feasibility of a comprehensive monitoring approach being fully integrated in the training process, and ii) test the predictive accuracy of a machine learning approach in terms of injury and illness prediction using the combination of external training load data and a variety of objective (neuromuscular performance and strength testing, biomarkers, heart rate) and subjective (questionnaire) internal load and recovery measures in an elite youth soccer team. It has been demonstrated that it is possible to develop machine learning models to predict injuries and to detect and predict illnesses.
Principal findingsIn general, the integration of a holistic monitoring approach into the training regime can only succeed if the following factors are present: 1) coach buy-in, 2) cost, time, and logistical prerequisites, 3) team adherence, 4) an interdisciplinary team, and 5) the benefits coaches see in an empirically based measure (Akenhead and Nassis, 2016). Therefore, it is important to integrate the majority of measures into the regular training routine with the help of an interdisciplinary team of practitioners, researchers, and clinicians to avoid disrupting the training process and causing additional burden to the players. It has been shown that a period of 15 minutes in the morning is sufficient for blood sampling. An additional 15 minutes before regular training was sufficient for measuring CMJ performance, which was integrated into the team warm-up program on the treadmill. Questionnaires as an easy-to-use and established tool were completed within 1-2 minutes in the morning and evening. Measurements of hip adductor, hip abductor, and hamstring strength were categorized as a strength training stimulus and therefore incorporated into S&C training on MD -4. The performance tests did not result in any adverse events. However, some of the players showed concerns on repetitive venous blood sampling. Accordingly, future research should focus on identifying key blood variables for monitoring training load and recovery which can be measured via capillary blood or even saliva, ideally via point-of-care testing, similar to the measurement of creatine kinase. Testing various machine learning models showed that the linear SVM resulted in the best classification results for the three different classification tasks of interest. To develop the best performing model, many variables were taken into account. However, in order to simplify data collection in the future the most relevant parameters were identified by a ROC curve variable importance analysis. For injury prediction, the three most important variables were sleep quality, the ACWR of tempo runs (> 19.8 km/h) and CMJ jump height. For illness prediction, ferritin, CRP and percentage EOS were identified to be most important, for illness determination CRP, creatinine and percentage EOS. For injury prediction, one of four injuries present in the test data set was detected and 96.3% of all data points were detected correctly. For illness prediction and determination, only one illness was present in the test data set, as the same random split of players into training or test data set for all three classification tasks was used. However, this data point was detected by the linear SVM for both illness prediction and determination. Unfortunately, the model showed quite low precision values for both predictive tasks. Thus, the model tends to predict false-positive injuries and illnesses. Differences in accuracy between illness determination and prediction appear reasonable because in some cases there was some time lag between blood collection and illness onset. Thus, the accuracy is not expected to be perfect for the prediction task. In addition, it should be noted that throughout the study period, COVID illnesses occurred as well as illnesses that may have been specifically related to the high density of training and competition. In practical terms, applying the present model could lead to over-estimation of players’ risk of injuries and illnesses. On the other hand, the model works perfectly to detect illness, driven mainly by the CRP variable, which was shown to have the highest importance among blood variables. According to a categorization framework presented by Landis and Koch (Landis and Koch, 1977), Cohens' Kappa values show slight performance for predicting injury and illness, but perfect performance for detecting illness. During the study period of three months, there were too few injuries and illnesses from a statistical perspective to develop a better predictive model. Comparable studies covered longer periods of at least about half a year or more and included more injuries in their predictive models (Rommers et al., 2020; Rossi et al., 2018). In addition, a higher frequency of data points would have been needed for some variables. For blood collection, two samplings per week were the maximum from an adherence perspective. Switching to capillary samples could help, with the disadvantage that fewer variables can be determined. The main challenge in classifying injuries and illnesses is the limited injury and illness data set, which contrasts with the almost daily data available on players' training load. We have therefore tried to oversample the number of illnesses and injuries in the training data set. Nevertheless, the number of illnesses and injuries in the test data set remains limited. The different time points for data collection also led to challenges in merging the different data sets. Since many variables (blood, jumps) were collected less frequently and over a shorter period compared to the training load data, this information was included in the machine learning models via clusters leading to factor variables. Therefore, the information on blood, jumps and fitness was aggregated and not included as detailed as would be possible with more frequent measures. When using longitudinal clusters to include the blood, questionnaire and jump data in the injury prediction analysis, we were faced with a decreasing willingness to fill out the questionnaires. Therefore, the number of missing values increased over time, and we could not use these data over the entire survey period. While there was an increase in questionnaire adherence compared to our pilot study (Haller et al., 2022), further strategies to increase adherence are necessary to minimize missing data. This includes, for example, regular educational talks (McGuigan et al., 2023), reminders by the coaching staff, and a clear message that the players will benefit enormously from filling out questionnaires on a frequent basis. It is expected that adherence increases if the monitoring results are coupled with consequences in training and recovery planning.
Practical applicationsThe strength of machine learning approaches is to not only seek linear relationships and consider only one or two parameters, but to consider specifically the interaction between many variables, which may be necessary due to the multifactorial nature of injury and illness. In the present study, training load data, questionnaire scores and blood variables were found to be potentially associated with impending injury or illness. Unfortunately, it is not possible to draw conclusions about the specific direction of the variables, which might be possible with other statistical methods. While tracking variables have been associated with impending injury, blood variables could be useful for early detection of illness. Specifically, illness determination showed the best model performance, but the precision was low for prediction tasks. From a practical point of view, this may lead to overcautious reactions from practitioners so far. Hence, it is necessary to test whether the identified important variables persist by feeding the algorithm with additional data points and to observe the evolution of the accuracy measures. The long-term goal is to capture the critical variables with minimal effort (i.e., omitting unnecessary methods to minimize human effort and avoid large amounts of data) or minimally invasive, e.g., for blood with point-of-care devices in the future. The use of minimally invasive point-of-care methods would also allow for more frequent blood collection than two days per week, and it is not recommended to collect venous blood at this regularity anyway, as this would impose an immense burden on the athletes in the long term (Carling et al., 2018). Strategies to further reduce the large amount of redundant data (e.g., tracking, blood and CMJ variables) are recommended and discussed elsewhere. Lastly, it should be noted that the study required a highly professional environment and significant human resources (two physicians for blood collection, two physiotherapists for hip abduction/adduction, two practitioners for the CMJ). The blood collection required staff and sophisticated equipment for the analysis including sophisticated qPCR methods for the determination of cfDNA. Thus, the approach is feasible for financially strong clubs, but not practical and cost-effective for non-elite clubs or professional clubs with limited resources, unless the machine models perform better.
A holistic approach to monitoring training load and training load response was successfully integrated into regular practice, and many variables indicative of the occurrence of injury and illness were identified. Whereas conventional statistical approaches have the disadvantage of focusing, for example, on factors linearly associated with injury and disease by performing regression analyses, we provide an approach to consider interactions among a large number of variables potentially associated with illness and injury. Future studies can build on our initial results and apply a longer study period with more data points to further train the algorithms and determine if the variables identified are truly critical. Further statistical methods can then be used i) to reveal and interpret the direction of the variables, or ii) the crucial variables can be used in practice over a longer period of time so that changes can be identified on an individual basis allowing early interventions to be made.
ACKNOWLEDGEMENTS |
We would like to thank all the players, practitioners, and club officials who agreed to, planned, participated in, helped with, or conducted the study. The study is a cooperation project between the University of Salzburg, the University of Mainz and the Red Bull Athlete Performance Center. The study receives funding from the Red Bull Athlete Performance Center for the scientific accompaniment of the monitoring concept in the context of which data for the current study were collected. Christina and Stefan Kranzinger acknowledge the financial support by the Austrian Federal Ministry for Climate Action, Environment, Energy, Mobility, Innovation and Technology and Land Salzburg under Contract No. 2021-0.641.557. All experiments comply with the current laws of the country in which they were performed. The authors declare that the research was conducted in the absence of any commercial or financial relationships that could be construed as a potential conflict of interest. The data sets generated and analyzed during the current study are not publicly available but are available from the corresponding author, who was an organizer of the study. |
|
AUTHOR BIOGRAPHY |
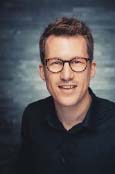 |
Nils Haller |
Employment: Senior Researcher, Department of Sport and Exercise Science, University of Salzburg; Department of Sports Medicine, Rehabilitation and Disease Prevention, University of Mainz, Mainz, Germany |
Degree: PhD |
Research interests: Exercise physiology, Load monitoring, Sports therapy |
E-mail: nhaller@uni-mainz.de |
|
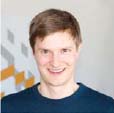 |
Stefan Kranzinger |
Employment: Researcher, Salzburg Research Forschungsgesellschaft m.b.H |
Degree: Mag., MSc., PhD |
Research interests: Human motion analysis, Machine learning, Statistics |
E-mail: stefan.kranzinger@salzburgresearch.at |
|
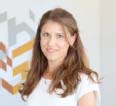 |
Christina Kranzinger |
Employment: Researcher, Salzburg Research Forschungsgesellschaft m.b.H |
Degree: Mag., MSc. |
Research interests: Human motion analysis, Machine learning, Statistics |
E-mail: christina.kranzinger@salzburgresearch.at |
|
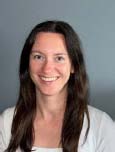 |
Julia C. Blumkaitis |
Employment: Research Assistant, Department of Sport and Exercise Science, University of Salzburg |
Degree: M.Sc. |
Research interests: Exercise physiology, Training load monitoring |
E-mail: julia.blumkaitis@plus.ac.at |
|
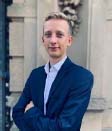 |
Tilmann Strepp |
Employment: PhD Student, Department of Sport and Exercise Science, University of Salzburg |
Degree: M.Sc. |
Research interests: Sport science, Exercise physiology |
E-mail: tilmann.strepp@plus.ac.at |
|
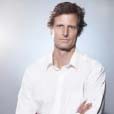 |
Perikles Simon |
Employment: Department of Sports Medicine, Rehabilitation and Disease Prevention, University of Mainz, Mainz, Germany |
Degree: MD, PhD |
Research interests: Exercise immunology, Molecular exercise physiology, Sports medicine |
E-mail: simonpe@uni-mainz.de |
|
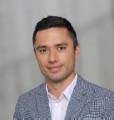 |
Aleksandar Tomaskovic |
Employment: Department of Sports Medicine, Rehabili-tation and Disease Prevention, University of Mainz, Mainz, Germany |
Degree: M.Sc. |
Research interests: Training load management, Sports physiotherapy |
E-mail: altomask@uni-mainz.de |
|
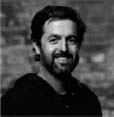 |
James O’Brien |
Employment: Red Bull Athlete Performance Center, Salzburg, Austria |
Degree: MSc, PhD |
Research interests: Injury prevention, Sports physiotherapy |
E-mail: james.obrien@redbullperformance.com |
|
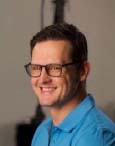 |
Manfred Düring |
Employment: Head of Performance at FC Red Bull Salzburg |
Degree: Dipl., Dr. Sports Science |
Research interests: Monitoring & training load management, Exercise physiology, Biomechanics, Performance diagnostics |
E-mail: manfred.duering@redbullsalzburg.at |
|
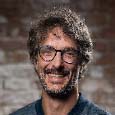 |
Thomas Stöggl |
Employment: Head of R&D and Science at the Red Bull Athlete Performance Center |
Degree: Univ-. Prof. Mag. Dr. |
Research interests: Physiology and biomechanics in sports from sedentary to the elite athlete; training intensity distribution among elite endurance athletes; Exercise physiology and performance diagnostics; Sensor technologies in various settings of sport science |
E-mail: thomas.stoeggl@redbullperformance.com |
|
|
|
REFERENCES |
 Akenhead R., Nassis G. P. (2016) Training Load and Player Monitoring in High-Level Football: Current Practice and Perceptions. International Journal of Sports Physiology and Performance 11, 587-593. Crossref
|
 Ayala F., Lopez-Valenciano A., Martin J. A. G., Croix M. D., Vera-Garcia F. J., Garcia-Vaquero M. D., Ruiz-Perez I., Myer G. D. (2019) A Preventive Model for Hamstring Injuries in Professional Soccer: Learning Algorithms. International Journal of Sports Medicine 40, 344-353. Crossref
|
 Carling C., Lacome M., McCall A., Dupont G., Le Gall F., Simpson B., Buchheit M. (2018) Monitoring of Post-match Fatigue in Professional Soccer: Welcome to the Real World. Sports Medicine. Crossref
|
 Chawla N. V., Bowyer K. W., Hall L. O., Kegelmeyer W. P. (2002) SMOTE: Synthetic minority over-sampling technique. Journal of Artificial Intelligence Research 16, 321-357. Crossref
|
 Claudino J. G., Cronin J., Mezencio B., McMaster D. T., McGuigan M., Tricoli V., Amadio A. C., Serrao J. C. (2017) The countermovement jump to monitor neuromuscular status: A meta-analysis. Journal of Science and Medicine in Sport 20, 397-402. Crossref
|
 Cohen J. (1960) A Coefficient of Agreement for Nominal Scales. Educational and Psychological Measurement 20, 37-46. Crossref
|
 Fuller C. W., Ekstrand J., Junge A., Andersen T. E., Bahr R., Dvorak J., Hagglund M., McCrory P., Meeuwisse W. H. (2006) Consensus statement on injury definitions and data collection procedures in studies of football (soccer) injuries. Clinical Journal of Sport Medicine 16, 97-106. Crossref
|
 Gabbett T. (2018) Infographic: The training-injury prevention paradox: should athletes be training smarter and harder? [Editorial]. British Journal of Sports Medicine 52, 203. Crossref
|
 Gathercole R., Sporer B., Stellingwerff T., Sleivert G. (2015) Alternative countermovement-jump analysis to quantify acute neuromuscular fatigue. International Journal of Sports Physiology and Performance 10, 84-92. Crossref
|
 Genolini C., Alacoque X., Sentenac M., Arnaud C. (2015) kml and kml3d: R packages to cluster longitudinal data. Journal of Statistical Software 65, 1-34. Crossref
|
 Guo X., Yin Y., Dong C., Yang G., Zhou G. (2008) On the class imbalance problem. Fourth international conference on natural computation. Crossref
|
 Haller N., Behringer M., Reichel T., Wahl P., Simon P., Kruger K., Zimmer P., Stöggl T. (2023a) Blood-Based Biomarkers for Managing Workload in Athletes: Considerations and Recommendations for Evidence-Based Use of Established Biomarkers. Sports Medicine 53, 1315-1333. Crossref
|
 Haller N., Blumkaitis J. C., Strepp T., Schmuttermair A., Aglas L., Simon P., Neuberger E., Kranzinger C., Kranzinger S., O'Brien J., Ergoth B., Raffetseder S., Fail C., During M., Stöggl T. (2022) Comprehensive training load monitoring with biomarkers, performance testing, local positioning data, and questionnaires-first results from elite youth soccer. Frontiers in Physiology 13. Crossref
|
 Haller N., Reichel T., Zimmer P., Behringer M., Wahl P., Stoggl T., Kruger K., Simon P. (2023b) Blood-Based Biomarkers for Managing Workload in Athletes: Perspectives for Research on Emerging Biomarkers. Sports Medicine. Crossref
|
 Hastie T., Tibshirani R., Friedman J. H., Friedman J. H. (2009) . The elements of statistical learning: data mining, inference, and prediction (Vol. 2) Springer. Crossref
|
 He H., Bai Y., Garcia E. A., Li S. (2008) . ADASYN: Adaptive synthetic sampling approach for imbalanced learning. 2008 IEEE international joint conference on neural networks (IEEE world congress on computational intelligence).
|
 Hearst M. A., Dumais S. T., Osuna E., Platt J., Scholkopf B. (1998) Support vector machines. IEEE Intelligent Systems and their applications 13, 18-28. Crossref
|
 Kassambara, A. and Mundt, F. (2020) Package 'factoextra': extract and visualize the results of multivariate data analyses. CRAN-R
Package. https://CRAN.R-project.org/package=factoextra
|
 Kotsiantis S., Kanellopoulos D., Pintelas P. (2006) Handling imbalanced datasets: A review. GESTS International Transactions on Computer Science and Engineering 30, 25-36.
|
 Kuhn, M. (2022) Package 'caret': Classification and Regression Training, Version 6.0-94.
https://cran.r-project.org/web/packages/caret/caret.pdf
|
 Landis J. R., Koch G. G. (1977) The measurement of observer agreement for categorical data. Biometrics 33, 159-174. Crossref
|
 Malone S., Owen A., Mendes B., Hughes B., Collins K., Gabbett T. J. (2018) High-speed running and sprinting as an injury risk factor in soccer: Can well-developed physical qualities reduce the risk?. Journal of Science and Medicine in Sport 21, 257-262. Crossref
|
 McGuigan H. E., Hassmen P., Rosic N., Thornton H. R., Stevens C. J. (2023) Does education improve adherence to a training monitoring program in recreational athletes?. International Journal of Sports Science & Coaching 18, 101-113. Crossref
|
 Murray N. B., Gabbett T. J., Townshend A. D., Blanch P. (2017) Calculating acute: chronic workload ratios using exponentially weighted moving averages provides a more sensitive indicator of injury likelihood than rolling averages. British Journal of Sports Medicine 51, 749-754. Crossref
|
 Nassis G. P., Verhagen E., Brito J., Figueiredo P., Krustrup P. (2023) A review of machine learning applications in soccer with an emphasis on injury risk. Biology of Sport 40, 233-239. Crossref
|
 Neuberger E. W. I., Brahmer A., Ehlert T., Kluge K., Philippi K. F. A., Boedecker S. C., Weinmann-Menke J., Simon P. (2021) Validating quantitative PCR assays for cfDNA detection without DNA extraction in exercising SLE patients. Scientific Reports 11. Crossref
|
 Opar D. A., Piatkowski T., Williams M. D., Shield A. J. (2013) A Novel Device Using the Nordic Hamstring Exercise to Assess Eccentric Knee Flexor Strength: A Reliability and Retrospective Injury Study. Journal of Orthopaedic & Sports Physical Therapy 43, 636-640. Crossref
|
 Rodas G., Osaba L., Arteta D., Pruna R., Fernandez D., Lucia A. (2020) Genomic Prediction of Tendinopathy Risk in Elite Team Sports. International Journal of Sports Physiology and Performance 15, 489-495. Crossref
|
 Rommers N., Rossler R., Verhagen E., Vandecasteele F., Verstockt S., Vaeyens R., Lenoir M., D'Hondt E., Witvrouw E. (2020) A Machine Learning Approach to Assess Injury Risk in Elite Youth Football Players. Medicine & Science in Sports & Exercise 52, 1745-1751. Crossref
|
 Rossi A., Pappalardo L., Cintia P., Iaia F. M., Fernandez J., Medina D. (2018) Effective injury forecasting in soccer with GPS training data and machine learning. Plos One 13. Crossref
|
 Rossi A., Pappalardo L., Filetti C., Cintia P. (2023) Blood sample profile helps to injury forecasting in elite soccer players. Sport Sciences for Health 19, 285-296. Crossref
|
 Ruddy J. D., Shield A. J., Maniar N., Williams M. D., Duhig S., Timmins R. G., Hickey J., Bourne M. N., Opar D. A. (2018) Predictive Modeling of Hamstring Strain Injuries in Elite Australian Footballers. Medicine & Science in Sports & Exercise 50, 906-914. Crossref
|
 Saw A. E., Main L. C., Gastin P. B. (2016) Monitoring the athlete training response: subjective self-reported measures trump commonly used objective measures: a systematic review [Research Support, Non-U.S. Gov't Review]. British Journal of Sports Medicine 50, 281-291. Crossref
|
 Schwellnus M., Soligard T., Alonso J. M., Bahr R., Clarsen B., Dijkstra H. P., Gabbett T. J., Gleeson M., Hagglund M., Hutchinson M. R., Janse Van Rensburg C., Meeusen R., Orchard J. W., Pluim B. M., Raftery M., Budgett R., Engebretsen L. (2016) How much is too much? (Part 2) International Olympic Committee consensus statement on load in sport and risk of illness [Consensus Development Conference]. British Journal of Sports Medicine 50, 1043-1052. Crossref
|
 Siriseriwan W. (2019) Smotefamily: A collection of oversampling techniques for class imbalance problem based on SMOTE. R Package Version 1.
|
 Soligard T., Schwellnus M., Alonso J. M., Bahr R., Clarsen B., Dijkstra H. P., Gabbett T., Gleeson M., Hagglund M., Hutchinson M. R., van Rensburg C. J., Khan K. M., Meeusen R., Orchard J. W., Pluim B. M., Raftery M., Budgett R., Engebretsen L. (2016) How much is too much? (Part 1) International Olympic Committee consensus statement on load in sport and risk of injury. British Journal of Sports Medicine 50, 1030-1041. Crossref
|
 Stagno K. M., Thatcher R., Van Someren K. A. (2007) A modified TRIMP to quantify the in-season training load of team sport players. Journal of Sports Sciences 25, 629-634. Crossref
|
 Stöggl T. L., Blumkaitis J. C., Strepp T., Sareban M., Simon P., Neuberger E. W. I., Finkenzeller T., Nunes N., Aglas L., Haller N. (2022) The Salzburg 10/7 HIIT shock cycle study: the effects of a 7-day high-intensity interval training shock microcycle with or without additional low-intensity training on endurance performance, well-being, stress and recovery in endurance trained athletes-study protocol of a randomized controlled trial. Bmc Sports Science Medicine and Rehabilitation 14. Crossref
|
 Suthaharan S. (2016) Machine learning models and algorithms for big data classification. Integrated Serien in Information Systems 36, 1-12. Crossref
|
 Twist C., Highton J. (2013) Monitoring fatigue and recovery in rugby league players. International Journal of Sports Physiology and Performance 8, 467-474. Crossref
|
 Van Eetvelde H., Mendonca L. D., Ley C., Seil R., Tischer T. (2021) Machine learning methods in sport injury prediction and prevention: a systematic review. Journal of Experimental Orthopaedics 8. Crossref
|
 Watkins C. M., Barillas S. R., Wong M. A., Archer D. C., Dobbs I. J., Lockie R. G., Coburn J. W., Tran T. T., Brown L. E. (2017) Determination of Vertical Jump as a Measure of Neuromuscular Readiness and Fatigue. Journal of Strength and Conditioning Research 31, 3305-3310. Crossref
|
 Watson A., Brickson S., Brooks M. A., Dunn W. (2017) Preseason Aerobic Fitness Predicts In-Season Injury and Illness in Female Youth Athletes. Orthopaedic Journal of Sports Medicine 5, 2325967117726976. Crossref
|
 Weiss, G. M. and Provost, F. (2001) The effect of class distribution on classifier learning: an empirical study. Rutgers University.
|
 Wu D. (2022) R package version 0.0.2.0000. https://github.com/dongyuanwu/RSBID
|
|
|
|
|
|
|